Tensorflow music prediction
In this article, I show how to use tensorflow to predict a style of music.
In my example, I compare techno and classical music.
You can find the code on my github :
https://github.com/victordalet/sound_to_partition
I - Dataset
For the first step, you need to create one dataset forlder and inside add one folder for music style, for example i add one techno folder and classic folder in which in put my wav soung.
II - Train
I create a train file, with the arguments max_epochs to be completed.
Modify the classes in the constructor that correspond to your directory in the dataset folder.
In the loading and processing method, I retrieve the wav file from a different directory and obtain the spectogram.
For training purposes, I use the Keras convolutions and model.
import os import sys from typing import List import librosa import numpy as np from tensorflow.keras.layers import Input, Conv2D, MaxPooling2D, Flatten, Dense from tensorflow.keras.models import Model from tensorflow.keras.optimizers import Adam from sklearn.model_selection import train_test_split from tensorflow.keras.utils import to_categorical from tensorflow.image import resize class Train: def __init__(self): self.X_train = None self.X_test = None self.y_train = None self.y_test = None self.data_dir: str = 'dataset' self.classes: List[str] = ['techno','classic'] self.max_epochs: int = int(sys.argv[1]) @staticmethod def load_and_preprocess_data(data_dir, classes, target_shape=(128, 128)): data = [] labels = [] for i, class_name in enumerate(classes): class_dir = os.path.join(data_dir, class_name) for filename in os.listdir(class_dir): if filename.endswith('.wav'): file_path = os.path.join(class_dir, filename) audio_data, sample_rate = librosa.load(file_path, sr=None) mel_spectrogram = librosa.feature.melspectrogram(y=audio_data, sr=sample_rate) mel_spectrogram = resize(np.expand_dims(mel_spectrogram, axis=-1), target_shape) data.append(mel_spectrogram) labels.append(i) return np.array(data), np.array(labels) def create_model(self): data, labels = self.load_and_preprocess_data(self.data_dir, self.classes) labels = to_categorical(labels, num_classes=len(self.classes)) # Convert labels to one-hot encoding self.X_train, self.X_test, self.y_train, self.y_test = train_test_split(data, labels, test_size=0.2, random_state=42) input_shape = self.X_train[0].shape input_layer = Input(shape=input_shape) x = Conv2D(32, (3, 3), activation='relu')(input_layer) x = MaxPooling2D((2, 2))(x) x = Conv2D(64, (3, 3), activation='relu')(x) x = MaxPooling2D((2, 2))(x) x = Flatten()(x) x = Dense(64, activation='relu')(x) output_layer = Dense(len(self.classes), activation='softmax')(x) self.model = Model(input_layer, output_layer) self.model.compile(optimizer=Adam(learning_rate=0.001), loss='categorical_crossentropy', metrics=['accuracy']) def train_model(self): self.model.fit(self.X_train, self.y_train, epochs=self.max_epochs, batch_size=32, validation_data=(self.X_test, self.y_test)) test_accuracy = self.model.evaluate(self.X_test, self.y_test, verbose=0) print(test_accuracy[1]) def save_model(self): self.model.save('weight.h5') if __name__ == '__main__': train = Train() train.create_model() train.train_model() train.save_model()
III - Test
To test and use the model, I've created this class to retrieve the weight and predict the style of the music.
Don't forget to add the right classes to the constructor.
from typing import List import librosa import numpy as np from tensorflow.keras.models import load_model from tensorflow.image import resize import tensorflow as tf class Test: def __init__(self, audio_file_path: str): self.model = load_model('weight.h5') self.target_shape = (128, 128) self.classes: List[str] = ['techno','classic'] self.audio_file_path: str = audio_file_path def test_audio(self, file_path, model): audio_data, sample_rate = librosa.load(file_path, sr=None) mel_spectrogram = librosa.feature.melspectrogram(y=audio_data, sr=sample_rate) mel_spectrogram = resize(np.expand_dims(mel_spectrogram, axis=-1), self.target_shape) mel_spectrogram = tf.reshape(mel_spectrogram, (1,) + self.target_shape + (1,)) predictions = model.predict(mel_spectrogram) class_probabilities = predictions[0] predicted_class_index = np.argmax(class_probabilities) return class_probabilities, predicted_class_index def test(self): class_probabilities, predicted_class_index = self.test_audio(self.audio_file_path, self.model) for i, class_label in enumerate(self.classes): probability = class_probabilities[i] print(f'Class: {class_label}, Probability: {probability:.4f}') predicted_class = self.classes[predicted_class_index] accuracy = class_probabilities[predicted_class_index] print(f'The audio is classified as: {predicted_class}') print(f'Accuracy: {accuracy:.4f}')
The above is the detailed content of Tensorflow music prediction. For more information, please follow other related articles on the PHP Chinese website!

Hot AI Tools

Undresser.AI Undress
AI-powered app for creating realistic nude photos

AI Clothes Remover
Online AI tool for removing clothes from photos.

Undress AI Tool
Undress images for free

Clothoff.io
AI clothes remover

Video Face Swap
Swap faces in any video effortlessly with our completely free AI face swap tool!

Hot Article

Hot Tools

Notepad++7.3.1
Easy-to-use and free code editor

SublimeText3 Chinese version
Chinese version, very easy to use

Zend Studio 13.0.1
Powerful PHP integrated development environment

Dreamweaver CS6
Visual web development tools

SublimeText3 Mac version
God-level code editing software (SublimeText3)

Hot Topics










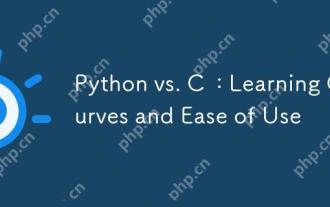
Python is easier to learn and use, while C is more powerful but complex. 1. Python syntax is concise and suitable for beginners. Dynamic typing and automatic memory management make it easy to use, but may cause runtime errors. 2.C provides low-level control and advanced features, suitable for high-performance applications, but has a high learning threshold and requires manual memory and type safety management.
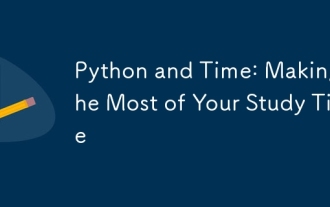
To maximize the efficiency of learning Python in a limited time, you can use Python's datetime, time, and schedule modules. 1. The datetime module is used to record and plan learning time. 2. The time module helps to set study and rest time. 3. The schedule module automatically arranges weekly learning tasks.
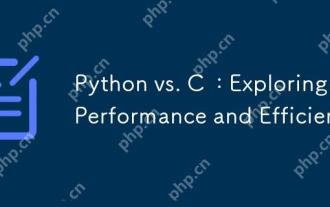
Python is better than C in development efficiency, but C is higher in execution performance. 1. Python's concise syntax and rich libraries improve development efficiency. 2.C's compilation-type characteristics and hardware control improve execution performance. When making a choice, you need to weigh the development speed and execution efficiency based on project needs.
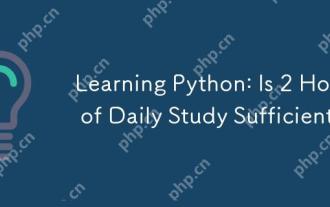
Is it enough to learn Python for two hours a day? It depends on your goals and learning methods. 1) Develop a clear learning plan, 2) Select appropriate learning resources and methods, 3) Practice and review and consolidate hands-on practice and review and consolidate, and you can gradually master the basic knowledge and advanced functions of Python during this period.
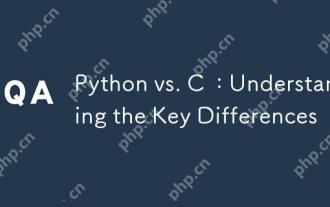
Python and C each have their own advantages, and the choice should be based on project requirements. 1) Python is suitable for rapid development and data processing due to its concise syntax and dynamic typing. 2)C is suitable for high performance and system programming due to its static typing and manual memory management.
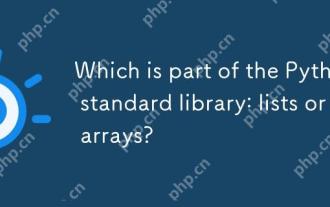
Pythonlistsarepartofthestandardlibrary,whilearraysarenot.Listsarebuilt-in,versatile,andusedforstoringcollections,whereasarraysareprovidedbythearraymoduleandlesscommonlyusedduetolimitedfunctionality.
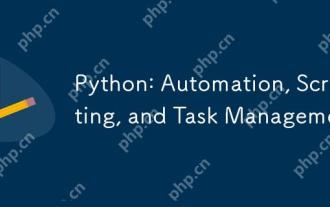
Python excels in automation, scripting, and task management. 1) Automation: File backup is realized through standard libraries such as os and shutil. 2) Script writing: Use the psutil library to monitor system resources. 3) Task management: Use the schedule library to schedule tasks. Python's ease of use and rich library support makes it the preferred tool in these areas.
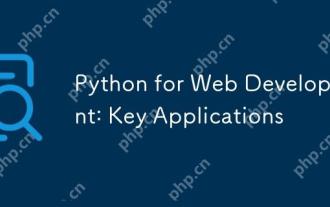
Key applications of Python in web development include the use of Django and Flask frameworks, API development, data analysis and visualization, machine learning and AI, and performance optimization. 1. Django and Flask framework: Django is suitable for rapid development of complex applications, and Flask is suitable for small or highly customized projects. 2. API development: Use Flask or DjangoRESTFramework to build RESTfulAPI. 3. Data analysis and visualization: Use Python to process data and display it through the web interface. 4. Machine Learning and AI: Python is used to build intelligent web applications. 5. Performance optimization: optimized through asynchronous programming, caching and code
