Using LLaMA Models with Groq: A Beginners Guide
Hey there, AI enthusiasts! Today, we're going to learn how to use LLaMA models with Groq. It's easier than you might think, and I'll guide you step-by-step on how to get started.
In this blog, we will explore how to use free AI models, discuss running them locally, and leverage Groq for API-powered applications. Whether you're building a text-based game or an AI-powered app, this guide will cover everything you need.
What You'll Need
- Python installed on your computer
- A Groq API key (you can get one from their website)
- Basic knowledge of Python (but don't worry, we'll keep it simple!)
- A curiosity to explore AI in creative ways!
Step 1: Set Up Your Environment
First, let's install the Groq library. Open your terminal and run:
pip install groq
Step 2: Import the Library and Set Up Your API Key
Now, let's write some Python code. Create a new file called llama_groq_test.py and add these lines:
import os from groq import Groq # Set your API key api_key = os.environ.get("GROQ_API_KEY") if not api_key: api_key = input("Please enter your Groq API key: ") os.environ["GROQ_API_KEY"] = api_key # Create a client client = Groq()
This method is more secure as it doesn't hardcode the API key directly in your script.
Step 3: Choose Your Model
Groq supports different LLaMA models. For this example, we'll use "llama2-70b-4096". Let's add this to our code:
model = "llama2-70b-4096"
Step 4: Send a Message and Get a Response
Now for the fun part! Let's ask LLaMA a question. Add this to your code:
# Define your message messages = [ { "role": "user", "content": "What's the best way to learn programming?", } ] # Send the message and get the response chat_completion = client.chat.completions.create( messages=messages, model=model, temperature=0.7, max_tokens=1000, ) # Print the response print(chat_completion.choices[0].message.content)
Step 5: Run Your Code
Save your file and run it from the terminal:
python llama_groq_test.py
You should see LLaMA's response printed out!
Bonus: Having a Conversation
Want to have a back-and-forth chat? Here's a simple way to do it:
while True: user_input = input("You: ") if user_input.lower() == 'quit': break messages.append({"role": "user", "content": user_input}) chat_completion = client.chat.completions.create( messages=messages, model=model, temperature=0.7, max_tokens=1000, ) ai_response = chat_completion.choices[0].message.content print("AI:", ai_response) messages.append({"role": "assistant", "content": ai_response})
This code creates a loop where you can keep chatting with LLaMA until you type 'quit'.
Free AI Options: Running LLaMA Locally
Many developers prefer free, open-source models like LLaMA by Meta because they can be run locally without costly API charges. While using APIs like OpenAI or Gemini can be convenient, the open-source nature of LLaMA offers more control and flexibility.
It's important to note that running LLaMA models locally often requires significant computational resources, especially for larger models. However, for those with the right hardware, this can lead to substantial savings, especially when running your projects without worrying about API costs.
You can test smaller LLaMA models on your local machine. For larger-scale projects or if you lack the necessary hardware, tools like Groq provide a simple way to integrate AI with just an API key.
Star Quest: My AI-Powered Sci-Fi Game
Speaking of AI-powered projects, I recently built a sci-fi text-based game called Star Quest using LLaMA (via Groq's API) and Next.js. The game allows players to explore a narrative-driven world, making choices that affect the storyline.
Here's a sneak peek into how it works:
- The user inputs a choice to guide the story.
- LLaMA processes the user's input, generating a dynamic response that shapes the next part of the plot.
- The game's logic and API integration allow for endless combinations, making it a truly interactive experience.
If you'd like to see the full project and try it out yourself, check out my GitHub repo here: https://github.com/Mohiit70/Star-Quest
You can clone the repository and start exploring sci-fi narratives powered by AI!
Wrapping Up
That's it! You now know how to use LLaMA with Groq to create AI-powered apps or even build your own games. Here's a quick summary:
- Install the Groq library.
- Set up your API key securely.
- Choose the LLaMA model.
- Send and receive messages from the AI.
- Experiment with creating your own AI-based applications, like my Star Quest text-based game.
I hope this guide has inspired you to explore the world of AI. Feel free to ask any questions or check out my Star Quest project on GitHub!
Happy Coding!
The above is the detailed content of Using LLaMA Models with Groq: A Beginners Guide. For more information, please follow other related articles on the PHP Chinese website!

Hot AI Tools

Undresser.AI Undress
AI-powered app for creating realistic nude photos

AI Clothes Remover
Online AI tool for removing clothes from photos.

Undress AI Tool
Undress images for free

Clothoff.io
AI clothes remover

Video Face Swap
Swap faces in any video effortlessly with our completely free AI face swap tool!

Hot Article

Hot Tools

Notepad++7.3.1
Easy-to-use and free code editor

SublimeText3 Chinese version
Chinese version, very easy to use

Zend Studio 13.0.1
Powerful PHP integrated development environment

Dreamweaver CS6
Visual web development tools

SublimeText3 Mac version
God-level code editing software (SublimeText3)

Hot Topics










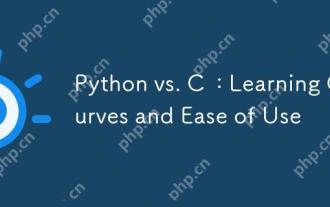
Python is easier to learn and use, while C is more powerful but complex. 1. Python syntax is concise and suitable for beginners. Dynamic typing and automatic memory management make it easy to use, but may cause runtime errors. 2.C provides low-level control and advanced features, suitable for high-performance applications, but has a high learning threshold and requires manual memory and type safety management.
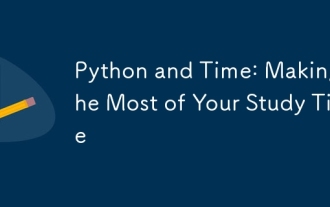
To maximize the efficiency of learning Python in a limited time, you can use Python's datetime, time, and schedule modules. 1. The datetime module is used to record and plan learning time. 2. The time module helps to set study and rest time. 3. The schedule module automatically arranges weekly learning tasks.
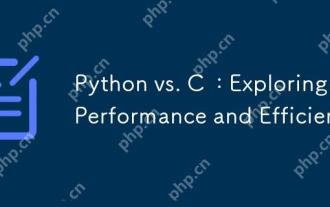
Python is better than C in development efficiency, but C is higher in execution performance. 1. Python's concise syntax and rich libraries improve development efficiency. 2.C's compilation-type characteristics and hardware control improve execution performance. When making a choice, you need to weigh the development speed and execution efficiency based on project needs.
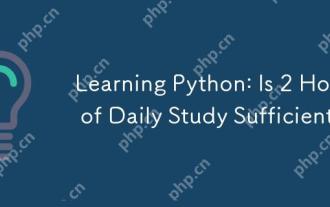
Is it enough to learn Python for two hours a day? It depends on your goals and learning methods. 1) Develop a clear learning plan, 2) Select appropriate learning resources and methods, 3) Practice and review and consolidate hands-on practice and review and consolidate, and you can gradually master the basic knowledge and advanced functions of Python during this period.
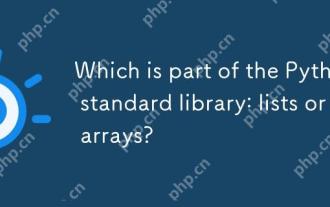
Pythonlistsarepartofthestandardlibrary,whilearraysarenot.Listsarebuilt-in,versatile,andusedforstoringcollections,whereasarraysareprovidedbythearraymoduleandlesscommonlyusedduetolimitedfunctionality.
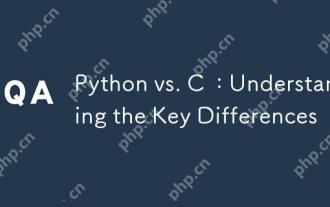
Python and C each have their own advantages, and the choice should be based on project requirements. 1) Python is suitable for rapid development and data processing due to its concise syntax and dynamic typing. 2)C is suitable for high performance and system programming due to its static typing and manual memory management.
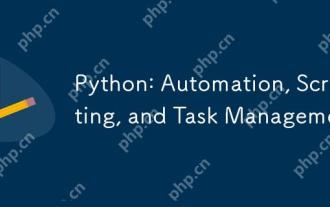
Python excels in automation, scripting, and task management. 1) Automation: File backup is realized through standard libraries such as os and shutil. 2) Script writing: Use the psutil library to monitor system resources. 3) Task management: Use the schedule library to schedule tasks. Python's ease of use and rich library support makes it the preferred tool in these areas.
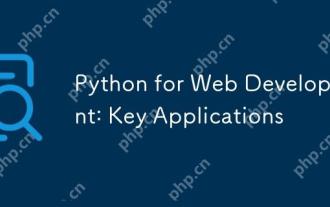
Key applications of Python in web development include the use of Django and Flask frameworks, API development, data analysis and visualization, machine learning and AI, and performance optimization. 1. Django and Flask framework: Django is suitable for rapid development of complex applications, and Flask is suitable for small or highly customized projects. 2. API development: Use Flask or DjangoRESTFramework to build RESTfulAPI. 3. Data analysis and visualization: Use Python to process data and display it through the web interface. 4. Machine Learning and AI: Python is used to build intelligent web applications. 5. Performance optimization: optimized through asynchronous programming, caching and code
