


Understanding Your Data: The Essentials of Exploratory Data Analysis (EDA)
Do you want to learn more about Exploratory Data Analysis? These are the fundamental concepts that you should be aware of.
Introduction to Exploratory Data Analysis
Understanding your dataset before beginning advanced analyses is not just wise, but necessary in the field of data science.
Exploratory Data Analysis is a data analytics procedure that seeks to fully comprehend the data and discover its many attributes, frequently through the use of visual aids. This enables the discovery of helpful patterns and a deeper understanding of the data.
It is imperative that you have a thorough understanding of it before you conduct data analysis and apply an algorithm to your data. Finding patterns in your data and identifying the variables that are crucial and those that don't affect the result are critical tasks. Moreover, there might be relationships between some variables and other ones. Errors in your data must also be identified.
Goals of Exploratory Data Analysis
Determining the distribution of variables in your data collection
Constructing a well-fitting model devoid of outliers to guarantee perfect data quality
Obtaining an accurate evaluation of the parameters
Estimating the estimations' uncertainty
Obtaining results that are statistically significant
Eliminating anomalies and superfluous numbers from the data
Aiding in the preparation of our dataset for examination
Allowing a machine learning model to more accurately predict our dataset
Providing results that are more accurate
Selecting a more effective machine learning model
Types of Exploratory Data Analysis
Descriptive Statistics
Summarizes the main features of a data set to provide a quick overview of the data using techniques such as Measures of central tendency (mean, median, mode). Measures of dispersion (range, variance, standard deviation). Frequency distributions.
Graphical Analysis
Uses visual tools to explore data to identify patterns, trends, and data anomalies through visualization using techniques such as Charts (bar charts, histograms, pie charts). Plots (scatter plots, line plots, box plots). Advanced visualizations (heatmaps, violin plots, pair plots).
Bivariate Analysis
In bivariate evaluation, the relationship between the variables is examined. Finding relationships, dependencies, and correlations between sets of variables is made possible by it. Some key techniques used in bivariate analysis:
- Scatter Plots
- Correlation Coefficient
- Cross-tabulation
- Line Graphs
- Covariance
Eploratory Data Analysis Tools
Data scientists can efficiently obtain deeper insights and prepare data for advanced analytics and modeling by utilizing the following tools for exploratory data analysis.
Python Libraries
Python Libraries
Pandas: Offers a wide range of functions for managing data structures and time series analysis, among other data manipulation and analysis tasks.
Matplotlib: A Python graphing package for making static, interactive, and animated charts.
Seaborn: It offers a high-level interface for creating eye-catching and educational statistical visuals and is built on top of Matplotlib.
Plotly: A graphing package that allows for interactive creation of plots and provides advanced visualization features.
Integrated Development Environments (IDEs)
Jupyter Notebook
RStudio
R Packages
ggplot2: It is a potent tool for creating intricate charts from data in a data frame and is a part of the tidyverse.
dplyr: A consistent set of verbs that will assist you in resolving the majority of data manipulation problems is known as the grammar of data manipulation.
tidyr: Aids in organizing your data.
Steps for Performing Exploratory Data Analysis
Step 1: Understand the Problem and the Data
Step 2: Import and Inspect the Data
Step 3: Handle Missing Data
Step 4: Explore Data Characteristics
Step 5: Perform Data Transformation
Step 6: Visualize Data Relationships
Step 7: Handling Outliers
Step 8: Communicate Findings and Insights
Final Thoughts
Exploratory Data Analysis is the cornerstone of data science efforts, providing priceless insights into the subtleties of datasets and clearing the way for well-informed decision-making.
EDA gives data scientists the ability to uncover hidden realities and guide initiatives toward success by exploring data distributions, correlations, and anomalies.
The above is the detailed content of Understanding Your Data: The Essentials of Exploratory Data Analysis (EDA). For more information, please follow other related articles on the PHP Chinese website!

Hot AI Tools

Undresser.AI Undress
AI-powered app for creating realistic nude photos

AI Clothes Remover
Online AI tool for removing clothes from photos.

Undress AI Tool
Undress images for free

Clothoff.io
AI clothes remover

Video Face Swap
Swap faces in any video effortlessly with our completely free AI face swap tool!

Hot Article

Hot Tools

Notepad++7.3.1
Easy-to-use and free code editor

SublimeText3 Chinese version
Chinese version, very easy to use

Zend Studio 13.0.1
Powerful PHP integrated development environment

Dreamweaver CS6
Visual web development tools

SublimeText3 Mac version
God-level code editing software (SublimeText3)

Hot Topics










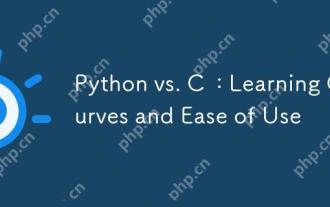
Python is easier to learn and use, while C is more powerful but complex. 1. Python syntax is concise and suitable for beginners. Dynamic typing and automatic memory management make it easy to use, but may cause runtime errors. 2.C provides low-level control and advanced features, suitable for high-performance applications, but has a high learning threshold and requires manual memory and type safety management.
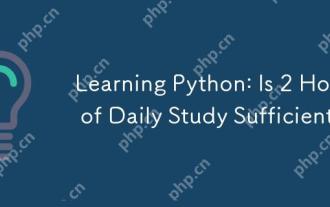
Is it enough to learn Python for two hours a day? It depends on your goals and learning methods. 1) Develop a clear learning plan, 2) Select appropriate learning resources and methods, 3) Practice and review and consolidate hands-on practice and review and consolidate, and you can gradually master the basic knowledge and advanced functions of Python during this period.
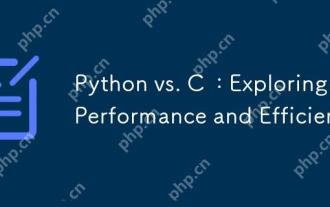
Python is better than C in development efficiency, but C is higher in execution performance. 1. Python's concise syntax and rich libraries improve development efficiency. 2.C's compilation-type characteristics and hardware control improve execution performance. When making a choice, you need to weigh the development speed and execution efficiency based on project needs.
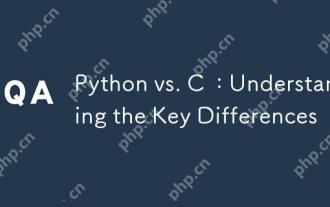
Python and C each have their own advantages, and the choice should be based on project requirements. 1) Python is suitable for rapid development and data processing due to its concise syntax and dynamic typing. 2)C is suitable for high performance and system programming due to its static typing and manual memory management.
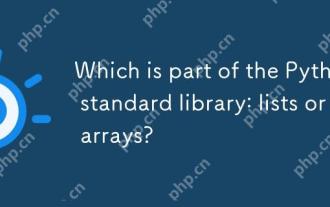
Pythonlistsarepartofthestandardlibrary,whilearraysarenot.Listsarebuilt-in,versatile,andusedforstoringcollections,whereasarraysareprovidedbythearraymoduleandlesscommonlyusedduetolimitedfunctionality.
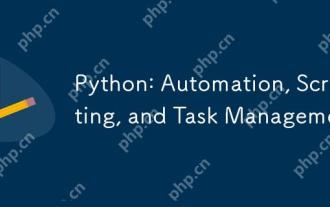
Python excels in automation, scripting, and task management. 1) Automation: File backup is realized through standard libraries such as os and shutil. 2) Script writing: Use the psutil library to monitor system resources. 3) Task management: Use the schedule library to schedule tasks. Python's ease of use and rich library support makes it the preferred tool in these areas.
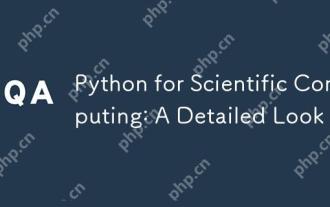
Python's applications in scientific computing include data analysis, machine learning, numerical simulation and visualization. 1.Numpy provides efficient multi-dimensional arrays and mathematical functions. 2. SciPy extends Numpy functionality and provides optimization and linear algebra tools. 3. Pandas is used for data processing and analysis. 4.Matplotlib is used to generate various graphs and visual results.
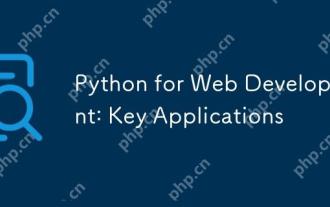
Key applications of Python in web development include the use of Django and Flask frameworks, API development, data analysis and visualization, machine learning and AI, and performance optimization. 1. Django and Flask framework: Django is suitable for rapid development of complex applications, and Flask is suitable for small or highly customized projects. 2. API development: Use Flask or DjangoRESTFramework to build RESTfulAPI. 3. Data analysis and visualization: Use Python to process data and display it through the web interface. 4. Machine Learning and AI: Python is used to build intelligent web applications. 5. Performance optimization: optimized through asynchronous programming, caching and code
