Python装饰器基础详解
装饰器(decorator)是一种高级Python语法。装饰器可以对一个函数、方法或者类进行加工。在Python中,我们有多种方法对函数和类进行加工,比如在Python闭包中,我们见到函数对象作为某一个函数的返回结果。相对于其它方式,装饰器语法简单,代码可读性高。因此,装饰器在Python项目中有广泛的应用。
前面快速介绍了装饰器的语法,在这里,我们将深入装饰器内部工作机制,更详细更系统地介绍装饰器的内容,并学习自己编写新的装饰器的更多高级语法。
什么是装饰器
装饰是为函数和类指定管理代码的一种方式。Python装饰器以两种形式呈现:
【1】函数装饰器在函数定义的时候进行名称重绑定,提供一个逻辑层来管理函数和方法或随后对它们的调用。
【2】类装饰器在类定义的时候进行名称重绑定,提供一个逻辑层来管理类,或管理随后调用它们所创建的实例。
简而言之,装饰器提供了一种方法,在函数和类定义语句的末尾插入自动运行的代码——对于函数装饰器,在def的末尾;对于类装饰器,在class的末尾。这样的代码可以扮演不同的角色。
装饰器提供了一些和代码维护性和审美相关的有点。此外,作为结构化工具,装饰器自然地促进了代码封装,这减少了冗余性并使得未来变得更容易。
函数装饰器
通过在一个函数的def语句的末尾运行另一个函数,把最初的函数名重新绑定到结果。
用法
装饰器在紧挨着定义一个函数或方法的def语句之前的一行编写,并且它由@符号以及紧随其后的对于元函数的一个引用组成——这是管理另一个函数的一个函数(或其他可调用对象)。
在编码上,函数装饰器自动将如下语法:
@decorator def F(arg): ... F(99)
映射为这个对等形式:
def F(arg): ... F = decorator(F) F(99)
这里的装饰器是一个单参数的可调用对象,它返回与F具有相同数目的参数的一个可调用对象。
当随后调用F函数的时候,它自动调用装饰器所返回的对象。
换句话说,装饰实际把如下的第一行映射为第二行(尽管装饰器只在装饰的时候运行一次)
fun(6,7) decorator(func)(6,7)
这一自动名称重绑定也解释了之前介绍的静态方法和property装饰器语法的原因:
class C: @staticmethod def meth(...):... @property def name(self):...
实现
装饰器自身是返回可调用对象的可调用对象。实际上,它可以是任意类型的可调用对象,并且返回任意类型的可调用对象:函数和类的任何组合都可以使用,尽管一些组合更适合于特定的背景。
有一种常用的编码模式——装饰器返回了一个包装器,包装器把最初的函数保持到一个封闭的作用域中:
def decorator(F): def wrapper(*args): # 使用 F 和 *args # 调用原来的F(*args) return wrapper @decorator def func(x,y): ... func(6,7)
当随后调用名称func的时候,它确实调用装饰器所返回的包装器函数;随后包装器函数可能运行最初的func,因为它在一个封闭的作用域中仍然可以使用。
为了对类做同样的事情,我们可以重载调用操作:
class decorator: def __init__(self,func): self.func = func def __call__(self,*args): # 使用self.func和args # self.func(*args)调用最初的func @decorator def func(x,y): ... func(6,7)
但是,要注意的是,基于类的代码中,它对于拦截简单函数有效,但当它应用于类方法函数时,并不很有效:
如下反例:
class decorator: def __init__(self,func): self.func = func def __call__(self,*args): # 调用self.func(*args)失败,因为C实例参数无法传递 class C: @decorator def method(self,x,y): ...
这时候装饰的方法重绑定到一个类的方法上,而不是一个简单的函数,这一点带来的问题是,当装饰器的方法__call__随后运行的时候,其中的self接受装饰器类实例,并且类C的实例不会包含到一个*args中。
这时候,嵌套函数的替代方法工作得更好:
def decorator: def warpper(*args): # ... return wrapper @decorator def func(x,y): ... func(6,7) class C: @decorator def method(self,x,y): ... x = C() x.method(6,7)
类装饰器
类装饰器与函数装饰器使用相同的语法和非常相似的编码方式。类装饰器是管理类的一种方式,或者用管理或扩展类所创建的实例的额外逻辑,来包装实例构建调用。
用法
假设类装饰器返回一个可调用对象的一个单参数的函数,类装饰器的语法为:
@decorator class C: ... x = C(99)
等同于下面的语法:
class C: ... C = decorator(C) x = C(99)
直接效果是随后调用类名会创建一个实例,该实例会触发装饰器所返回的可调用对象,而不是调用最初的类自身。
实现
类装饰器返回的可调用对象,通常创建并返回最初的类的一个新的实例,以某种方式来扩展对其接口的管理。例如,下面的实例插入一个对象来拦截一个类实例的未定义的属性:
def decorator(cls): class Wrapper: def __init__(self,*args): self.wrapped = cls(*args) def __getattr__(self,name): return getattr(self.wrapped,name) return Wrapper @decorator class C: # C = decorator(C) def __init__(self,x,y): # Run by Wrapper.__init__ self.attr = 'spam' x = C(6,7) # 等价于Wrapper(6,7) print(x.attr)
在这个例子中,装饰器把类的名称重新绑定到另一个类,这个类在一个封闭的作用域中保持了最初的类。
就像函数装饰器一样,类装饰器通常可以编写为一个创建并返回可调用对象的“工厂”函数。
装饰器嵌套
有时候,一个装饰器不够,装饰器语法允许我们向一个装饰器的函数或方法添加包装器逻辑的多个层。这种形式的装饰器的语法为:
@A @B @C def f(...): ...
如下这样转换:
def f(...): ... f = A(B(C(f)))
这里,最初的函数通过3个不同的装饰器传递,每个装饰器处理前一个结果。
装饰器参数
函数装饰器和类装饰器都能接受参数,如下:
@decorator(A,B) def F(arg): ... F(99)
自动映射到其对等形式:
def F(arg): ... F = decorator(A,B)(F) F(99)
装饰器参数在装饰之前就解析了,并且它们通常用来保持状态信息供随后的调用使用。例如,这个例子中的装饰器函数,可能采用如下形式:
def decorator(A,B): # 保存或使用A和B def actualDecorator(F): # 保存或使用函数 F # 返回一个可调用对象 return callable return actualDecorator
以上,这是装饰器的基础知识,接下来将学习编写自己的装饰器。

Hot AI Tools

Undresser.AI Undress
AI-powered app for creating realistic nude photos

AI Clothes Remover
Online AI tool for removing clothes from photos.

Undress AI Tool
Undress images for free

Clothoff.io
AI clothes remover

Video Face Swap
Swap faces in any video effortlessly with our completely free AI face swap tool!

Hot Article

Hot Tools

Notepad++7.3.1
Easy-to-use and free code editor

SublimeText3 Chinese version
Chinese version, very easy to use

Zend Studio 13.0.1
Powerful PHP integrated development environment

Dreamweaver CS6
Visual web development tools

SublimeText3 Mac version
God-level code editing software (SublimeText3)

Hot Topics










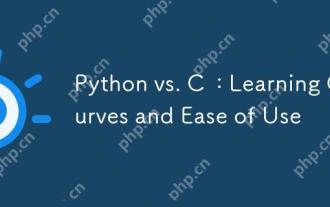
Python is easier to learn and use, while C is more powerful but complex. 1. Python syntax is concise and suitable for beginners. Dynamic typing and automatic memory management make it easy to use, but may cause runtime errors. 2.C provides low-level control and advanced features, suitable for high-performance applications, but has a high learning threshold and requires manual memory and type safety management.
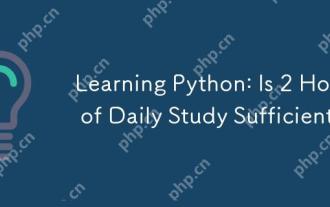
Is it enough to learn Python for two hours a day? It depends on your goals and learning methods. 1) Develop a clear learning plan, 2) Select appropriate learning resources and methods, 3) Practice and review and consolidate hands-on practice and review and consolidate, and you can gradually master the basic knowledge and advanced functions of Python during this period.
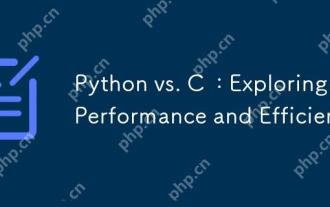
Python is better than C in development efficiency, but C is higher in execution performance. 1. Python's concise syntax and rich libraries improve development efficiency. 2.C's compilation-type characteristics and hardware control improve execution performance. When making a choice, you need to weigh the development speed and execution efficiency based on project needs.
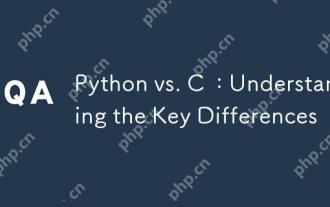
Python and C each have their own advantages, and the choice should be based on project requirements. 1) Python is suitable for rapid development and data processing due to its concise syntax and dynamic typing. 2)C is suitable for high performance and system programming due to its static typing and manual memory management.
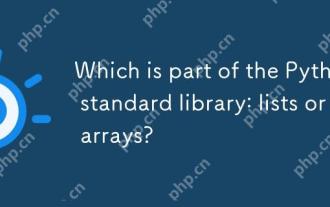
Pythonlistsarepartofthestandardlibrary,whilearraysarenot.Listsarebuilt-in,versatile,andusedforstoringcollections,whereasarraysareprovidedbythearraymoduleandlesscommonlyusedduetolimitedfunctionality.
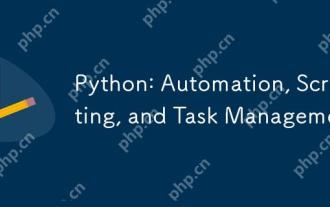
Python excels in automation, scripting, and task management. 1) Automation: File backup is realized through standard libraries such as os and shutil. 2) Script writing: Use the psutil library to monitor system resources. 3) Task management: Use the schedule library to schedule tasks. Python's ease of use and rich library support makes it the preferred tool in these areas.
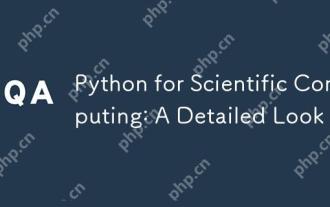
Python's applications in scientific computing include data analysis, machine learning, numerical simulation and visualization. 1.Numpy provides efficient multi-dimensional arrays and mathematical functions. 2. SciPy extends Numpy functionality and provides optimization and linear algebra tools. 3. Pandas is used for data processing and analysis. 4.Matplotlib is used to generate various graphs and visual results.
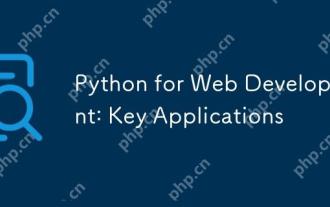
Key applications of Python in web development include the use of Django and Flask frameworks, API development, data analysis and visualization, machine learning and AI, and performance optimization. 1. Django and Flask framework: Django is suitable for rapid development of complex applications, and Flask is suitable for small or highly customized projects. 2. API development: Use Flask or DjangoRESTFramework to build RESTfulAPI. 3. Data analysis and visualization: Use Python to process data and display it through the web interface. 4. Machine Learning and AI: Python is used to build intelligent web applications. 5. Performance optimization: optimized through asynchronous programming, caching and code
