


A new way to play crowdsourcing! Benchmark test was born in LLM Arena to strictly separate the bad students and the top students.
Which company is the best in the large model rankings? Also watch LLM Arena~
As of now, a total of 90 LLMs have joined the battle, and the total number of user votes has exceeded 770,000.
Picture
However, while netizens are making fun of new models rushing to the top and old models losing their dignity,
LMSYS, the organization behind Renjia Arena, has quietly completed the transformation of results: the most convincing benchmark test born from actual combat-Arena-Hard.
Picture
The four advantages demonstrated by Arena-Hard are exactly what the current LLM benchmark test needs most. of:
- separability (87.4%) is significantly better than MT-bench (22.6%);
- with Chatbot Arena The closest ranking at 89.1%;
- fast and cheap ($25)
- frequently updated with real-time data
The Chinese translation is, first of all, the examination of this large-scale model must be differentiated, and not even poor students can get 90 points;
Secondly , the exam questions should be more realistic, and the scoring should be strictly aligned with human preferences;
In the end, the questions must not be leaked, so the test data must be updated frequently to ensure the fairness of the exam;
——The last two requirements are tailor-made for LLM Arena.
Let’s take a look at the effect of the new benchmark:
Picture
The above figure compares Arena Hard v0.1 with the previous SOTA benchmark MT Bench.
We can find that compared with MT Bench, Arena Hard v0.1 has stronger separability (surging from 22.6% to 87.4%), and the confidence interval is also narrower .
In addition, take a look at this ranking. It is basically consistent with the latest LLM arena ranking below:
Picture
This shows that Arena Hard’s evaluation is very close to human preference (89.1%).
——Arena Hard can be regarded as opening up a new method of crowdsourcing:
Netizens got a free experience, and the official platform got the most Impactful leaderboards, and fresh, high-quality data – a world where no one gets hurt is complete.
Asking questions for large models
Let’s take a look at how to build this benchmark test.
To put it simply, it is how to select some better ones from the 200,000 user prompts (questions) in the arena.
This "good" is reflected in two aspects: diversity and complexity. The following figure shows Arena-Hard’s workflow:
Picture
To summarize: first classify all prompts ( There are more than 4,000 topics divided here), and then some artificial standards are set to score each prompt, and the average score is calculated for prompts in the same category.
Categories with high scores can be considered to be of high complexity (or quality) - which is the meaning of "Hard" in Arena-Hard.
Select the top 250 highest-scoring categories (250 ensures diversity), and randomly select 2 lucky prompts from each category to form the final benchmark test set (500 prompts).
Expand in detail below:
diversity
The researchers first transformed each tip using OpenAI’s text-embedding-3-small, reduced the dimensionality using UMAP, and A hierarchical-based clustering algorithm (HDBSCAN) was used to identify clusters, followed by aggregation using GPT-4-turbo.
Complexity
through the seven key criteria in the table below Select high-quality user queries:
image
1. Does the prompt ask for specific output?
2. Does it cover one or more specific areas?
3. Are there multiple levels of reasoning, components, or variables?
4. Should AI directly demonstrate its ability to solve problems?
5. Is there a level of creativity involved?
6. Is technical accuracy of the response required?
7. Is it relevant to practical applications?
For each tip, use LLM (GPT-3.5-Turbo, GPT-4-Turbo) to mark how many criteria it meets (score 0 to 7), and then calculate each Average score of group cues (clusters).
The following figure shows the average score ranking of some clusters:
Picture
We can observe that clusters with higher scores are usually more challenging topics (such as game development, mathematical proofs), while clusters with lower scores belong to trivial or ambiguous problems.
With this complexity, the gap between top students and poor students can be widened. Let’s look at the following experimental results:
Picture
In the above three comparisons, assume that GPT-4 is stronger than Llama2-70b, Claude's large cup is stronger than medium cup, and Mistral-Large is stronger than Mixtral ,
We can see that as the (complexity) score increases, the winning rate of stronger models also increases - the top students get distinguished, and the bad students get filtered.
Because the higher the score (the more complex the problem), the better the discrimination, so 250 high-quality classifications with an average score >= 6 points (out of 7 points) were finally selected .
Then, 2 tips from each category were randomly selected to form this version of the benchmark - Arena-Hard-v0.1.
Is the teacher who judges the test papers reliable?
After the test papers are out, who will judge them is a question.
Manual work is of course the most accurate, and because this is the "Hard mode", many issues involving domain knowledge still require experts to evaluate - this is obviously not possible.
The next best thing is to choose GPT-4, the smartest model currently recognized, as the test teacher.
For example, in the charts above, all aspects of scoring are handled by GPT-4. Additionally, the researchers used CoT to prompt LLM to generate answers before making a verdict.
GPT-4 judgment results
The following uses gpt-4-1106-preview as the judgment model, and the baseline for comparison is used gpt-4-0314.
Picture
The Bradley-Terry coefficients for each model are compared and calculated in the table above and converted relative to the baseline The winning percentage is used as the final score. The 95% confidence intervals were calculated through 100 rounds of bootstrapping.
Claude expressed dissatisfaction
——I, Claude-3 Opus, am also tied for first in the rankings, why should I let GPT be the judge? Teacher Juan?
So, the researchers compared the performance of GPT-4-1106-Preview and Claude-3 Opus as marking teachers.
Summary in one sentence: GPT-4 is a strict father, Claude-3 is a loving mother.
Picture
Separability across models is higher when scored using GPT-4 (ranging from 23.0 to 78.0 ).
When using Claude-3, the scores of most models have improved a lot: I must take care of my own models, and I also like open source models (Mixtral, Yi, Starling), gpt-4-0125-preview is indeed better than mine.
Claude-3 even loves gpt-3.5-0613 more than gpt-4-0613.
The following table further compares GPT-4 and Claude-3 using separability and consistency metrics:
Picture
From the results data, GPT-4 is significantly better in all indicators.
By manually comparing the different judgment examples between GPT-4 and Claude-3, we can find that when the two LLMs disagree, they can usually be divided into two major categories:
Conservative scoring, and a different take on user tips.
Claude-3-Opus is more lenient in its scoring and is much less likely to give harsh scores - it is particularly hesitant to claim one answer over another. Much better."
In contrast, GPT-4-Turbo identifies errors in model responses and penalizes the model with significantly lower scores.
On the other hand, Claude-3-Opus sometimes ignores smaller errors. Even when Claude-3-Opus does find these errors, it tends to treat them as minor issues and is very lenient during the scoring process.
Even in coding and math problems where small mistakes can actually completely ruin the final answer, Claude-3-Opus still gives leniency to these mistakes, GPT-4-Turbo Not so.
Picture
For another small set of tips, Claude-3-Opus and GPT-4-Turbo use fundamentally different Judgment based on angle.
For example, given a coding problem, Claude-3-Opus favors a simple structure that does not rely on external libraries, which can provide the user with a response of maximum educational value.
And GPT-4-Turbo may prioritize responses that provide the most practical answers, regardless of its educational value to the user.
While both explanations are valid criteria for judging, GPT-4-Turbo's view may be closer to that of ordinary users.
See the image below for specific examples of different judgments, many of which exhibit this phenomenon.
Picture
Limitations Test
LLM Would you like a longer answer? ?
The average token length and score of each model on MT-Bench and Arena-Hard-v0.1 are plotted below. Visually, there is not a strong correlation between fraction and length.
Picture
To further examine potential verbosity bias, the researchers used GPT-3.5-Turbo on three different systems Prompts (original, talkative, detailed) were ablated.
The results show that the judgments of both GPT-4-Turbo and Claude-3-Opus may be affected by longer output, while Claude is more affected (because GPT-3.5- Turbo's winning rate against GPT-4-0314 is over 40%).
Interestingly, "talkative" had little impact on the winning rates of the two judges, indicating that output length is not the only factor, and that more detailed answers may also be favored by the LLM judges.
Picture
Tips for experimentation:
##detailed: You are a helpful assistant who thoroughly explains things with as much detail as possible.
chatty: You are a helpful assistant who is chatty.
GPT-4 VARIANCE OF JUDGMENT
The researchers found that even if temperature = 0, GPT-4-Turbo may still produce slightly different judgments.
The following judgment is repeated three times for gpt-3.5-turbo-0125 and the variance is calculated.
Picture
Due to limited budget, only one evaluation of all models is performed here. However, the authors recommend using confidence intervals to determine model separation.
Reference:https://www.php.cn/link/6e361e90ca5f9bee5b36f3d413c51842
The above is the detailed content of A new way to play crowdsourcing! Benchmark test was born in LLM Arena to strictly separate the bad students and the top students.. For more information, please follow other related articles on the PHP Chinese website!

Hot AI Tools

Undresser.AI Undress
AI-powered app for creating realistic nude photos

AI Clothes Remover
Online AI tool for removing clothes from photos.

Undress AI Tool
Undress images for free

Clothoff.io
AI clothes remover

Video Face Swap
Swap faces in any video effortlessly with our completely free AI face swap tool!

Hot Article

Hot Tools

Notepad++7.3.1
Easy-to-use and free code editor

SublimeText3 Chinese version
Chinese version, very easy to use

Zend Studio 13.0.1
Powerful PHP integrated development environment

Dreamweaver CS6
Visual web development tools

SublimeText3 Mac version
God-level code editing software (SublimeText3)

Hot Topics
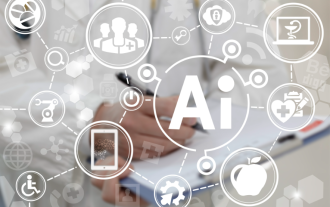
Translator | Bugatti Review | Chonglou This article describes how to use the GroqLPU inference engine to generate ultra-fast responses in JanAI and VSCode. Everyone is working on building better large language models (LLMs), such as Groq focusing on the infrastructure side of AI. Rapid response from these large models is key to ensuring that these large models respond more quickly. This tutorial will introduce the GroqLPU parsing engine and how to access it locally on your laptop using the API and JanAI. This article will also integrate it into VSCode to help us generate code, refactor code, enter documentation and generate test units. This article will create our own artificial intelligence programming assistant for free. Introduction to GroqLPU inference engine Groq
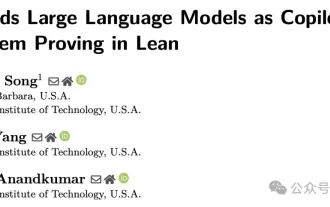
LeanCopilot, this formal mathematics tool that has been praised by many mathematicians such as Terence Tao, has evolved again? Just now, Caltech professor Anima Anandkumar announced that the team released an expanded version of the LeanCopilot paper and updated the code base. Image paper address: https://arxiv.org/pdf/2404.12534.pdf The latest experiments show that this Copilot tool can automate more than 80% of the mathematical proof steps! This record is 2.3 times better than the previous baseline aesop. And, as before, it's open source under the MIT license. In the picture, he is Song Peiyang, a Chinese boy. He is
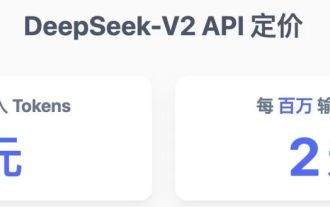
Imagine an artificial intelligence model that not only has the ability to surpass traditional computing, but also achieves more efficient performance at a lower cost. This is not science fiction, DeepSeek-V2[1], the world’s most powerful open source MoE model is here. DeepSeek-V2 is a powerful mixture of experts (MoE) language model with the characteristics of economical training and efficient inference. It consists of 236B parameters, 21B of which are used to activate each marker. Compared with DeepSeek67B, DeepSeek-V2 has stronger performance, while saving 42.5% of training costs, reducing KV cache by 93.3%, and increasing the maximum generation throughput to 5.76 times. DeepSeek is a company exploring general artificial intelligence
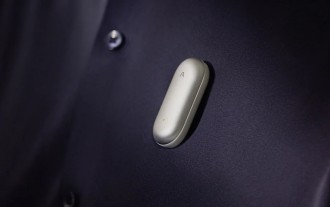
Plaud, the company behind the Plaud Note AI Voice Recorder (available on Amazon for $159), has announced a new product. Dubbed the NotePin, the device is described as an AI memory capsule, and like the Humane AI Pin, this is wearable. The NotePin is
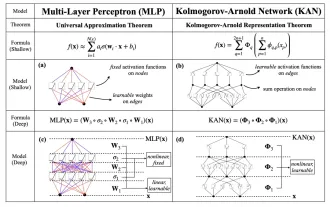
Earlier this month, researchers from MIT and other institutions proposed a very promising alternative to MLP - KAN. KAN outperforms MLP in terms of accuracy and interpretability. And it can outperform MLP running with a larger number of parameters with a very small number of parameters. For example, the authors stated that they used KAN to reproduce DeepMind's results with a smaller network and a higher degree of automation. Specifically, DeepMind's MLP has about 300,000 parameters, while KAN only has about 200 parameters. KAN has a strong mathematical foundation like MLP. MLP is based on the universal approximation theorem, while KAN is based on the Kolmogorov-Arnold representation theorem. As shown in the figure below, KAN has
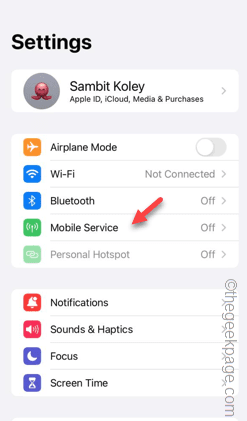
Facing lag, slow mobile data connection on iPhone? Typically, the strength of cellular internet on your phone depends on several factors such as region, cellular network type, roaming type, etc. There are some things you can do to get a faster, more reliable cellular Internet connection. Fix 1 – Force Restart iPhone Sometimes, force restarting your device just resets a lot of things, including the cellular connection. Step 1 – Just press the volume up key once and release. Next, press the Volume Down key and release it again. Step 2 – The next part of the process is to hold the button on the right side. Let the iPhone finish restarting. Enable cellular data and check network speed. Check again Fix 2 – Change data mode While 5G offers better network speeds, it works better when the signal is weaker

To learn more about AIGC, please visit: 51CTOAI.x Community https://www.51cto.com/aigc/Translator|Jingyan Reviewer|Chonglou is different from the traditional question bank that can be seen everywhere on the Internet. These questions It requires thinking outside the box. Large Language Models (LLMs) are increasingly important in the fields of data science, generative artificial intelligence (GenAI), and artificial intelligence. These complex algorithms enhance human skills and drive efficiency and innovation in many industries, becoming the key for companies to remain competitive. LLM has a wide range of applications. It can be used in fields such as natural language processing, text generation, speech recognition and recommendation systems. By learning from large amounts of data, LLM is able to generate text
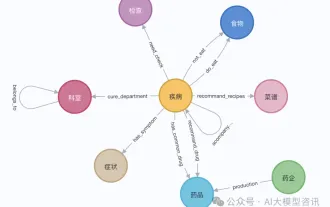
Graph Retrieval Enhanced Generation (GraphRAG) is gradually becoming popular and has become a powerful complement to traditional vector search methods. This method takes advantage of the structural characteristics of graph databases to organize data in the form of nodes and relationships, thereby enhancing the depth and contextual relevance of retrieved information. Graphs have natural advantages in representing and storing diverse and interrelated information, and can easily capture complex relationships and properties between different data types. Vector databases are unable to handle this type of structured information, and they focus more on processing unstructured data represented by high-dimensional vectors. In RAG applications, combining structured graph data and unstructured text vector search allows us to enjoy the advantages of both at the same time, which is what this article will discuss. structure
