ROBOFLOW - train & test with python
Roboflow is a platform for annotating images for use in object detection AI.
I use this platform for C2SMR c2smr.fr, my computer vision association for maritime rescue.
In this article I show you how to use this platform and train your model with python.
You can find more sample code on my github : https://github.com/C2SMR/detector
I - Dataset
To create your dataset, go to https://app.roboflow.com/ and start annotating your image as shown in the following image.
In this example, I detour all the swimmers to predict their position in future images.
To get a good result, crop all the swimmers and place the bounding box just after the object to surround it correctly.
You can already use a public roboflow dataset, for this check https://universe.roboflow.com/
II - Training
For the training stage, you can use roboflow directly, but by the third time you'll have to pay, which is why I'm showing you how to do it with your laptop.
The first step is to import your dataset. To do this, you can import the Roboflow library.
pip install roboflow
To create a model, you need to use the YOLO algorithm, which you can import with the ultralytics library.
pip install ultralytics
In my script, I use the following command :
py train.py api-key project-workspace project-name project-version nb-epoch size_model
You must obtain :
- the access key
- workspace
- roboflow project name
- project dataset version
- number of epochs to train the model
- neural network size
Initially, the script downloads yolov8-obb.pt, the default yolo weight with pre-workout data, to facilitate training.
import sys import os import random from roboflow import Roboflow from ultralytics import YOLO import yaml import time class Main: rf: Roboflow project: object dataset: object model: object results: object model_size: str def __init__(self): self.model_size = sys.argv[6] self.import_dataset() self.train() def import_dataset(self): self.rf = Roboflow(api_key=sys.argv[1]) self.project = self.rf.workspace(sys.argv[2]).project(sys.argv[3]) self.dataset = self.project.version(sys.argv[4]).download("yolov8-obb") with open(f'{self.dataset.location}/data.yaml', 'r') as file: data = yaml.safe_load(file) data['path'] = self.dataset.location with open(f'{self.dataset.location}/data.yaml', 'w') as file: yaml.dump(data, file, sort_keys=False) def train(self): list_of_models = ["n", "s", "m", "l", "x"] if self.model_size != "ALL" and self.model_size in list_of_models: self.model = YOLO(f"yolov8{self.model_size}-obb.pt") self.results = self.model.train(data=f"{self.dataset.location}/" f"yolov8-obb.yaml", epochs=int(sys.argv[5]), imgsz=640) elif self.model_size == "ALL": for model_size in list_of_models: self.model = YOLO(f"yolov8{model_size}.pt") self.results = self.model.train(data=f"{self.dataset.location}" f"/yolov8-obb.yaml", epochs=int(sys.argv[5]), imgsz=640) else: print("Invalid model size") if __name__ == '__main__': Main()
III - Display
After training the model, you get the files best.py and last.py, which correspond to the weight.
With ultralytics library, you can also import YOLO and load your weight and then your test video.
In this example, I'm using the tracking function to get an ID for each swimmer.
import cv2 from ultralytics import YOLO import sys def main(): cap = cv2.VideoCapture(sys.argv[1]) model = YOLO(sys.argv[2]) while True: ret, frame = cap.read() results = model.track(frame, persist=True) res_plotted = results[0].plot() cv2.imshow("frame", res_plotted) if cv2.waitKey(1) == 27: break cap.release() cv2.destroyAllWindows() if __name__ == "__main__": main()
To analyze the prediction, you can obtain the model json as follows.
results = model.track(frame, persist=True) results_json = json.loads(results[0].tojson())
The above is the detailed content of ROBOFLOW - train & test with python. For more information, please follow other related articles on the PHP Chinese website!

Hot AI Tools

Undresser.AI Undress
AI-powered app for creating realistic nude photos

AI Clothes Remover
Online AI tool for removing clothes from photos.

Undress AI Tool
Undress images for free

Clothoff.io
AI clothes remover

Video Face Swap
Swap faces in any video effortlessly with our completely free AI face swap tool!

Hot Article

Hot Tools

Notepad++7.3.1
Easy-to-use and free code editor

SublimeText3 Chinese version
Chinese version, very easy to use

Zend Studio 13.0.1
Powerful PHP integrated development environment

Dreamweaver CS6
Visual web development tools

SublimeText3 Mac version
God-level code editing software (SublimeText3)

Hot Topics










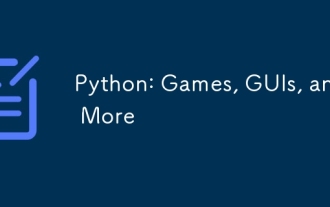
Python excels in gaming and GUI development. 1) Game development uses Pygame, providing drawing, audio and other functions, which are suitable for creating 2D games. 2) GUI development can choose Tkinter or PyQt. Tkinter is simple and easy to use, PyQt has rich functions and is suitable for professional development.
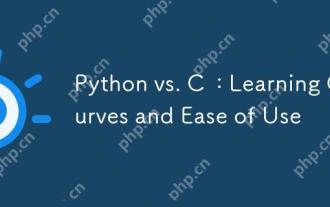
Python is easier to learn and use, while C is more powerful but complex. 1. Python syntax is concise and suitable for beginners. Dynamic typing and automatic memory management make it easy to use, but may cause runtime errors. 2.C provides low-level control and advanced features, suitable for high-performance applications, but has a high learning threshold and requires manual memory and type safety management.
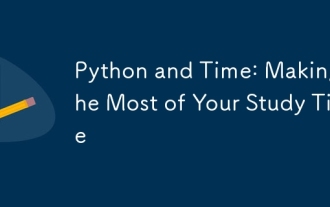
To maximize the efficiency of learning Python in a limited time, you can use Python's datetime, time, and schedule modules. 1. The datetime module is used to record and plan learning time. 2. The time module helps to set study and rest time. 3. The schedule module automatically arranges weekly learning tasks.
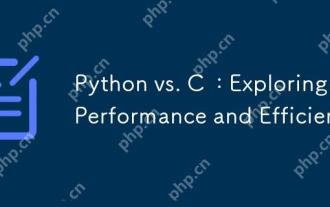
Python is better than C in development efficiency, but C is higher in execution performance. 1. Python's concise syntax and rich libraries improve development efficiency. 2.C's compilation-type characteristics and hardware control improve execution performance. When making a choice, you need to weigh the development speed and execution efficiency based on project needs.
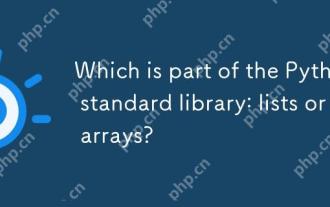
Pythonlistsarepartofthestandardlibrary,whilearraysarenot.Listsarebuilt-in,versatile,andusedforstoringcollections,whereasarraysareprovidedbythearraymoduleandlesscommonlyusedduetolimitedfunctionality.
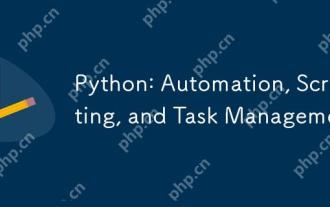
Python excels in automation, scripting, and task management. 1) Automation: File backup is realized through standard libraries such as os and shutil. 2) Script writing: Use the psutil library to monitor system resources. 3) Task management: Use the schedule library to schedule tasks. Python's ease of use and rich library support makes it the preferred tool in these areas.
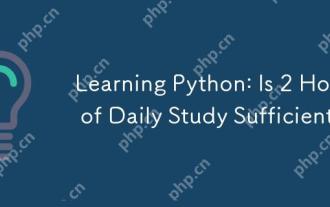
Is it enough to learn Python for two hours a day? It depends on your goals and learning methods. 1) Develop a clear learning plan, 2) Select appropriate learning resources and methods, 3) Practice and review and consolidate hands-on practice and review and consolidate, and you can gradually master the basic knowledge and advanced functions of Python during this period.
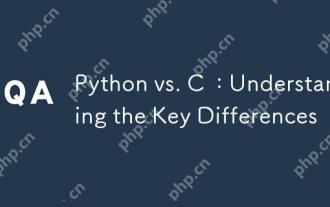
Python and C each have their own advantages, and the choice should be based on project requirements. 1) Python is suitable for rapid development and data processing due to its concise syntax and dynamic typing. 2)C is suitable for high performance and system programming due to its static typing and manual memory management.
