


Why does my Keras model seem to only use part of my Fashion MNIST dataset during training, even though it\'s processing 1875 batches?
Keras Training Limitations: Resolving Partial Dataset Usage
When training a neural network model using Keras, it's crucial to ensure that the entire dataset is utilized during training. However, in some instances, users may encounter issues where only a fraction of the data is used. This article explores a specific case where a model trained on the Fashion MNIST dataset uses only a portion of the available data, providing a comprehensive explanation and solution.
The provided code snippet utilizes the model.fit() method with default parameters, which includes a batch size of 32. This means that during each iteration or epoch, the model processes 32 samples from the training dataset. In the case of the Fashion MNIST dataset, which consists of 60,000 samples, the model would need to iterate through the entire dataset multiple times to complete training. However, the output shown in the console indicates that the model is completing one epoch in 1875 iterations.
This discrepancy arises because the model.fit() method reports the number of batches processed during training, not the total number of samples. Therefore, in this case, the model is training on 1875 batches, each containing 32 samples, resulting in a total of 1875 * 32 = 60,000 samples. This means that the model is indeed utilizing the entire dataset for training, despite the misleading progress bar that displays "1875/1875" during each epoch.
To avoid confusion and accurately track the progress of the training process, it is recommended to calculate and display the number of samples processed per epoch. This can be achieved by modifying the code to print the progress as follows:
<code class="python">for epoch in range(10): print(f"Current Epoch: {epoch + 1}") for batch_idx in range(1875): model.train_step((train_images[batch_idx * 32 : (batch_idx + 1) * 32], train_labels[batch_idx * 32 : (batch_idx + 1) * 32])) print(f"Batch {batch_idx + 1} processed.")</code>
Using this approach, the console will display the progress in terms of both batches and samples, providing a clear understanding of the training process and confirming that the model is utilizing the entire dataset as intended.
The above is the detailed content of Why does my Keras model seem to only use part of my Fashion MNIST dataset during training, even though it\'s processing 1875 batches?. For more information, please follow other related articles on the PHP Chinese website!

Hot AI Tools

Undresser.AI Undress
AI-powered app for creating realistic nude photos

AI Clothes Remover
Online AI tool for removing clothes from photos.

Undress AI Tool
Undress images for free

Clothoff.io
AI clothes remover

Video Face Swap
Swap faces in any video effortlessly with our completely free AI face swap tool!

Hot Article

Hot Tools

Notepad++7.3.1
Easy-to-use and free code editor

SublimeText3 Chinese version
Chinese version, very easy to use

Zend Studio 13.0.1
Powerful PHP integrated development environment

Dreamweaver CS6
Visual web development tools

SublimeText3 Mac version
God-level code editing software (SublimeText3)

Hot Topics










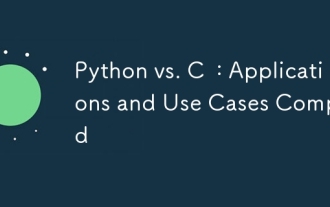
Python is suitable for data science, web development and automation tasks, while C is suitable for system programming, game development and embedded systems. Python is known for its simplicity and powerful ecosystem, while C is known for its high performance and underlying control capabilities.
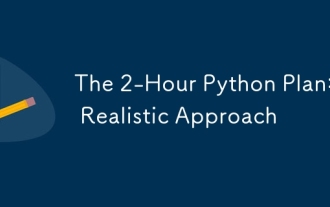
You can learn basic programming concepts and skills of Python within 2 hours. 1. Learn variables and data types, 2. Master control flow (conditional statements and loops), 3. Understand the definition and use of functions, 4. Quickly get started with Python programming through simple examples and code snippets.
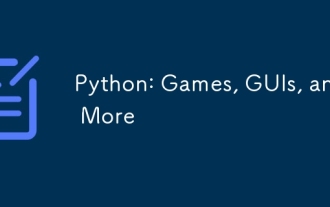
Python excels in gaming and GUI development. 1) Game development uses Pygame, providing drawing, audio and other functions, which are suitable for creating 2D games. 2) GUI development can choose Tkinter or PyQt. Tkinter is simple and easy to use, PyQt has rich functions and is suitable for professional development.
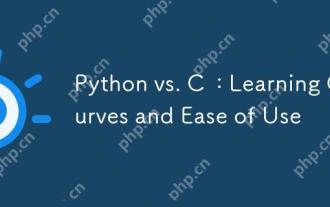
Python is easier to learn and use, while C is more powerful but complex. 1. Python syntax is concise and suitable for beginners. Dynamic typing and automatic memory management make it easy to use, but may cause runtime errors. 2.C provides low-level control and advanced features, suitable for high-performance applications, but has a high learning threshold and requires manual memory and type safety management.
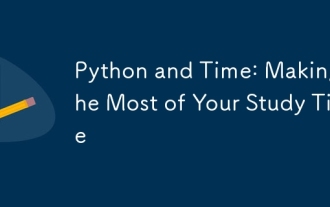
To maximize the efficiency of learning Python in a limited time, you can use Python's datetime, time, and schedule modules. 1. The datetime module is used to record and plan learning time. 2. The time module helps to set study and rest time. 3. The schedule module automatically arranges weekly learning tasks.
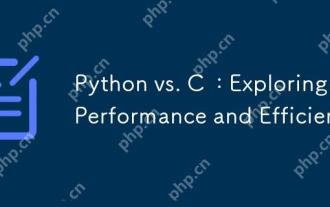
Python is better than C in development efficiency, but C is higher in execution performance. 1. Python's concise syntax and rich libraries improve development efficiency. 2.C's compilation-type characteristics and hardware control improve execution performance. When making a choice, you need to weigh the development speed and execution efficiency based on project needs.
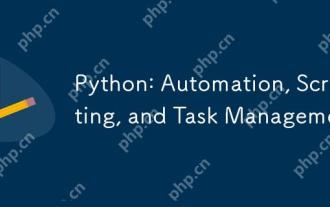
Python excels in automation, scripting, and task management. 1) Automation: File backup is realized through standard libraries such as os and shutil. 2) Script writing: Use the psutil library to monitor system resources. 3) Task management: Use the schedule library to schedule tasks. Python's ease of use and rich library support makes it the preferred tool in these areas.
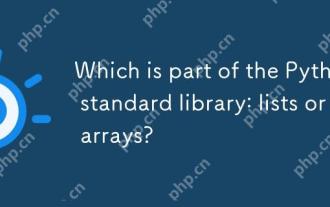
Pythonlistsarepartofthestandardlibrary,whilearraysarenot.Listsarebuilt-in,versatile,andusedforstoringcollections,whereasarraysareprovidedbythearraymoduleandlesscommonlyusedduetolimitedfunctionality.
