From legacy to cloud serverless - Part 1
Note: This article was originally published on Nov 4, 2023 here. It has been republished here to reach a broader audience.
Welcome to the first article in a series that will walk you through the process of migrating a legacy app from on-premises to the cloud, with a focus on modernization, serverless platforms, and integrated DevOps practices.
In this article, we will focus on containerizing your app. However, if you're building an app from scratch, that's perfectly fine (in fact, it's even better). For this example, I'm using this DigitalOcean guide to build a simple TODO app using Python (Flask) and MongoDB as the database. I've made some customizations to make it look better, but the main point is to build something that uses a NoSQL document-based database, as this will be required for the upcoming work.
You can clone the repository of the app here on GitHub if you haven't built your own.
Once you have your app built, let's get started!
Dockerfile
Here is the structure of the application directory that we will containerize, followed by the Dockerfile.
. ├── app.py ├── LICENSE ├── README.md ├── requirements.txt ├── static │ └── style.css └── templates └── index.html
The app.py file is the main application file that contains the Flask app code. The requirements.txt file contains the list of Python dependencies required by the application. The static/ directory contains static files such as CSS, JavaScript, and images. The templates/ directory contains the HTML templates used by the Flask app.
# Use a minimal base image FROM python:3.9.7-slim-buster AS base # Create a non-root user RUN useradd -m -s /bin/bash flaskuser USER flaskuser # Set the working directory WORKDIR /app # Copy the requirements file and install dependencies COPY requirements.txt . RUN pip install --no-cache-dir -r requirements.txt # Add the directory containing the flask command to the PATH ENV PATH="/home/flaskuser/.local/bin:${PATH}" # Use a multi-stage build to minimize the size of the image FROM base AS final # Copy the app code COPY app.py . COPY templates templates/ COPY static static/ # Set environment variables ENV FLASK_APP=app.py ENV FLASK_ENV=production # Expose the port EXPOSE 5000 # Run the app CMD ["flask", "run", "--host=0.0.0.0"]
Here's a walkthrough and breakdown of the Dockerfile:
The Dockerfile starts with a FROM instruction that specifies the base image to use. In this case, it's python:3.9.7-slim-buster, which is a minimal base image that includes Python 3.9.7 and some essential libraries.
The next instruction creates a non-root user named flaskuser using the RUN and useradd commands. This is a security best practice to avoid running the container as the root user.
The WORKDIR instruction sets the working directory to /app, which is where the application code will be copied.
The COPY instruction copies the requirements.txt file to the container's /app directory.
The RUN instruction installs the dependencies listed in requirements.txt using pip. The --no-cache-dir option is used to avoid caching the downloaded packages, which helps to keep the image size small.
The ENV instruction adds the directory containing the flask command to the PATH environment variable. This is necessary to run the flask command later.
The FROM instruction starts a new build stage using the base image defined earlier. This is a multi-stage build that helps to minimize the size of the final image.
The COPY instruction copies the application code (app.py), templates (templates/), and static files (static/) to the container's /app directory.
The ENV instruction sets the FLASK_APP and FLASK_ENV environment variables. FLASK_APP specifies the name of the main application file, and FLASK_ENV sets the environment to production.
The EXPOSE instruction exposes port 5000, which is the default port used by Flask.
The CMD instruction specifies the command to run when the container starts. In this case, it runs the flask run command with the --host=0.0.0.0 option to bind to all network interfaces.
With this Dockerfile, the application can be containerized and executed. However, it's important to note that our app requires a database to store the data created or generated while it's running. Of course, you could separately pull a MongoDB database image and run it independently. Then, make adjustments on both sides to establish communication between the two containers so that the app can successfully store data in the database. While this approach works, it may consume time and be a bit tedious. To streamline the process, we will instead move forward with Docker Compose. In Docker Compose, everything is declared in a YAML file, and by using the docker-compose up command, we can start and operate the different services seamlessly, saving time and effort.
Streamlining Database Integration with Docker Compose
Here is the basic Docker Compose YAML file that we will use to streamline the process.
version: '3.9' services: db: image: mongo:4.4.14 ports: - "27017:27017" volumes: - mongo-data:/data/db web: build: . container_name: "myflaskapp" ports: - "5000:5000" environment: - MONGO_URI=mongodb://db:27017 depends_on: - db volumes: mongo-data:
This Docker Compose YAML file is configured to set up two services: a MongoDB database (db) and a web application (web). Here's a breakdown:
Version: Specifies the version of the Docker Compose file format being used (3.9 in this case).
-
Services:
-
Database (db):
- MongoDB バージョン 4.4.14 イメージを使用します。
- ホスト ポート 27017 をコンテナ ポート 27017 にマッピングします。
- mongo-data という名前のボリュームを利用して、MongoDB データを永続的に保存します。
-
Web アプリケーション (Web):
- 現在のディレクトリ (.) から Docker イメージを構築します。
- コンテナ名を「myflaskapp」に設定します。
- ホスト ポート 5000 をコンテナ ポート 5000 にマッピングします。
- 環境変数 MONGO_URI を値 mongodb://db:27017 で定義し、MongoDB サービスへの接続を確立します。
- DB サービスへの依存関係を指定し、データベースが Web サービスより前に開始されるようにします。
-
-
ボリューム:
- MongoDB データを永続化するために、mongo-data という名前のボリュームを定義します。
要約すると、この Docker Compose ファイルは MongoDB データベースと Flask Web アプリケーションのデプロイメントを調整し、それらがシームレスに通信して連携できるようにします。
次に、Docker Compose ファイルのあるディレクトリに移動し、docker-compose up を実行して MongoDB と Flask Web アプリを起動します。 http://localhost:5000 でアプリにアクセスし、すべてが期待どおりに動作することを確認します。
停止するには、docker-compose down を使用します。
大丈夫ですか?次は、次の記事でワークフローを Kubernetes に移行します。
The above is the detailed content of From legacy to cloud serverless - Part 1. For more information, please follow other related articles on the PHP Chinese website!

Hot AI Tools

Undresser.AI Undress
AI-powered app for creating realistic nude photos

AI Clothes Remover
Online AI tool for removing clothes from photos.

Undress AI Tool
Undress images for free

Clothoff.io
AI clothes remover

Video Face Swap
Swap faces in any video effortlessly with our completely free AI face swap tool!

Hot Article

Hot Tools

Notepad++7.3.1
Easy-to-use and free code editor

SublimeText3 Chinese version
Chinese version, very easy to use

Zend Studio 13.0.1
Powerful PHP integrated development environment

Dreamweaver CS6
Visual web development tools

SublimeText3 Mac version
God-level code editing software (SublimeText3)

Hot Topics










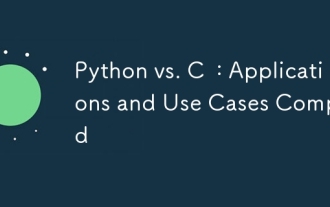
Python is suitable for data science, web development and automation tasks, while C is suitable for system programming, game development and embedded systems. Python is known for its simplicity and powerful ecosystem, while C is known for its high performance and underlying control capabilities.
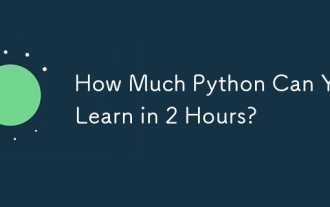
You can learn the basics of Python within two hours. 1. Learn variables and data types, 2. Master control structures such as if statements and loops, 3. Understand the definition and use of functions. These will help you start writing simple Python programs.
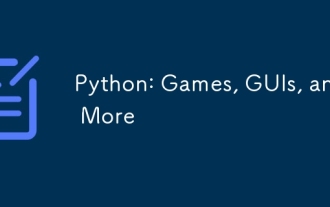
Python excels in gaming and GUI development. 1) Game development uses Pygame, providing drawing, audio and other functions, which are suitable for creating 2D games. 2) GUI development can choose Tkinter or PyQt. Tkinter is simple and easy to use, PyQt has rich functions and is suitable for professional development.
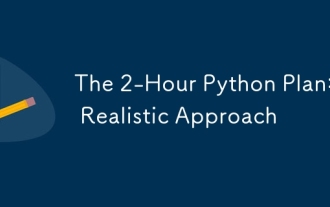
You can learn basic programming concepts and skills of Python within 2 hours. 1. Learn variables and data types, 2. Master control flow (conditional statements and loops), 3. Understand the definition and use of functions, 4. Quickly get started with Python programming through simple examples and code snippets.
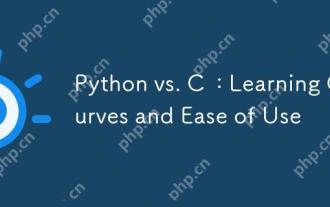
Python is easier to learn and use, while C is more powerful but complex. 1. Python syntax is concise and suitable for beginners. Dynamic typing and automatic memory management make it easy to use, but may cause runtime errors. 2.C provides low-level control and advanced features, suitable for high-performance applications, but has a high learning threshold and requires manual memory and type safety management.
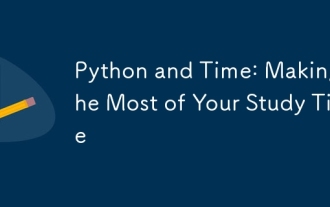
To maximize the efficiency of learning Python in a limited time, you can use Python's datetime, time, and schedule modules. 1. The datetime module is used to record and plan learning time. 2. The time module helps to set study and rest time. 3. The schedule module automatically arranges weekly learning tasks.
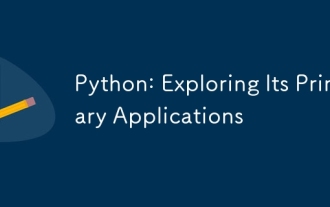
Python is widely used in the fields of web development, data science, machine learning, automation and scripting. 1) In web development, Django and Flask frameworks simplify the development process. 2) In the fields of data science and machine learning, NumPy, Pandas, Scikit-learn and TensorFlow libraries provide strong support. 3) In terms of automation and scripting, Python is suitable for tasks such as automated testing and system management.
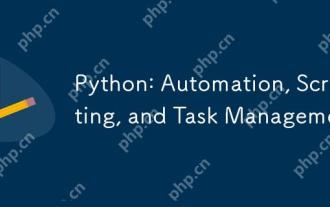
Python excels in automation, scripting, and task management. 1) Automation: File backup is realized through standard libraries such as os and shutil. 2) Script writing: Use the psutil library to monitor system resources. 3) Task management: Use the schedule library to schedule tasks. Python's ease of use and rich library support makes it the preferred tool in these areas.
