


Unlock Pythons Hidden Power: Master Abstract Syntax Trees for Code Wizardry
Python's metaprogramming capabilities are pretty awesome, and Abstract Syntax Trees (ASTs) take it to a whole new level. I've been playing around with ASTs lately, and I'm excited to share what I've learned.
At its core, an AST is a tree-like representation of the structure of your Python code. It's like looking at your code through a different lens, where each part of your program becomes a node in this tree. The cool thing is, you can manipulate this tree to change how your code behaves.
Let's start with a simple example. Say we have this piece of code:
x = 5 + 3 print(x)
When we parse this into an AST, it looks something like this:
import ast code = """ x = 5 + 3 print(x) """ tree = ast.parse(code) print(ast.dump(tree))
This will output a representation of the AST. It's a bit messy, but you can see how each part of our code is represented as a node in the tree.
Now, why is this useful? Well, it lets us do some pretty neat tricks. We can analyze code, modify it, or even generate new code on the fly. It's like having X-ray vision for your Python programs.
One of the coolest things you can do with ASTs is create custom language features. Imagine you're working on a big data project, and you're tired of writing the same boilerplate code for data validation. With ASTs, you could create a custom decorator that automatically adds validation code to your functions.
Here's a simple example:
import ast import inspect def validate_types(func): source = inspect.getsource(func) tree = ast.parse(source) for node in ast.walk(tree): if isinstance(node, ast.FunctionDef): for arg in node.args.args: if arg.annotation: check = ast.parse(f'if not isinstance({arg.arg}, {arg.annotation.id}): raise TypeError("Invalid type for {arg.arg}")').body[0] node.body.insert(0, check) new_func = compile(ast.fix_missing_locations(tree), '<string>', 'exec') namespace = {} exec(new_func, namespace) return namespace[func.__name__] @validate_types def greet(name: str, times: int): for _ in range(times): print(f"Hello, {name}!") greet("Alice", 3) # This works greet("Bob", "not a number") # This raises a TypeError
In this example, we've created a decorator that automatically adds type checking to our function. It parses the function into an AST, adds type checking code for each annotated argument, and then recompiles the function. Pretty cool, right?
But we're just scratching the surface here. ASTs can be used for all sorts of things. Code optimization is another big one. You could write an AST transformer that looks for certain patterns in your code and replaces them with more efficient versions.
For instance, let's say you're working with a lot of string concatenation in your code. You know that using join() is often faster than the operator for strings, especially when dealing with many strings. You could write an AST transformer that automatically converts string concatenation to join() calls:
import ast class StringConcatOptimizer(ast.NodeTransformer): def visit_BinOp(self, node): if isinstance(node.op, ast.Add) and isinstance(node.left, ast.Str) and isinstance(node.right, ast.Str): return ast.Call( func=ast.Attribute( value=ast.Str(s=''), attr='join', ctx=ast.Load() ), args=[ ast.List( elts=[node.left, node.right], ctx=ast.Load() ) ], keywords=[] ) return node # Usage code = """ result = "Hello, " + "world!" """ tree = ast.parse(code) optimizer = StringConcatOptimizer() optimized_tree = optimizer.visit(tree) print(ast.unparse(optimized_tree)) # Output: result = ''.join(['Hello, ', 'world!'])
This transformer looks for string concatenation operations and replaces them with join() calls. It's a simple example, but you can imagine how powerful this could be for larger codebases.
ASTs are also great for static analysis. You can write tools that scan your code for potential bugs, style violations, or security vulnerabilities. Many popular linting tools use ASTs under the hood to analyze your code.
Here's a simple example of how you might use an AST to find all the function definitions in a piece of code:
x = 5 + 3 print(x)
This function parses the code into an AST, then walks through the tree looking for FunctionDef nodes. It's a simple example, but you can see how this could be extended to do more complex analysis.
One area where ASTs really shine is in creating domain-specific languages (DSLs). These are languages tailored for a specific task or domain. With ASTs, you can parse these custom languages and translate them into Python code.
For example, let's say you're working on a data analysis project, and you want to create a simple language for defining data transformations. You could use ASTs to parse this language and generate Python code:
import ast code = """ x = 5 + 3 print(x) """ tree = ast.parse(code) print(ast.dump(tree))
This parser takes a simple DSL for data transformation and converts it into Python code using pandas. It's a basic example, but it shows how you can use ASTs to create your own mini-languages tailored to your specific needs.
ASTs are also incredibly useful for code refactoring. You can write tools that automatically update your code to follow new patterns or conventions. For instance, let's say you want to update all your print statements to use f-strings:
import ast import inspect def validate_types(func): source = inspect.getsource(func) tree = ast.parse(source) for node in ast.walk(tree): if isinstance(node, ast.FunctionDef): for arg in node.args.args: if arg.annotation: check = ast.parse(f'if not isinstance({arg.arg}, {arg.annotation.id}): raise TypeError("Invalid type for {arg.arg}")').body[0] node.body.insert(0, check) new_func = compile(ast.fix_missing_locations(tree), '<string>', 'exec') namespace = {} exec(new_func, namespace) return namespace[func.__name__] @validate_types def greet(name: str, times: int): for _ in range(times): print(f"Hello, {name}!") greet("Alice", 3) # This works greet("Bob", "not a number") # This raises a TypeError
This transformer looks for print statements using the old %-formatting and converts them to use f-strings. It's a bit complex because it needs to handle different cases, but it shows the power of ASTs for automated refactoring.
One thing to keep in mind when working with ASTs is that they can be a bit finicky. You need to make sure all the nodes in your AST are properly set up, or you'll get errors when you try to compile or execute the code. The ast.fix_missing_locations() function is your friend here – it fills in any missing position information in your AST.
Also, while ASTs are powerful, they're not always the best tool for the job. For simple string manipulations or regexp-based changes, you might be better off with simpler methods. ASTs shine when you need to understand or manipulate the structure of the code itself.
In conclusion, Abstract Syntax Trees are a powerful tool in your Python metaprogramming toolkit. They let you analyze, transform, and generate code in ways that would be difficult or impossible with other methods. Whether you're optimizing performance, creating custom language features, or building tools for code analysis and refactoring, ASTs give you the power to work with Python code at a fundamental level. It's like having a superpower for your Python programs!
Our Creations
Be sure to check out our creations:
Investor Central | Smart Living | Epochs & Echoes | Puzzling Mysteries | Hindutva | Elite Dev | JS Schools
We are on Medium
Tech Koala Insights | Epochs & Echoes World | Investor Central Medium | Puzzling Mysteries Medium | Science & Epochs Medium | Modern Hindutva
The above is the detailed content of Unlock Pythons Hidden Power: Master Abstract Syntax Trees for Code Wizardry. For more information, please follow other related articles on the PHP Chinese website!

Hot AI Tools

Undresser.AI Undress
AI-powered app for creating realistic nude photos

AI Clothes Remover
Online AI tool for removing clothes from photos.

Undress AI Tool
Undress images for free

Clothoff.io
AI clothes remover

Video Face Swap
Swap faces in any video effortlessly with our completely free AI face swap tool!

Hot Article

Hot Tools

Notepad++7.3.1
Easy-to-use and free code editor

SublimeText3 Chinese version
Chinese version, very easy to use

Zend Studio 13.0.1
Powerful PHP integrated development environment

Dreamweaver CS6
Visual web development tools

SublimeText3 Mac version
God-level code editing software (SublimeText3)

Hot Topics










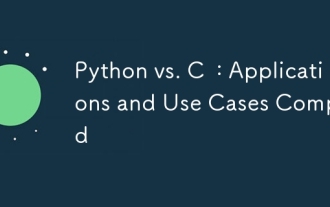
Python is suitable for data science, web development and automation tasks, while C is suitable for system programming, game development and embedded systems. Python is known for its simplicity and powerful ecosystem, while C is known for its high performance and underlying control capabilities.
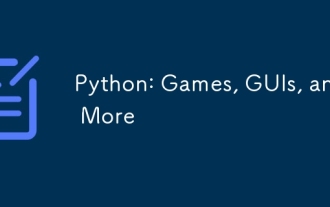
Python excels in gaming and GUI development. 1) Game development uses Pygame, providing drawing, audio and other functions, which are suitable for creating 2D games. 2) GUI development can choose Tkinter or PyQt. Tkinter is simple and easy to use, PyQt has rich functions and is suitable for professional development.
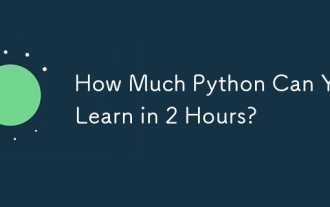
You can learn the basics of Python within two hours. 1. Learn variables and data types, 2. Master control structures such as if statements and loops, 3. Understand the definition and use of functions. These will help you start writing simple Python programs.
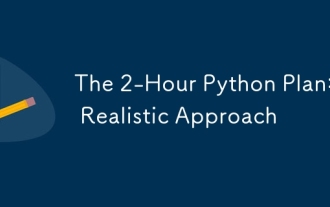
You can learn basic programming concepts and skills of Python within 2 hours. 1. Learn variables and data types, 2. Master control flow (conditional statements and loops), 3. Understand the definition and use of functions, 4. Quickly get started with Python programming through simple examples and code snippets.
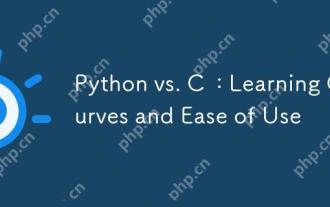
Python is easier to learn and use, while C is more powerful but complex. 1. Python syntax is concise and suitable for beginners. Dynamic typing and automatic memory management make it easy to use, but may cause runtime errors. 2.C provides low-level control and advanced features, suitable for high-performance applications, but has a high learning threshold and requires manual memory and type safety management.
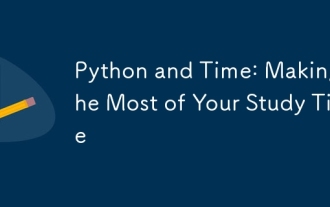
To maximize the efficiency of learning Python in a limited time, you can use Python's datetime, time, and schedule modules. 1. The datetime module is used to record and plan learning time. 2. The time module helps to set study and rest time. 3. The schedule module automatically arranges weekly learning tasks.
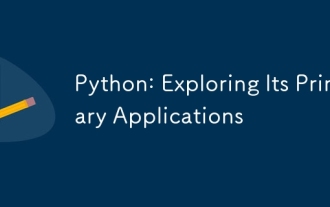
Python is widely used in the fields of web development, data science, machine learning, automation and scripting. 1) In web development, Django and Flask frameworks simplify the development process. 2) In the fields of data science and machine learning, NumPy, Pandas, Scikit-learn and TensorFlow libraries provide strong support. 3) In terms of automation and scripting, Python is suitable for tasks such as automated testing and system management.
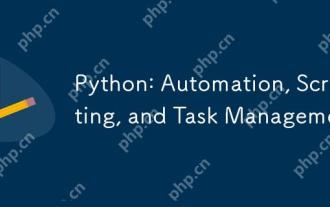
Python excels in automation, scripting, and task management. 1) Automation: File backup is realized through standard libraries such as os and shutil. 2) Script writing: Use the psutil library to monitor system resources. 3) Task management: Use the schedule library to schedule tasks. Python's ease of use and rich library support makes it the preferred tool in these areas.
