Introduction to python for data analysis
What is Python?
Python is a popular programming language. It was created by Guido van Rossum, and released in 1991.
It is used for:
- web development (server-side),
- software development,
- mathematics,
- system scripting.
*What can Python do?
*
- Python can be used on a server to create web applications.
- Python can be used alongside software to create workflows.
- Python can connect to database systems. It can also read and modify files.
- Python can be used to handle big data and perform complex mathematics.
Python can be used for rapid prototyping, or for production-ready software development
.
Why Python?Python works on different platforms (Windows, Mac, Linux, Raspberry Pi, etc).
Python has a simple syntax similar to the English language.
Python has syntax that allows developers to write programs with fewer lines than some other programming languages.
Python runs on an interpreter system, meaning that code can be executed as soon as it is written. This means that prototyping can be very quick.
Python can be treated in a procedural way, an object-oriented way or a functional way.
**
Why Use Python for Data Analysis?
**
Ease of Learning: Python’s syntax is clear and intuitive, making it accessible for beginners.
Rich Libraries: Python offers powerful libraries specifically designed for data analysis, such as:
Pandas: For data manipulation and analysis.
NumPy: For numerical computations.
Matplotlib & Seaborn: For data visualization.
SciPy: For scientific and technical computing.
Statsmodels: For statistical modeling.
Community and Resources: A large community means plenty of resources, tutorials, and forums for support.
Key Libraries for Data Analysis
Pandas
Used for data manipulation and analysis.
Offers data structures like DataFrames and Series, which simplify handling and analyzing structured data.
Common operations include filtering, grouping, aggregating, and merging datasets.
python
Copy code
import pandas as pd
Load a dataset
df = pd.read_csv('data.csv')
Display the first few rows
print(df.head())
NumPy
Provides support for large, multi-dimensional arrays and matrices.
Offers mathematical functions to operate on these arrays.
python
Copy code
import numpy as np
Create a NumPy array
array = np.array([1, 2, 3, 4])
Matplotlib & Seaborn
Matplotlib: The foundational library for creating static, interactive, and animated visualizations in Python.
Seaborn: Built on top of Matplotlib, it provides a higher-level interface for drawing attractive statistical graphics.
python
Copy code
import matplotlib.pyplot as plt
import seaborn as sns
Create a simple line plot
plt.plot(df['column1'], df['column2'])
plt.show()
SciPy
Built on NumPy, it provides additional functionality for optimization, integration, interpolation, eigenvalue problems, and other advanced mathematical computations.
Statsmodels
**
Useful for statistical modeling and hypothesis testing.
**
Provides tools for regression analysis, time series analysis, and more.
Basic Data Analysis Workflow
Data Collection: Gather data from various sources, such as CSV files, databases, or web scraping.
Data Cleaning: Handle missing values, duplicates, and inconsistencies.
Exploratory Data Analysis (EDA): Analyze the data through summary statistics and visualizations to understand its structure and patterns.
Data Manipulation: Transform the data as needed for analysis (e.g., filtering, aggregating).
Modeling: Apply statistical or machine learning models to derive insights or make predictions.
Visualization: Create plots to effectively communicate findings.
Reporting: Summarize results in a clear format for stakeholders.
Conclusion
Python's robust ecosystem makes it an excellent choice for data analysis. By leveraging libraries like Pandas, NumPy, Matplotlib, and others, you can efficiently manipulate, analyze, and visualize data. Whether you're a beginner or an experienced analyst, mastering Python will enhance your ability to derive insights from data.
The above is the detailed content of Introduction to python for data analysis. For more information, please follow other related articles on the PHP Chinese website!

Hot AI Tools

Undresser.AI Undress
AI-powered app for creating realistic nude photos

AI Clothes Remover
Online AI tool for removing clothes from photos.

Undress AI Tool
Undress images for free

Clothoff.io
AI clothes remover

Video Face Swap
Swap faces in any video effortlessly with our completely free AI face swap tool!

Hot Article

Hot Tools

Notepad++7.3.1
Easy-to-use and free code editor

SublimeText3 Chinese version
Chinese version, very easy to use

Zend Studio 13.0.1
Powerful PHP integrated development environment

Dreamweaver CS6
Visual web development tools

SublimeText3 Mac version
God-level code editing software (SublimeText3)

Hot Topics










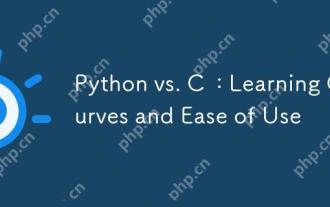
Python is easier to learn and use, while C is more powerful but complex. 1. Python syntax is concise and suitable for beginners. Dynamic typing and automatic memory management make it easy to use, but may cause runtime errors. 2.C provides low-level control and advanced features, suitable for high-performance applications, but has a high learning threshold and requires manual memory and type safety management.
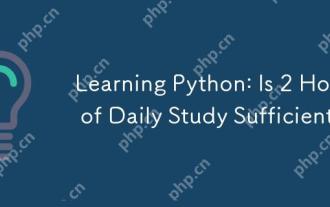
Is it enough to learn Python for two hours a day? It depends on your goals and learning methods. 1) Develop a clear learning plan, 2) Select appropriate learning resources and methods, 3) Practice and review and consolidate hands-on practice and review and consolidate, and you can gradually master the basic knowledge and advanced functions of Python during this period.
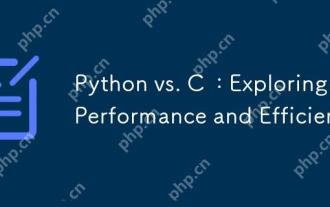
Python is better than C in development efficiency, but C is higher in execution performance. 1. Python's concise syntax and rich libraries improve development efficiency. 2.C's compilation-type characteristics and hardware control improve execution performance. When making a choice, you need to weigh the development speed and execution efficiency based on project needs.
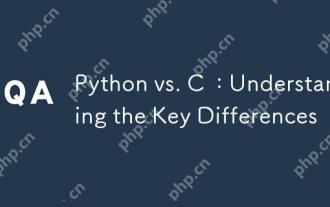
Python and C each have their own advantages, and the choice should be based on project requirements. 1) Python is suitable for rapid development and data processing due to its concise syntax and dynamic typing. 2)C is suitable for high performance and system programming due to its static typing and manual memory management.
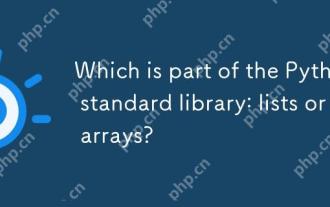
Pythonlistsarepartofthestandardlibrary,whilearraysarenot.Listsarebuilt-in,versatile,andusedforstoringcollections,whereasarraysareprovidedbythearraymoduleandlesscommonlyusedduetolimitedfunctionality.
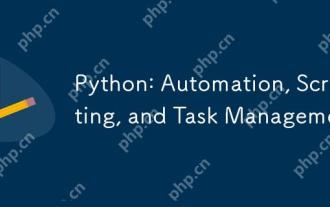
Python excels in automation, scripting, and task management. 1) Automation: File backup is realized through standard libraries such as os and shutil. 2) Script writing: Use the psutil library to monitor system resources. 3) Task management: Use the schedule library to schedule tasks. Python's ease of use and rich library support makes it the preferred tool in these areas.
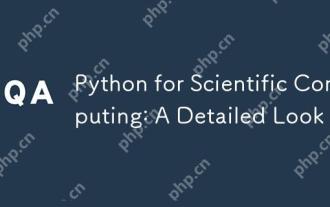
Python's applications in scientific computing include data analysis, machine learning, numerical simulation and visualization. 1.Numpy provides efficient multi-dimensional arrays and mathematical functions. 2. SciPy extends Numpy functionality and provides optimization and linear algebra tools. 3. Pandas is used for data processing and analysis. 4.Matplotlib is used to generate various graphs and visual results.
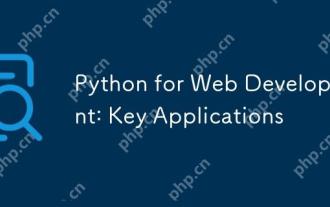
Key applications of Python in web development include the use of Django and Flask frameworks, API development, data analysis and visualization, machine learning and AI, and performance optimization. 1. Django and Flask framework: Django is suitable for rapid development of complex applications, and Flask is suitable for small or highly customized projects. 2. API development: Use Flask or DjangoRESTFramework to build RESTfulAPI. 3. Data analysis and visualization: Use Python to process data and display it through the web interface. 4. Machine Learning and AI: Python is used to build intelligent web applications. 5. Performance optimization: optimized through asynchronous programming, caching and code
