


Mastering Closures and Decorators in Python: From Basics to Advanced
Introduction
Closures and decorators are powerful features in Python that allow you to write more flexible and reusable code. Understanding these concepts will take your Python skills to the next level, allowing you to handle more complex scenarios like logging, access control, and memoization with ease.
In this blog post, we'll explore:
- What are closures?
- Understanding how closures work in Python
- Use cases for closures
- What are decorators?
- Understanding how decorators work
- Using built-in decorators
- Writing custom decorators
- Advanced concepts with decorators
By the end of this article, you'll have a solid grasp of closures and decorators, and you'll be able to apply them effectively in your own code.
What Are Closures?
In Python, closures are functions that retain the values of variables from their enclosing lexical scope even when the outer function has finished executing. Closures are a way to retain state between function calls, which makes them useful for scenarios where you need to maintain some context.
Closures consist of three main components:
- A nested function
- A reference to a free variable in the enclosing function
- The enclosing function has finished executing, but the nested function still remembers the state of the free variable.
Basic Example of a Closure
Here’s an example of a simple closure:
def outer_function(message): def inner_function(): print(message) return inner_function # Create a closure closure = outer_function("Hello, World!") closure() # Output: Hello, World!
In this example, inner_function references the message variable from outer_function, even after outer_function has finished executing. The inner function "closes over" the variable from the outer scope, hence the term closure.
How Closures Work Internally
Closures work by capturing the state of free variables and storing them in the function object’s __closure__ attribute.
Let’s inspect the closure from the previous example:
print(closure.__closure__[0].cell_contents) # Output: Hello, World!
The __closure__ attribute holds the references to the variables that the closure retains. Each variable is stored in a "cell," and you can access its contents with cell_contents.
Use Cases for Closures
Closures are especially useful when you want to maintain state between function calls without using global variables or classes. Here are some common use cases:
1. Function Factories
You can use closures to create functions dynamically.
def multiplier(factor): def multiply_by_factor(number): return number * factor return multiply_by_factor times_two = multiplier(2) times_three = multiplier(3) print(times_two(5)) # Output: 10 print(times_three(5)) # Output: 15
In this example, multiplier returns a function that multiplies a given number by a specific factor. The closures times_two and times_three retain the value of the factor from their enclosing scope.
2. Encapsulation
Closures allow you to encapsulate behavior without exposing the internal state. This is similar to the concept of private methods in object-oriented programming.
def counter(): count = 0 def increment(): nonlocal count count += 1 return count return increment counter_fn = counter() print(counter_fn()) # Output: 1 print(counter_fn()) # Output: 2
In this example, the count variable is encapsulated within the closure, and only the increment function can modify its value.
What Are Decorators?
A decorator is a function that takes another function and extends or alters its behavior without modifying the original function's code. Decorators are often used to add functionality such as logging, access control, or timing to functions and methods.
In Python, decorators are applied to functions using the @ symbol above the function definition.
Basic Example of a Decorator
def decorator_function(original_function): def wrapper_function(): print(f"Wrapper executed before {original_function.__name__}()") return original_function() return wrapper_function @decorator_function def say_hello(): print("Hello!") say_hello() # Output: # Wrapper executed before say_hello() # Hello!
Here, decorator_function is applied to say_hello, adding extra functionality before say_hello() executes.
How Decorators Work
Decorators are essentially syntactic sugar for a common pattern in Python: higher-order functions, which take other functions as arguments. When you write @decorator, it’s equivalent to:
say_hello = decorator_function(say_hello)
The decorator function returns a new function (wrapper_function), which extends the behavior of the original function.
Decorators with Arguments
If the function being decorated takes arguments, the wrapper function needs to accept *args and **kwargs to pass the arguments along.
def decorator_function(original_function): def wrapper_function(*args, **kwargs): print(f"Wrapper executed before {original_function.__name__}()") return original_function(*args, **kwargs) return wrapper_function @decorator_function def display_info(name, age): print(f"display_info ran with arguments ({name}, {age})") display_info("John", 25) # Output: # Wrapper executed before display_info() # display_info ran with arguments (John, 25)
Built-In Decorators in Python
Python provides several built-in decorators, such as @staticmethod, @classmethod, and @property.
@staticmethod and @classmethod
These decorators are commonly used in object-oriented programming to define methods that are either not bound to the instance (@staticmethod) or bound to the class itself (@classmethod).
class MyClass: @staticmethod def static_method(): print("Static method called") @classmethod def class_method(cls): print(f"Class method called from {cls}") MyClass.static_method() # Output: Static method called MyClass.class_method() # Output: Class method called from <class '__main__.MyClass'>
@property
The @property decorator allows you to define a method that can be accessed like an attribute.
class Circle: def __init__(self, radius): self._radius = radius @property def radius(self): return self._radius @radius.setter def radius(self, value): if value <= 0: raise ValueError("Radius must be positive") self._radius = value c = Circle(5) print(c.radius) # Output: 5 c.radius = 10 print(c.radius) # Output: 10
Writing Custom Decorators
You can write your own decorators to add custom functionality to your functions or methods. Decorators can be stacked, meaning you can apply multiple decorators to a single function.
Example: Timing a Function
Here’s a custom decorator that measures the execution time of a function:
import time def timer_decorator(func): def wrapper(*args, **kwargs): start_time = time.time() result = func(*args, **kwargs) end_time = time.time() print(f"{func.__name__} ran in {end_time - start_time:.4f} seconds") return result return wrapper @timer_decorator def calculate_square(numbers): result = [n * n for n in numbers] return result nums = range(1, 1000000) calculate_square(nums)
Decorators with Arguments
Decorators can also accept their own arguments. This is useful when you need to pass configuration values to the decorator.
Example: Logger with Custom Message
def logger_decorator(message): def decorator(func): def wrapper(*args, **kwargs): print(f"{message}: Executing {func.__name__}") return func(*args, **kwargs) return wrapper return decorator @logger_decorator("DEBUG") def greet(name): print(f"Hello, {name}!") greet("Alice") # Output: # DEBUG: Executing greet # Hello, Alice!
In this example, the decorator logger_decorator takes a message as an argument, and then it wraps the greet function with additional logging functionality.
Advanced Decorator Concepts
1. Decorating Classes
Decorators can be applied not only to functions but also to classes. Class decorators modify or extend the behavior of entire classes.
def add_str_repr(cls): cls.__str__ = lambda self: f"Instance of {cls.__name__}" return cls @add_str_repr class Dog: pass dog = Dog() print(dog) # Output: Instance of Dog
2. Memoization with Decorators
Memoization is an optimization technique where the results of expensive function calls are cached, so subsequent calls with the same arguments can be returned faster.
def memoize(func): cache = {} def wrapper(*args): if args not in cache: cache[args] = func(*args) return cache[args] return wrapper @memoize def fibonacci(n): if n in [0, 1]: return n return fibonacci(n - 1) + fibonacci(n - 2) print(fibonacci(30)) # Output: 832040
Conclusion
Closures and decorators are advanced Python concepts that unlock powerful capabilities for writing cleaner, more efficient code. Closures allow you to maintain state and encapsulate data, while decorators let you modify or extend the behavior of functions and methods in a reusable way. Whether you're optimizing performance with memoization, implementing access control, or adding logging, decorators are an essential tool in your Python toolkit.
By mastering these concepts, you'll be able to write more concise and maintainable code and handle complex programming tasks with ease.
Feel free to experiment with closures and decorators in your projects and discover how they can make your code more elegant and powerful!
Connect with Me
- GitHub
The above is the detailed content of Mastering Closures and Decorators in Python: From Basics to Advanced. For more information, please follow other related articles on the PHP Chinese website!

Hot AI Tools

Undresser.AI Undress
AI-powered app for creating realistic nude photos

AI Clothes Remover
Online AI tool for removing clothes from photos.

Undress AI Tool
Undress images for free

Clothoff.io
AI clothes remover

Video Face Swap
Swap faces in any video effortlessly with our completely free AI face swap tool!

Hot Article

Hot Tools

Notepad++7.3.1
Easy-to-use and free code editor

SublimeText3 Chinese version
Chinese version, very easy to use

Zend Studio 13.0.1
Powerful PHP integrated development environment

Dreamweaver CS6
Visual web development tools

SublimeText3 Mac version
God-level code editing software (SublimeText3)

Hot Topics










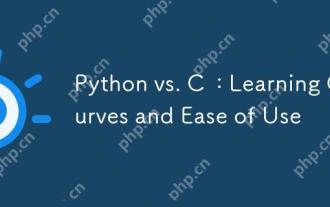
Python is easier to learn and use, while C is more powerful but complex. 1. Python syntax is concise and suitable for beginners. Dynamic typing and automatic memory management make it easy to use, but may cause runtime errors. 2.C provides low-level control and advanced features, suitable for high-performance applications, but has a high learning threshold and requires manual memory and type safety management.
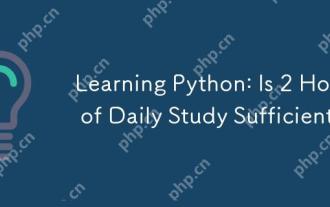
Is it enough to learn Python for two hours a day? It depends on your goals and learning methods. 1) Develop a clear learning plan, 2) Select appropriate learning resources and methods, 3) Practice and review and consolidate hands-on practice and review and consolidate, and you can gradually master the basic knowledge and advanced functions of Python during this period.
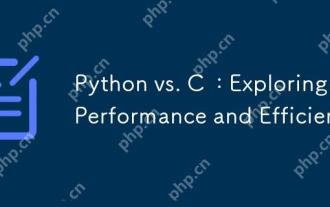
Python is better than C in development efficiency, but C is higher in execution performance. 1. Python's concise syntax and rich libraries improve development efficiency. 2.C's compilation-type characteristics and hardware control improve execution performance. When making a choice, you need to weigh the development speed and execution efficiency based on project needs.
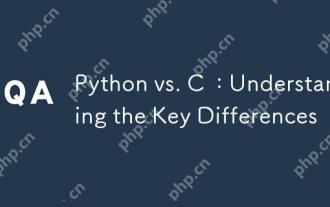
Python and C each have their own advantages, and the choice should be based on project requirements. 1) Python is suitable for rapid development and data processing due to its concise syntax and dynamic typing. 2)C is suitable for high performance and system programming due to its static typing and manual memory management.
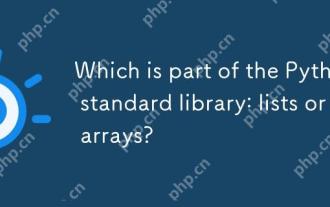
Pythonlistsarepartofthestandardlibrary,whilearraysarenot.Listsarebuilt-in,versatile,andusedforstoringcollections,whereasarraysareprovidedbythearraymoduleandlesscommonlyusedduetolimitedfunctionality.
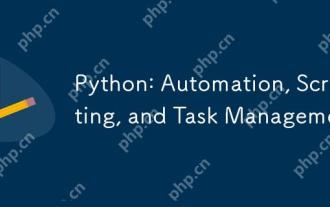
Python excels in automation, scripting, and task management. 1) Automation: File backup is realized through standard libraries such as os and shutil. 2) Script writing: Use the psutil library to monitor system resources. 3) Task management: Use the schedule library to schedule tasks. Python's ease of use and rich library support makes it the preferred tool in these areas.
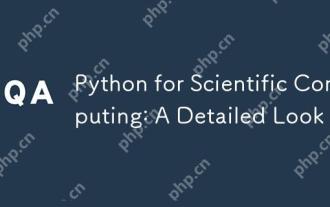
Python's applications in scientific computing include data analysis, machine learning, numerical simulation and visualization. 1.Numpy provides efficient multi-dimensional arrays and mathematical functions. 2. SciPy extends Numpy functionality and provides optimization and linear algebra tools. 3. Pandas is used for data processing and analysis. 4.Matplotlib is used to generate various graphs and visual results.
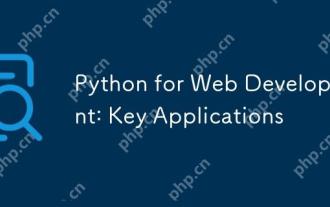
Key applications of Python in web development include the use of Django and Flask frameworks, API development, data analysis and visualization, machine learning and AI, and performance optimization. 1. Django and Flask framework: Django is suitable for rapid development of complex applications, and Flask is suitable for small or highly customized projects. 2. API development: Use Flask or DjangoRESTFramework to build RESTfulAPI. 3. Data analysis and visualization: Use Python to process data and display it through the web interface. 4. Machine Learning and AI: Python is used to build intelligent web applications. 5. Performance optimization: optimized through asynchronous programming, caching and code
