Production Readiness Checklist
I have been working on multiple pojects where I have moved applications from PoC to Production.
These are the checklists I have prepared for myself and my team to ensure we are ready for production.
Here the checklists are in focus as the application is in Python programming language and deployed to AWS via Kubernetes.
Not all of these are mandatory, but they are the ones I have found most useful.
1. Alerts & Metrics
- [ ] Are there alerts set up for infrastructure issues (e.g., memory or CPU usage increase, service unavailability)?
- [ ] Are there alerts set up for critical application-specific logic failures?
- [ ] Can we view historical data (past few hours/days) of infrastructure and resource usage?
- [ ] Is there a real-time monitoring dashboard in place?
2. Dashboard and SOP
- [ ] Is there an SOP document for handling alerts and known issues?
- [ ] Are there runbooks available for common scenarios?
- [ ] Is there an incident response plan in place?
3. On-call mapping and cadence
- [ ] Is there an on-call person mapping for application-level issues?
- [ ] Is there an on-call person mapping for infrastructure-related issues?
- [ ] Is there a defined rotation schedule and escalation policy?
4. Deployment
- [ ] Has the appropriate instance type (GPU or CPU) been determined?
- [ ] Has the required server type been specified?
- [ ] Is there multi-availability zone support for failover?
- [ ] Is there support for multiple regions?
- [ ] Is auto-scaling set up (e.g., HPA, Keda) for traffic spikes?
- [ ] Are health checks configured for the server?
- [ ] Have resource limits been defined and documented?
- [ ] Is there a blue-green or canary deployment strategy in place?
- [ ] Is there a defined rollback plan and procedure?
5. Observability and tracing
- [ ] Is there a dashboard showing relevant metrics (e.g., request count, HTTP status codes, usage)?
- [ ] Can we trace a single request end-to-end for debugging purposes?
- [ ] Is there a log aggregation and analysis system in place?
- [ ] Is distributed tracing implemented?
6. Load tests
- [ ] Has capacity planning been performed to determine the server's load handling capabilities?
- [ ] Are there defined performance benchmarks?
- [ ] Has stress testing been conducted?
7. Quality
- [ ] Are there automated unit tests?
- [ ] Are there automated integration tests?
- [ ] Is static code analysis (e.g., complexity checks) performed?
- [ ] Is code coverage measured and at an acceptable level?
- [ ] Are there production sanity test cases?
- [ ] Is there a CI/CD pipeline in place?
- [ ] Are security scans and vulnerability assessments performed regularly?
8. Release
- [ ] Is Swagger/OpenAPI documentation available and up-to-date?
- [ ] Is there a versioning system for APIs and releases?
- [ ] Is there an established communication channel for scheduled maintenance?
- [ ] Is there a change management process?
- [ ] Are feature flags used for gradual rollout of new features?
9. Disaster Recovery and Business Continuity
- [ ] Are backup and restore procedures in place and tested?
- [ ] Is there a data replication strategy?
- [ ] Have Recovery Time Objective (RTO) and Recovery Point Objective (RPO) been defined?
- [ ] Are regular disaster recovery drills conducted?
10. Compliance and Security
- [ ] Is data encrypted at rest and in transit?
- [ ] Are access control and authentication mechanisms in place?
- [ ] Are regular security audits conducted?
- [ ] Does the application comply with relevant industry standards (e.g., GDPR, HIPAA)?
11. Documentation
- [ ] Is system architecture documentation available and up-to-date?
- [ ] Is API documentation complete and current?
- [ ] Are operational procedures documented?
- [ ] Is there a comprehensive troubleshooting guide?
The above is the detailed content of Production Readiness Checklist. For more information, please follow other related articles on the PHP Chinese website!

Hot AI Tools

Undresser.AI Undress
AI-powered app for creating realistic nude photos

AI Clothes Remover
Online AI tool for removing clothes from photos.

Undress AI Tool
Undress images for free

Clothoff.io
AI clothes remover

Video Face Swap
Swap faces in any video effortlessly with our completely free AI face swap tool!

Hot Article

Hot Tools

Notepad++7.3.1
Easy-to-use and free code editor

SublimeText3 Chinese version
Chinese version, very easy to use

Zend Studio 13.0.1
Powerful PHP integrated development environment

Dreamweaver CS6
Visual web development tools

SublimeText3 Mac version
God-level code editing software (SublimeText3)

Hot Topics










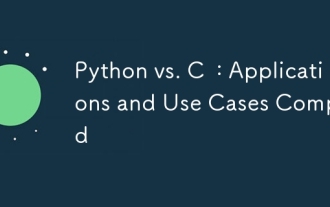
Python is suitable for data science, web development and automation tasks, while C is suitable for system programming, game development and embedded systems. Python is known for its simplicity and powerful ecosystem, while C is known for its high performance and underlying control capabilities.
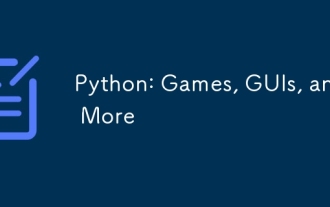
Python excels in gaming and GUI development. 1) Game development uses Pygame, providing drawing, audio and other functions, which are suitable for creating 2D games. 2) GUI development can choose Tkinter or PyQt. Tkinter is simple and easy to use, PyQt has rich functions and is suitable for professional development.
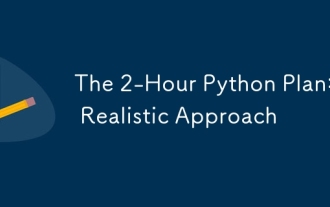
You can learn basic programming concepts and skills of Python within 2 hours. 1. Learn variables and data types, 2. Master control flow (conditional statements and loops), 3. Understand the definition and use of functions, 4. Quickly get started with Python programming through simple examples and code snippets.
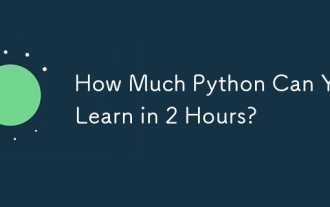
You can learn the basics of Python within two hours. 1. Learn variables and data types, 2. Master control structures such as if statements and loops, 3. Understand the definition and use of functions. These will help you start writing simple Python programs.
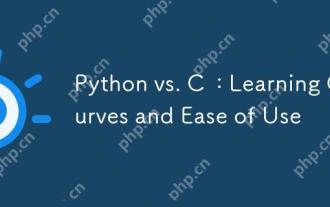
Python is easier to learn and use, while C is more powerful but complex. 1. Python syntax is concise and suitable for beginners. Dynamic typing and automatic memory management make it easy to use, but may cause runtime errors. 2.C provides low-level control and advanced features, suitable for high-performance applications, but has a high learning threshold and requires manual memory and type safety management.
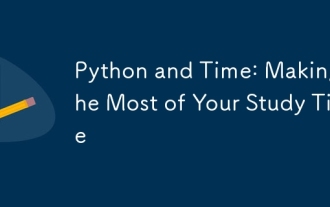
To maximize the efficiency of learning Python in a limited time, you can use Python's datetime, time, and schedule modules. 1. The datetime module is used to record and plan learning time. 2. The time module helps to set study and rest time. 3. The schedule module automatically arranges weekly learning tasks.
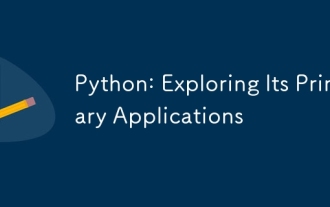
Python is widely used in the fields of web development, data science, machine learning, automation and scripting. 1) In web development, Django and Flask frameworks simplify the development process. 2) In the fields of data science and machine learning, NumPy, Pandas, Scikit-learn and TensorFlow libraries provide strong support. 3) In terms of automation and scripting, Python is suitable for tasks such as automated testing and system management.
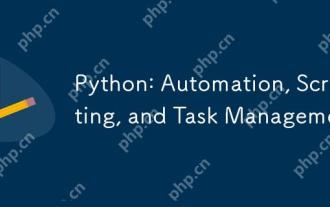
Python excels in automation, scripting, and task management. 1) Automation: File backup is realized through standard libraries such as os and shutil. 2) Script writing: Use the psutil library to monitor system resources. 3) Task management: Use the schedule library to schedule tasks. Python's ease of use and rich library support makes it the preferred tool in these areas.
