


Mastering CRUD Operations with OpenSearch in Python: A Practical Guide
OpenSearch, an open-source alternative to Elasticsearch, is a powerful search and analytics engine built to handle large datasets with ease. In this blog, we’ll demonstrate how to perform basic CRUD (Create, Read, Update, Delete) operations in OpenSearch using Python.
Prerequisites:
- Python 3.7+
- OpenSearch installed locally using Docker
- Familiarity with RESTful APIs
Step 1: Setting Up OpenSearch Locally with Docker
To get started, we need a local OpenSearch instance. Below is a simple docker-compose.yml file that spins up OpenSearch and OpenSearch Dashboards.
version: '3' services: opensearch-test-node-1: image: opensearchproject/opensearch:2.13.0 container_name: opensearch-test-node-1 environment: - cluster.name=opensearch-test-cluster - node.name=opensearch-test-node-1 - discovery.seed_hosts=opensearch-test-node-1,opensearch-test-node-2 - cluster.initial_cluster_manager_nodes=opensearch-test-node-1,opensearch-test-node-2 - bootstrap.memory_lock=true - "OPENSEARCH_JAVA_OPTS=-Xms512m -Xmx512m" - "DISABLE_INSTALL_DEMO_CONFIG=true" - "DISABLE_SECURITY_PLUGIN=true" ulimits: memlock: soft: -1 hard: -1 nofile: soft: 65536 hard: 65536 volumes: - opensearch-test-data1:/usr/share/opensearch/data ports: - 9200:9200 - 9600:9600 networks: - opensearch-test-net opensearch-test-node-2: image: opensearchproject/opensearch:2.13.0 container_name: opensearch-test-node-2 environment: - cluster.name=opensearch-test-cluster - node.name=opensearch-test-node-2 - discovery.seed_hosts=opensearch-test-node-1,opensearch-test-node-2 - cluster.initial_cluster_manager_nodes=opensearch-test-node-1,opensearch-test-node-2 - bootstrap.memory_lock=true - "OPENSEARCH_JAVA_OPTS=-Xms512m -Xmx512m" - "DISABLE_INSTALL_DEMO_CONFIG=true" - "DISABLE_SECURITY_PLUGIN=true" ulimits: memlock: soft: -1 hard: -1 nofile: soft: 65536 hard: 65536 volumes: - opensearch-test-data2:/usr/share/opensearch/data networks: - opensearch-test-net opensearch-test-dashboards: image: opensearchproject/opensearch-dashboards:2.13.0 container_name: opensearch-test-dashboards ports: - 5601:5601 expose: - "5601" environment: - 'OPENSEARCH_HOSTS=["http://opensearch-test-node-1:9200","http://opensearch-test-node-2:9200"]' - "DISABLE_SECURITY_DASHBOARDS_PLUGIN=true" networks: - opensearch-test-net volumes: opensearch-test-data1: opensearch-test-data2: networks: opensearch-test-net:
Run the following command to bring up your OpenSearch instance:
docker-compose up
OpenSearch will be accessible at http://localhost:9200.
Step 2: Setting Up the Python Environment
python -m venv .venv source .venv/bin/activate pip install opensearch-py
We'll also structure our project as follows:
├── interfaces.py ├── main.py ├── searchservice.py ├── docker-compose.yml
Step 3: Defining Interfaces and Resources (interfaces.py)
In the interfaces.py file, we define our Resource and Resources classes. These will help us dynamically handle different resource types in OpenSearch (in this case, users).
from dataclasses import dataclass, field @dataclass class Resource: name: str def __post_init__(self) -> None: self.name = self.name.lower() @dataclass class Resources: users: Resource = field(default_factory=lambda: Resource("Users"))
Step 4: CRUD Operations with OpenSearch (searchservice.py)
In searchservice.py, we define an abstract class SearchService to outline the required operations. The HTTPOpenSearchService class then implements these CRUD methods, interacting with the OpenSearch client.
# coding: utf-8 import abc import logging import typing as t from dataclasses import dataclass from uuid import UUID from interfaces import Resource, Resources from opensearchpy import NotFoundError, OpenSearch resources = Resources() class SearchService(abc.ABC): def search( self, kinds: t.List[Resource], tenants_id: UUID, companies_id: UUID, query: t.Dict[str, t.Any], ) -> t.Dict[t.Literal["hits"], t.Dict[str, t.Any]]: raise NotImplementedError def delete_index( self, kind: Resource, tenants_id: UUID, companies_id: UUID, data: t.Dict[str, t.Any], ) -> None: raise NotImplementedError def index( self, kind: Resource, tenants_id: UUID, companies_id: UUID, data: t.Dict[str, t.Any], ) -> t.Dict[str, t.Any]: raise NotImplementedError def delete_document( self, kind: Resource, tenants_id: UUID, companies_id: UUID, document_id: str, ) -> t.Optional[t.Dict[str, t.Any]]: raise NotImplementedError def create_index( self, kind: Resource, tenants_id: UUID, companies_id: UUID, data: t.Dict[str, t.Any], ) -> None: raise NotImplementedError @dataclass(frozen=True) class HTTPOpenSearchService(SearchService): client: OpenSearch def _gen_index( self, kind: Resource, tenants_id: UUID, companies_id: UUID, ) -> str: return ( f"tenant_{str(UUID(str(tenants_id)))}" f"_company_{str(UUID(str(companies_id)))}" f"_kind_{kind.name}" ) def index( self, kind: Resource, tenants_id: UUID, companies_id: UUID, data: t.Dict[str, t.Any], ) -> t.Dict[str, t.Any]: self.client.index( index=self._gen_index(kind, tenants_id, companies_id), body=data, id=data.get("id"), ) return data def delete_index( self, kind: Resource, tenants_id: UUID, companies_id: UUID, ) -> None: try: index = self._gen_index(kind, tenants_id, companies_id) if self.client.indices.exists(index): self.client.indices.delete(index) except NotFoundError: pass def create_index( self, kind: Resource, tenants_id: UUID, companies_id: UUID, ) -> None: body: t.Dict[str, t.Any] = {} self.client.indices.create( index=self._gen_index(kind, tenants_id, companies_id), body=body, ) def search( self, kinds: t.List[Resource], tenants_id: UUID, companies_id: UUID, query: t.Dict[str, t.Any], ) -> t.Dict[t.Literal["hits"], t.Dict[str, t.Any]]: return self.client.search( index=",".join( [self._gen_index(kind, tenants_id, companies_id) for kind in kinds] ), body={"query": query}, ) def delete_document( self, kind: Resource, tenants_id: UUID, companies_id: UUID, document_id: str, ) -> t.Optional[t.Dict[str, t.Any]]: try: response = self.client.delete( index=self._gen_index(kind, tenants_id, companies_id), id=document_id, ) return response except Exception as e: logging.error(f"Error deleting document: {e}") return None
Step 5: Implementing CRUD in Main (main.py)
In main.py, we demonstrate how to:
- Create an index in OpenSearch.
- Index documents with sample user data.
- Search for documents based on a query.
- Delete a document using its ID.
main.py
# coding=utf-8 import logging import os import typing as t from uuid import uuid4 import searchservice from interfaces import Resources from opensearchpy import OpenSearch resources = Resources() logging.basicConfig(level=logging.INFO) search_service = searchservice.HTTPOpenSearchService( client=OpenSearch( hosts=[ { "host": os.getenv("OPENSEARCH_HOST", "localhost"), "port": os.getenv("OPENSEARCH_PORT", "9200"), } ], http_auth=( os.getenv("OPENSEARCH_USERNAME", ""), os.getenv("OPENSEARCH_PASSWORD", ""), ), use_ssl=False, verify_certs=False, ), ) tenants_id: str = "f0835e2d-bd68-406c-99a7-ad63a51e9ef9" companies_id: str = "bf58c749-c90a-41e2-b66f-6d98aae17a6c" search_str: str = "frank" document_id_to_delete: str = str(uuid4()) fake_data: t.List[t.Dict[str, t.Any]] = [ {"id": document_id_to_delete, "name": "Franklin", "tech": "python,node,golang"}, {"id": str(uuid4()), "name": "Jarvis", "tech": "AI"}, {"id": str(uuid4()), "name": "Parry", "tech": "Golang"}, {"id": str(uuid4()), "name": "Steve", "tech": "iOS"}, {"id": str(uuid4()), "name": "Frank", "tech": "node"}, ] search_service.delete_index( kind=resources.users, tenants_id=tenants_id, companies_id=companies_id ) search_service.create_index( kind=resources.users, tenants_id=tenants_id, companies_id=companies_id, ) for item in fake_data: search_service.index( kind=resources.users, tenants_id=tenants_id, companies_id=companies_id, data=dict(tenants_id=tenants_id, companies_id=companies_id, **item), ) search_query: t.Dict[str, t.Any] = { "bool": { "must": [], "must_not": [], "should": [], "filter": [ {"term": {"tenants_id.keyword": tenants_id}}, {"term": {"companies_id.keyword": companies_id}}, ], } } search_query["bool"]["must"].append( { "multi_match": { "query": search_str, "type": "phrase_prefix", "fields": ["name", "tech"], } } ) search_results = search_service.search( kinds=[resources.users], tenants_id=tenants_id, companies_id=companies_id, query=search_query, ) final_result = search_results.get("hits", {}).get("hits", []) for item in final_result: logging.info(["Item -> ", item.get("_source", {})]) deleted_result = search_service.delete_document( kind=resources.users, tenants_id=tenants_id, companies_id=companies_id, document_id=document_id_to_delete, ) logging.info(["Deleted result -> ", deleted_result])
Step 6: Running the project
docker compose up
python main.py
Results:
It should print found & deleted records information.
Step 7: Conclusion
In this blog, we’ve demonstrated how to set up OpenSearch locally using Docker and perform basic CRUD operations with Python. OpenSearch provides a powerful and scalable solution for managing and querying large datasets. While this guide focuses on integrating OpenSearch with dummy data, in real-world applications, OpenSearch is often used as a read-optimized store for faster data retrieval. In such cases, it is common to implement different indexing strategies to ensure data consistency by updating both the primary database and OpenSearch concurrently.
This ensures that OpenSearch remains in sync with your primary data source, optimizing both performance and accuracy in data retrieval.
References:
https://github.com/FranklinThaker/opensearch-integration-example
The above is the detailed content of Mastering CRUD Operations with OpenSearch in Python: A Practical Guide. For more information, please follow other related articles on the PHP Chinese website!

Hot AI Tools

Undresser.AI Undress
AI-powered app for creating realistic nude photos

AI Clothes Remover
Online AI tool for removing clothes from photos.

Undress AI Tool
Undress images for free

Clothoff.io
AI clothes remover

Video Face Swap
Swap faces in any video effortlessly with our completely free AI face swap tool!

Hot Article

Hot Tools

Notepad++7.3.1
Easy-to-use and free code editor

SublimeText3 Chinese version
Chinese version, very easy to use

Zend Studio 13.0.1
Powerful PHP integrated development environment

Dreamweaver CS6
Visual web development tools

SublimeText3 Mac version
God-level code editing software (SublimeText3)

Hot Topics
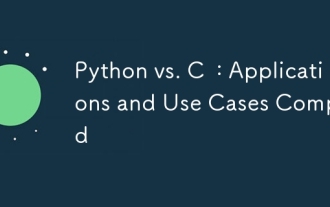
Python is suitable for data science, web development and automation tasks, while C is suitable for system programming, game development and embedded systems. Python is known for its simplicity and powerful ecosystem, while C is known for its high performance and underlying control capabilities.
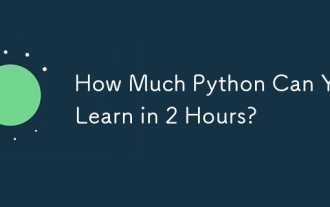
You can learn the basics of Python within two hours. 1. Learn variables and data types, 2. Master control structures such as if statements and loops, 3. Understand the definition and use of functions. These will help you start writing simple Python programs.
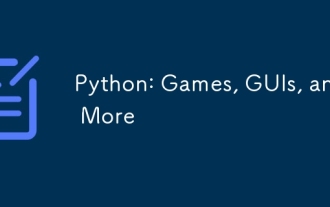
Python excels in gaming and GUI development. 1) Game development uses Pygame, providing drawing, audio and other functions, which are suitable for creating 2D games. 2) GUI development can choose Tkinter or PyQt. Tkinter is simple and easy to use, PyQt has rich functions and is suitable for professional development.
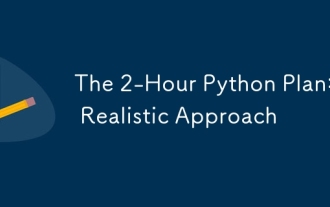
You can learn basic programming concepts and skills of Python within 2 hours. 1. Learn variables and data types, 2. Master control flow (conditional statements and loops), 3. Understand the definition and use of functions, 4. Quickly get started with Python programming through simple examples and code snippets.
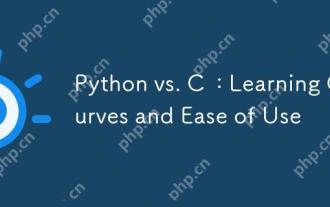
Python is easier to learn and use, while C is more powerful but complex. 1. Python syntax is concise and suitable for beginners. Dynamic typing and automatic memory management make it easy to use, but may cause runtime errors. 2.C provides low-level control and advanced features, suitable for high-performance applications, but has a high learning threshold and requires manual memory and type safety management.
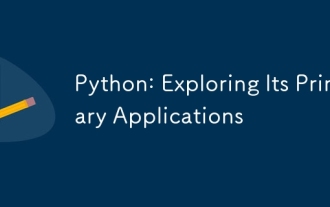
Python is widely used in the fields of web development, data science, machine learning, automation and scripting. 1) In web development, Django and Flask frameworks simplify the development process. 2) In the fields of data science and machine learning, NumPy, Pandas, Scikit-learn and TensorFlow libraries provide strong support. 3) In terms of automation and scripting, Python is suitable for tasks such as automated testing and system management.
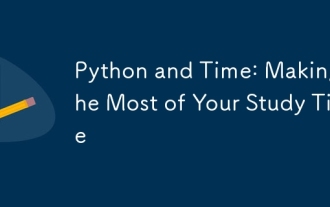
To maximize the efficiency of learning Python in a limited time, you can use Python's datetime, time, and schedule modules. 1. The datetime module is used to record and plan learning time. 2. The time module helps to set study and rest time. 3. The schedule module automatically arranges weekly learning tasks.
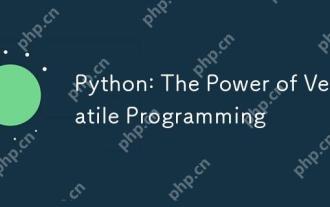
Python is highly favored for its simplicity and power, suitable for all needs from beginners to advanced developers. Its versatility is reflected in: 1) Easy to learn and use, simple syntax; 2) Rich libraries and frameworks, such as NumPy, Pandas, etc.; 3) Cross-platform support, which can be run on a variety of operating systems; 4) Suitable for scripting and automation tasks to improve work efficiency.
