


How Can I Use NumPy Array Operations on Shared Memory in Multiprocessing?
Using NumPy Array in Shared Memory for Multiprocessing
Introduction
Utilizing NumPy arrays in shared memory is essential for parallelizing computations using the multiprocessing module. However, accessing and manipulating shared memory arrays as NumPy arrays can be challenging. This article delves into a solution to this issue.
Problem Statement
Creating a shared NumPy array accessible from multiple processes requires employing the multiprocessing module. The challenge lies in enabling operations such as element-wise multiplication and array summations, which are inherently supported by NumPy but not directly through ctypes.
Solution
The key to resolving this issue is to convert the ctypes array representing the shared memory into a NumPy array. To achieve this, we utilize the frombuffer function from NumPy. The resulting NumPy array maintains its shared memory status, allowing for seamless access across processes.
Example
import multiprocessing as mp import numpy as np # Create a shared ctypes array shared_arr = mp.Array(ctypes.c_double, 10) # Convert the shared array to a NumPy array np_arr = np.frombuffer(shared_arr.get_obj()) # Perform operations on the NumPy array np_arr[0] = -np_arr[0] np_arr.sum()
This approach provides the functionality of both ctypes and NumPy, allowing you to access and manipulate the shared memory array with the flexibility of NumPy operations.
Synchronization
While the conversion to a NumPy array provides access to NumPy operations, it does not guarantee synchronized access. If multiple processes attempt to access shared memory simultaneously, it can lead to unexpected results. To prevent this, a locking mechanism should be implemented using shared_arr.get_lock().
Additional Notes
- Alternatively, one could use mp.sharedctypes.RawArray for creating shared arrays without synchronization.
- A NumPy array with a shared memory backing is not directly serializable using pickle or other standard serialization methods.
The above is the detailed content of How Can I Use NumPy Array Operations on Shared Memory in Multiprocessing?. For more information, please follow other related articles on the PHP Chinese website!

Hot AI Tools

Undresser.AI Undress
AI-powered app for creating realistic nude photos

AI Clothes Remover
Online AI tool for removing clothes from photos.

Undress AI Tool
Undress images for free

Clothoff.io
AI clothes remover

Video Face Swap
Swap faces in any video effortlessly with our completely free AI face swap tool!

Hot Article

Hot Tools

Notepad++7.3.1
Easy-to-use and free code editor

SublimeText3 Chinese version
Chinese version, very easy to use

Zend Studio 13.0.1
Powerful PHP integrated development environment

Dreamweaver CS6
Visual web development tools

SublimeText3 Mac version
God-level code editing software (SublimeText3)

Hot Topics
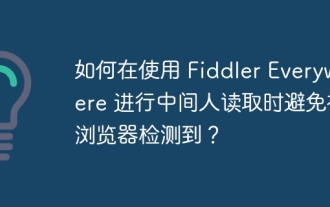
How to avoid being detected when using FiddlerEverywhere for man-in-the-middle readings When you use FiddlerEverywhere...
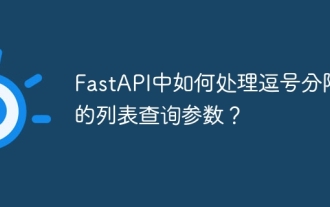
Fastapi ...
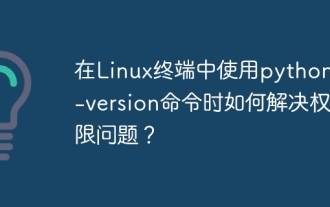
Using python in Linux terminal...
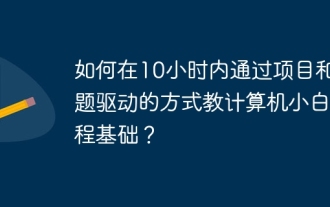
How to teach computer novice programming basics within 10 hours? If you only have 10 hours to teach computer novice some programming knowledge, what would you choose to teach...
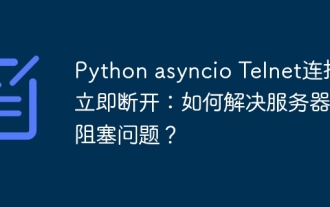
About Pythonasyncio...
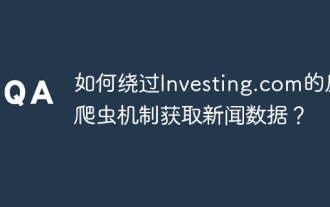
Understanding the anti-crawling strategy of Investing.com Many people often try to crawl news data from Investing.com (https://cn.investing.com/news/latest-news)...
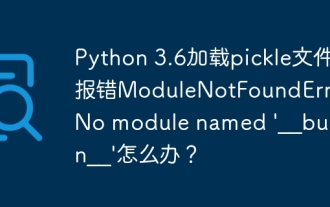
Loading pickle file in Python 3.6 environment error: ModuleNotFoundError:Nomodulenamed...
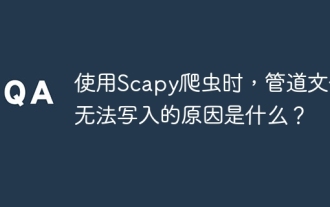
Discussion on the reasons why pipeline files cannot be written when using Scapy crawlers When learning and using Scapy crawlers for persistent data storage, you may encounter pipeline files...
