ETL: Extracting a Persons Name from Text
Let's say we want to scrape chicagomusiccompass.com.
As you can see, it has several cards, each representing an event. Now, let's check out the next one:
Notice that the name of the event is:
jazmin bean: the traumatic livelihood tour
So now the question is: How do we extract the artist's name from the text?
As a human, I can "easily" tell that jazmin bean is the artist—just check out their wiki page. But writing code to extract that name can get tricky.
We could think, "Hey, anything before the : should be the artist's name," which seems clever, right? It works for this case, but what about this one:
happy hour on the patio: kathryn & chris
Here, the order is flipped. We could keep adding logic to handle different cases, but soon we'll end up with a ton of rules that are fragile and probably won't cover everything.
That’s where Named Entity Recognition (NER) models come in handy. They’re open source and can help us extract names from text. It won’t catch every case, but most of the time, they’ll get us the info we need.
With this approach, the extraction becomes way easier. I'm going with Python because the community around Machine Learning in Python is just unbeatable.
from gliner import GLiNER model = GLiNER.from_pretrained("urchade/gliner_base") text = "jazmin bean: the traumatic livelihood tour" labels = ["person", "bands", "projects"] entities = model.predict_entities(text, labels) for entity in entities: print(entity["text"], "=>", entity["label"])
Which generates the output:
jazmin bean => person
Now, let’s take a look at that other case:
happy hour on the patio: kathryn & chris
Output:
kathryn => person chris => person
source-GLiNER
Awesome, right? No more tedious logic to extract names, just use a model. Sure, it won’t cover every possible case, but for my project, this level of flexibility works just fine. If you need more accuracy, you can always:
- Try a different model
- Contribute to the existing model
- Fork the project and tweak it to fit your needs
Conclusion
As a Software Developer, it's highly recommended to stay updated with the tools in the Machine Learning space. Not everything can be solved with just plain programming and logic—some challenges are better tackled using models and statistics.
The above is the detailed content of ETL: Extracting a Persons Name from Text. For more information, please follow other related articles on the PHP Chinese website!

Hot AI Tools

Undresser.AI Undress
AI-powered app for creating realistic nude photos

AI Clothes Remover
Online AI tool for removing clothes from photos.

Undress AI Tool
Undress images for free

Clothoff.io
AI clothes remover

Video Face Swap
Swap faces in any video effortlessly with our completely free AI face swap tool!

Hot Article

Hot Tools

Notepad++7.3.1
Easy-to-use and free code editor

SublimeText3 Chinese version
Chinese version, very easy to use

Zend Studio 13.0.1
Powerful PHP integrated development environment

Dreamweaver CS6
Visual web development tools

SublimeText3 Mac version
God-level code editing software (SublimeText3)

Hot Topics










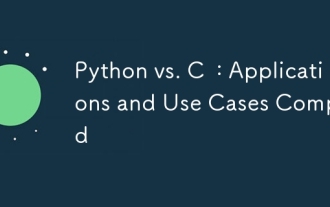
Python is suitable for data science, web development and automation tasks, while C is suitable for system programming, game development and embedded systems. Python is known for its simplicity and powerful ecosystem, while C is known for its high performance and underlying control capabilities.
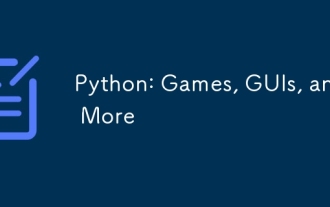
Python excels in gaming and GUI development. 1) Game development uses Pygame, providing drawing, audio and other functions, which are suitable for creating 2D games. 2) GUI development can choose Tkinter or PyQt. Tkinter is simple and easy to use, PyQt has rich functions and is suitable for professional development.
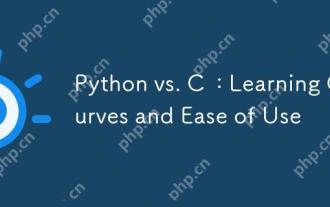
Python is easier to learn and use, while C is more powerful but complex. 1. Python syntax is concise and suitable for beginners. Dynamic typing and automatic memory management make it easy to use, but may cause runtime errors. 2.C provides low-level control and advanced features, suitable for high-performance applications, but has a high learning threshold and requires manual memory and type safety management.
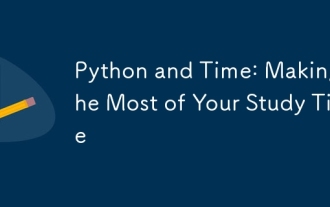
To maximize the efficiency of learning Python in a limited time, you can use Python's datetime, time, and schedule modules. 1. The datetime module is used to record and plan learning time. 2. The time module helps to set study and rest time. 3. The schedule module automatically arranges weekly learning tasks.
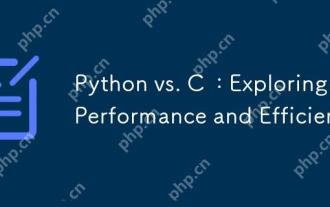
Python is better than C in development efficiency, but C is higher in execution performance. 1. Python's concise syntax and rich libraries improve development efficiency. 2.C's compilation-type characteristics and hardware control improve execution performance. When making a choice, you need to weigh the development speed and execution efficiency based on project needs.
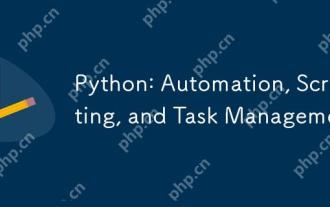
Python excels in automation, scripting, and task management. 1) Automation: File backup is realized through standard libraries such as os and shutil. 2) Script writing: Use the psutil library to monitor system resources. 3) Task management: Use the schedule library to schedule tasks. Python's ease of use and rich library support makes it the preferred tool in these areas.
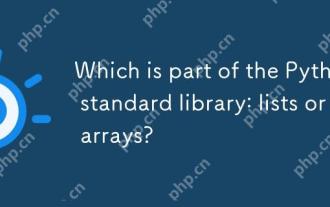
Pythonlistsarepartofthestandardlibrary,whilearraysarenot.Listsarebuilt-in,versatile,andusedforstoringcollections,whereasarraysareprovidedbythearraymoduleandlesscommonlyusedduetolimitedfunctionality.
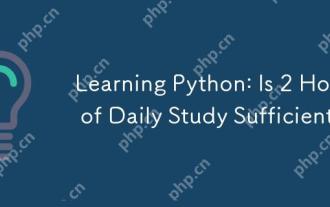
Is it enough to learn Python for two hours a day? It depends on your goals and learning methods. 1) Develop a clear learning plan, 2) Select appropriate learning resources and methods, 3) Practice and review and consolidate hands-on practice and review and consolidate, and you can gradually master the basic knowledge and advanced functions of Python during this period.
