


Expert advice for building a successful career in data science: Education, skills and job search tips
Data science
It is one of the most dynamic and sought-after fields in the technology industry today. With the promise of solving complex problems and deriving actionable insights from data, it's no wonder that many are eager to join this exciting field. But how do you build a successful career in data science?
Here's expert advice on education, essential skills and effective job hunting tips to guide you on your way.
1. Lay a strong educational foundation
Math is key
A robust understanding of mathematics forms the foundation of data science. Here are the basic areas you should master:
- Linear Algebra: Essential for understanding data transformations and algorithms such as Singular Value Decomposition (SVD).
- Matrix Theory: Knowing how to manipulate matrices is essential for various machine learning algorithms.
- Calculus: Integral and differential calculus helps understand changes and trends in data.
- Statistics: Fundamentals of data analysis, covering distributions, hypothesis testing, and regression.
- Probability: A Key to Understanding Data Uncertainty and Basic Machine Learning Concepts.
Formal education
While self-study is valuable, formal education can provide a structured pathway:
- Bachelor's degree: In fields such as computer science, mathematics, statistics or engineering.
- Master's Degree: Consider advanced degrees in data science, computer science, or related fields to deepen your expertise.
- Online courses: Platforms like Coursera, edX, and Udacity offer specialized data science courses from top universities.
2. Develop core data science skills
Programming languages
Programming skills are non-negotiable in data science. Focus on:
- Python: The most popular language due to its simplicity and powerful libraries like NumPy, Pandas and Scikit-learn.
- R: Great for statistical analysis and data visualization.
Data manipulation and analysis
Learn how to clean, transform and analyze data using tools such as:
- Pandas: For manipulating data in Python.
- dplyr and tidyr: For data manipulation in R.
Data visualization
Data visualization helps understand and communicate insights:
- Matplotlib, Seaborn: Python visualization libraries.
- ggplot2: A visualization package in R.
- Tableau, Power BI: Tools for creating interactive visualizations.
Machine learning and AI
Understand and implement machine learning algorithms:
- Scikit-learn: For implementing machine learning algorithms in Python.
- TensorFlow, Keras: For deep learning projects.
- Natural Language Processing (NLP): Libraries like NLTK and spaCy for text analysis.
3. Practical projects and practical experiences
Create a portfolio
A portfolio showcases your skills and projects. Include:
- Data Cleaning Projects: Demonstrate your ability to preprocess and clean messy data.
- Exploratory Data Analysis (EDA): Show how you can derive insights from raw data.
- Machine Learning Models: Include projects where you implemented and tuned machine learning algorithms.
- Kaggle Challenges: Participate in Kaggle challenges to gain experience and visibility.
Internships and work experience
- Gaining practical experience through internships can be invaluable:
- Internships: Look for internships at tech companies, startups, or research institutions.
- Freelance projects: Offer your data science skills on platforms like Upwork or Fiverr.
4. Job hunting tips
networking
Networking can greatly boost your job search:
- Attending Meetups and Conferences: Engage with the data science community through events and conferences.
- LinkedIn: Connect with industry professionals and join relevant groups.
- Online communities: Participate in forums like Stack Overflow, Reddit (r/datascience), and GitHub.
Personalize your resume and cover letter
- Edit your resume and cover letter for each job application:
- Highlight relevant skills and projects: Focus on the skills and experience most relevant to the job description.
- Use keywords: Make sure your resume includes keywords from the job posting to get through the applicant tracking system (ATS).
Prepare for interviews
Data science interviews often include technical and behavioral components:
- Technical questions: Be prepared to answer questions about algorithms, data structures, and coding issues.
- Case Studies: Practice solving data science problems and presenting your findings.
- Soft skills: Demonstrate your ability to effectively communicate complex ideas and collaborate.
5. Continuous learning and adaptation
Data science is a constantly evolving field. Stay informed about the latest trends and technologies:
- Read Research Articles: Keep up with the latest advances by reading articles on Arxiv.
- Online Courses: Continuously enroll in courses to learn new tools and techniques.
- Stay curious: Never stop experimenting with new datasets, tools and algorithms
Conclusion
Building a successful career in data science requires a combination of strong educational foundations, practical skills, hands-on experience, and effective job search strategies. By following these expert tips and continuing to educate yourself, you can carve out a thriving career in this dynamic and rewarding field. Remember, consistency is key – every step you take brings you closer to becoming a proficient and sought-after data scientist.
The above is the detailed content of Expert advice for building a successful career in data science: Education, skills and job search tips. For more information, please follow other related articles on the PHP Chinese website!

Hot AI Tools

Undresser.AI Undress
AI-powered app for creating realistic nude photos

AI Clothes Remover
Online AI tool for removing clothes from photos.

Undress AI Tool
Undress images for free

Clothoff.io
AI clothes remover

Video Face Swap
Swap faces in any video effortlessly with our completely free AI face swap tool!

Hot Article

Hot Tools

Notepad++7.3.1
Easy-to-use and free code editor

SublimeText3 Chinese version
Chinese version, very easy to use

Zend Studio 13.0.1
Powerful PHP integrated development environment

Dreamweaver CS6
Visual web development tools

SublimeText3 Mac version
God-level code editing software (SublimeText3)

Hot Topics










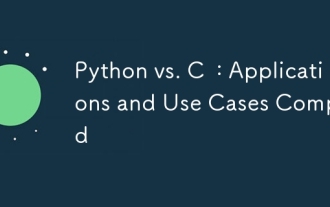
Python is suitable for data science, web development and automation tasks, while C is suitable for system programming, game development and embedded systems. Python is known for its simplicity and powerful ecosystem, while C is known for its high performance and underlying control capabilities.
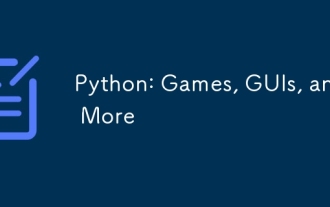
Python excels in gaming and GUI development. 1) Game development uses Pygame, providing drawing, audio and other functions, which are suitable for creating 2D games. 2) GUI development can choose Tkinter or PyQt. Tkinter is simple and easy to use, PyQt has rich functions and is suitable for professional development.
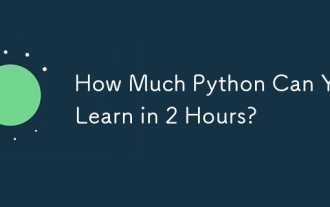
You can learn the basics of Python within two hours. 1. Learn variables and data types, 2. Master control structures such as if statements and loops, 3. Understand the definition and use of functions. These will help you start writing simple Python programs.
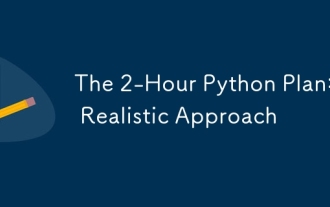
You can learn basic programming concepts and skills of Python within 2 hours. 1. Learn variables and data types, 2. Master control flow (conditional statements and loops), 3. Understand the definition and use of functions, 4. Quickly get started with Python programming through simple examples and code snippets.
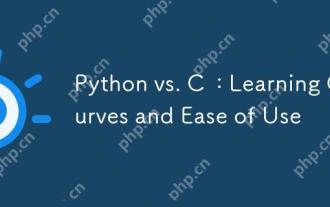
Python is easier to learn and use, while C is more powerful but complex. 1. Python syntax is concise and suitable for beginners. Dynamic typing and automatic memory management make it easy to use, but may cause runtime errors. 2.C provides low-level control and advanced features, suitable for high-performance applications, but has a high learning threshold and requires manual memory and type safety management.
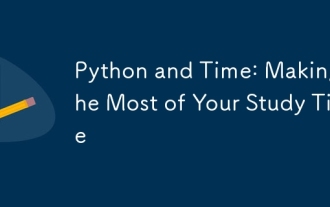
To maximize the efficiency of learning Python in a limited time, you can use Python's datetime, time, and schedule modules. 1. The datetime module is used to record and plan learning time. 2. The time module helps to set study and rest time. 3. The schedule module automatically arranges weekly learning tasks.
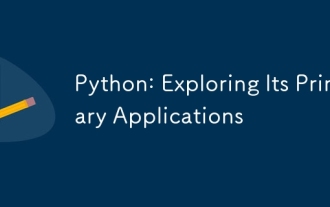
Python is widely used in the fields of web development, data science, machine learning, automation and scripting. 1) In web development, Django and Flask frameworks simplify the development process. 2) In the fields of data science and machine learning, NumPy, Pandas, Scikit-learn and TensorFlow libraries provide strong support. 3) In terms of automation and scripting, Python is suitable for tasks such as automated testing and system management.
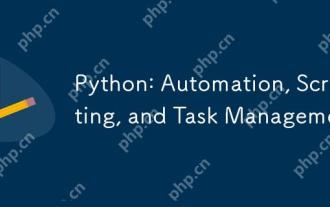
Python excels in automation, scripting, and task management. 1) Automation: File backup is realized through standard libraries such as os and shutil. 2) Script writing: Use the psutil library to monitor system resources. 3) Task management: Use the schedule library to schedule tasks. Python's ease of use and rich library support makes it the preferred tool in these areas.
