Spark on YARN
Spark在YARN中有yarn-cluster和yarn-client两种运行模式: I. Yarn Cluster Spark Driver首先作为一个ApplicationMaster在YARN集群中启动,客户端提交给ResourceManager的每一个job都会在集群的worker节点上分配一个唯一的ApplicationMaster,由该Application
Spark在YARN中有yarn-cluster和yarn-client两种运行模式:
I. Yarn Cluster
Spark Driver首先作为一个ApplicationMaster在YARN集群中启动,客户端提交给ResourceManager的每一个job都会在集群的worker节点上分配一个唯一的ApplicationMaster,由该ApplicationMaster管理全生命周期的应用。因为Driver程序在YARN中运行,所以事先不用启动Spark Master/Client,应用的运行结果不能在客户端显示(可以在history server中查看),所以最好将结果保存在HDFS而非stdout输出,客户端的终端显示的是作为YARN的job的简单运行状况。
by @Sandy Ryza
by 明风@taobao
从terminal的output中看到任务初始化更详细的四个步骤:
14/09/28 11:24:52 INFO RMProxy: Connecting to ResourceManager at hdp01/172.19.1.231:8032 14/09/28 11:24:52 INFO Client: Got Cluster metric info from ApplicationsManager (ASM), number of NodeManagers: 4 14/09/28 11:24:52 INFO Client: Queue info ... queueName: root.default, queueCurrentCapacity: 0.0, queueMaxCapacity: -1.0, queueApplicationCount = 0, queueChildQueueCount = 0 14/09/28 11:24:52 INFO Client: Max mem capabililty of a single resource in this cluster 8192 14/09/28 11:24:53 INFO Client: Uploading file:/usr/lib/spark/examples/lib/spark-examples_2.10-1.0.0-cdh5.1.0.jar to hdfs://hdp01:8020/user/spark/.sparkStaging/application_1411874193696_0003/spark-examples_2.10-1.0.0-cdh5.1.0.jar 14/09/28 11:24:54 INFO Client: Uploading file:/usr/lib/spark/assembly/lib/spark-assembly-1.0.0-cdh5.1.0-hadoop2.3.0-cdh5.1.0.jar to hdfs://hdp01:8020/user/spark/.sparkStaging/application_1411874193696_0003/spark-assembly-1.0.0-cdh5.1.0-hadoop2.3.0-cdh5.1.0.jar 14/09/28 11:24:55 INFO Client: Setting up the launch environment 14/09/28 11:24:55 INFO Client: Setting up container launch context 14/09/28 11:24:55 INFO Client: Command for starting the Spark ApplicationMaster: List($JAVA_HOME/bin/java, -server, -Xmx512m, -Djava.io.tmpdir=$PWD/tmp, -Dspark.master=\"spark://hdp01:7077\", -Dspark.app.name=\"org.apache.spark.examples.SparkPi\", -Dspark.eventLog.enabled=\"true\", -Dspark.eventLog.dir=\"/user/spark/applicationHistory\", -Dlog4j.configuration=log4j-spark-container.properties, org.apache.spark.deploy.yarn.ApplicationMaster, --class, org.apache.spark.examples.SparkPi, --jar , file:/usr/lib/spark/examples/lib/spark-examples_2.10-1.0.0-cdh5.1.0.jar, , --executor-memory, 1024, --executor-cores, 1, --num-executors , 2, 1>, /stdout, 2>, /stderr) 14/09/28 11:24:55 INFO Client: Submitting application to ASM 14/09/28 11:24:55 INFO YarnClientImpl: Submitted application application_1411874193696_0003 14/09/28 11:24:56 INFO Client: Application report from ASM: application identifier: application_1411874193696_0003 appId: 3 clientToAMToken: null appDiagnostics: appMasterHost: N/A appQueue: root.spark appMasterRpcPort: -1 appStartTime: 1411874695327 yarnAppState: ACCEPTED distributedFinalState: UNDEFINED appTrackingUrl: http://hdp01:8088/proxy/application_1411874193696_0003/ appUser: spark
1. 由client向ResourceManager提交请求,并上传jar到HDFS上
这期间包括四个步骤:
a). 连接到RM
b). 从RM ASM(ApplicationsManager )中获得metric、queue和resource等信息。
c). upload app jar and spark-assembly jar
d). 设置运行环境和container上下文(launch-container.sh等脚本)
2. ResouceManager向NodeManager申请资源,创建Spark ApplicationMaster(每个SparkContext都有一个ApplicationMaster)
3. NodeManager启动Spark App Master,并向ResourceManager AsM注册
4. Spark ApplicationMaster从HDFS中找到jar文件,启动DAGscheduler和YARN Cluster Scheduler
5. ResourceManager向ResourceManager AsM注册申请container资源(INFO YarnClientImpl: Submitted application)
6. ResourceManager通知NodeManager分配Container,这时可以收到来自ASM关于container的报告。(每个container的对应一个executor)
7. Spark ApplicationMaster直接和container(executor)进行交互,完成这个分布式任务。
需要注意的是:
a). Spark中的localdir会被yarn.nodemanager.local-dirs替换
b). 允许失败的节点数(spark.yarn.max.worker.failures)为executor数量的两倍数量,最小为3.
c). SPARK_YARN_USER_ENV传递给spark进程的环境变量
d). 传递给app的参数应该通过–args指定。
部署:
环境介绍:
hdp0[1-4]四台主机
hadoop使用CDH 5.1版本: hadoop-2.3.0+cdh5.1.0+795-1.cdh5.1.0.p0.58.el6.x86_64
直接下载对应2.3.0的pre-build版本http://spark.apache.org/downloads.html
下载完毕后解压,检查spark-assembly目录:
file /home/spark/spark-1.1.0-bin-hadoop2.3/lib/spark-assembly-1.1.0-hadoop2.3.0.jar
/home/spark/spark-1.1.0-bin-hadoop2.3/lib/spark-assembly-1.1.0-hadoop2.3.0.jar: Zip archive data, at least v2.0 to extract
然后输出环境变量HADOOP_CONF_DIR/YARN_CONF_DIR和SPARK_JAR(可以设置到spark-env.sh中)
export HADOOP_CONF_DIR=/etc/hadoop/etc
export SPARK_JAR=/home/spark/spark-1.1.0-bin-hadoop2.3/lib/spark-assembly-1.1.0-hadoop2.3.0.jar
如果使用cloudera manager 5,在Spark Service的操作中可以找到Upload Spark Jar将spark-assembly上传到HDFS上。
Spark Jar Location (HDFS) spark_jar_hdfs_path |
/user/spark/share/lib/spark-assembly.jar 默认值 |
The location of the Spark jar in HDFS |
Spark History Location (HDFS) spark.eventLog.dir |
/user/spark/applicationHistory 默认值 |
The location of Spark application history logs in HDFS. Changing this value will not move existing logs to the new location. |
提交任务,此时在YARN的web UI和history Server上就可以看到运行状态信息。
spark-submit --class org.apache.spark.examples.SparkPi --master yarn-cluster /usr/lib/spark/examples/lib/spark-examples_2.10-1.0.0-cdh5.1.0.jar
II. yarn-client
(YarnClientClusterScheduler)查看对应类的文件
在yarn-client模式下,Driver运行在Client上,通过ApplicationMaster向RM获取资源。本地Driver负责与所有的executor container进行交互,并将最后的结果汇总。结束掉终端,相当于kill掉这个spark应用。一般来说,如果运行的结果仅仅返回到terminal上时需要配置这个。
客户端的Driver将应用提交给Yarn后,Yarn会先后启动ApplicationMaster和executor,另外ApplicationMaster和executor都 是装载在container里运行,container默认的内存是1G,ApplicationMaster分配的内存是driver- memory,executor分配的内存是executor-memory。同时,因为Driver在客户端,所以程序的运行结果可以在客户端显 示,Driver以进程名为SparkSubmit的形式存在。
配置YARN-Client模式同样需要HADOOP_CONF_DIR/YARN_CONF_DIR和SPARK_JAR变量。
提交任务测试:
spark-submit --class org.apache.spark.examples.SparkPi --deploy-mode client /usr/lib/spark/examples/lib/spark-examples_2.10-1.0.0-cdh5.1.0.jar terminal output: 14/09/28 11:18:34 INFO Client: Command for starting the Spark ApplicationMaster: List($JAVA_HOME/bin/java, -server, -Xmx512m, -Djava.io.tmpdir=$PWD/tmp, -Dspark.tachyonStore.folderName=\"spark-9287f0f2-2e72-4617-a418-e0198626829b\", -Dspark.eventLog.enabled=\"true\", -Dspark.yarn.secondary.jars=\"\", -Dspark.driver.host=\"hdp01\", -Dspark.driver.appUIHistoryAddress=\"\", -Dspark.app.name=\"Spark Pi\", -Dspark.jars=\"file:/usr/lib/spark/examples/lib/spark-examples_2.10-1.0.0-cdh5.1.0.jar\", -Dspark.fileserver.uri=\"http://172.19.17.231:53558\", -Dspark.eventLog.dir=\"/user/spark/applicationHistory\", -Dspark.master=\"yarn-client\", -Dspark.driver.port=\"35938\", -Dspark.httpBroadcast.uri=\"http://172.19.17.231:43804\", -Dlog4j.configuration=log4j-spark-container.properties, org.apache.spark.deploy.yarn.ExecutorLauncher, --class, notused, --jar , null, --args 'hdp01:35938' , --executor-memory, 1024, --executor-cores, 1, --num-executors , 2, 1>, /stdout, 2>, /stderr) 14/09/28 11:18:34 INFO Client: Submitting application to ASM 14/09/28 11:18:34 INFO YarnClientSchedulerBackend: Application report from ASM: appMasterRpcPort: -1 appStartTime: 1411874314198 yarnAppState: ACCEPTED ......
##最后将结果输出到terminal中
Pi is roughly 3.14528
^^
原文地址:Spark on YARN, 感谢原作者分享。

Hot AI Tools

Undresser.AI Undress
AI-powered app for creating realistic nude photos

AI Clothes Remover
Online AI tool for removing clothes from photos.

Undress AI Tool
Undress images for free

Clothoff.io
AI clothes remover

Video Face Swap
Swap faces in any video effortlessly with our completely free AI face swap tool!

Hot Article

Hot Tools

Notepad++7.3.1
Easy-to-use and free code editor

SublimeText3 Chinese version
Chinese version, very easy to use

Zend Studio 13.0.1
Powerful PHP integrated development environment

Dreamweaver CS6
Visual web development tools

SublimeText3 Mac version
God-level code editing software (SublimeText3)

Hot Topics
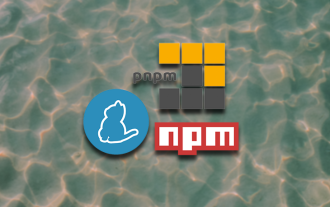
This article will take you through the three JavaScript package managers (npm, yarn, pnpm), compare these three package managers, and talk about the differences and relationships between npm, yarn, and pnpm. I hope it will be helpful to everyone. Please help, if you have any questions please point them out!
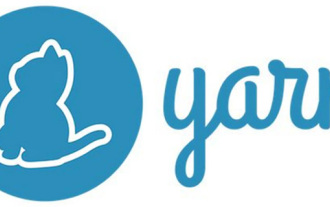
Yarn, like npm, is also a JavaScript package management tool. In this article, I will introduce you to the yarn package management tool. I hope it will be helpful to you!
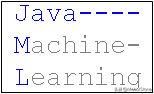
ChatGPT has been popular for more than half a year this year, and its popularity has not dropped at all. Deep learning and NLP have also returned to everyone's attention. Some friends in the company are asking me, as a Java developer, how to get started with artificial intelligence. It is time to take out the hidden Java library for learning AI and introduce it to everyone. These libraries and frameworks provide a wide range of tools and algorithms for machine learning, deep learning, natural language processing, and more. Depending on the specific needs of your AI project, you can choose the most appropriate library or framework and start experimenting with different algorithms to build your AI solution. 1.Deeplearning4j It is an open source distributed deep learning library for Java and Scala. Deeplearning
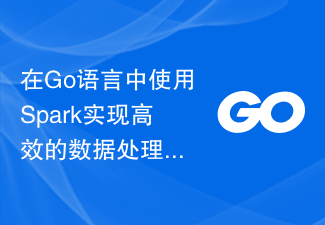
With the advent of the big data era, data processing has become increasingly important. For various data processing tasks, different technologies have emerged. Among them, Spark, as a technology suitable for large-scale data processing, has been widely used in various fields. In addition, Go language, as an efficient programming language, has also received more and more attention in recent years. In this article, we will explore how to use Spark in Go language to achieve efficient data processing. We will first introduce some basic concepts and principles of Spark
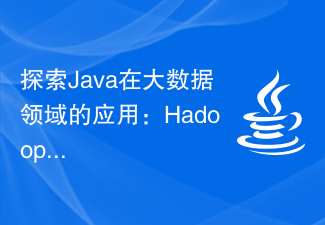
Java big data technology stack: Understand the application of Java in the field of big data, such as Hadoop, Spark, Kafka, etc. As the amount of data continues to increase, big data technology has become a hot topic in today's Internet era. In the field of big data, we often hear the names of Hadoop, Spark, Kafka and other technologies. These technologies play a vital role, and Java, as a widely used programming language, also plays a huge role in the field of big data. This article will focus on the application of Java in large
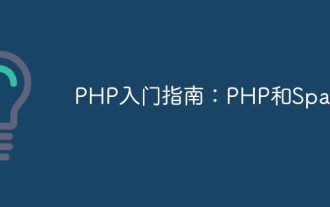
PHP is a very popular server-side programming language because it is easy to learn, open source, and cross-platform. Currently, many large companies use PHP language to build applications, such as Facebook and WordPress. Spark is a fast and lightweight development framework for building web applications. It is based on Java Virtual Machine (JVM) and works with PHP. This article will introduce how to build web applications using PHP and Spark. What is PHP? PH
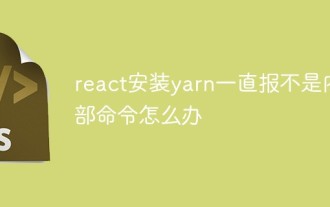
The solution to the problem that the react installation yarn keeps reporting that it is not an internal command: 1. Uninstall yarn through the command "pm uninstall yarn -g"; 2. Reinstall yarn using "npm install yarn"; 3. Add "C:\ WINDOWS\system32\node_modules\yarn\bin"; 4. Re-open cmd and execute the "yarn -v" command.
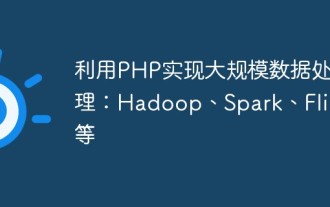
As the amount of data continues to increase, large-scale data processing has become a problem that enterprises must face and solve. Traditional relational databases can no longer meet this demand. For the storage and analysis of large-scale data, distributed computing platforms such as Hadoop, Spark, and Flink have become the best choices. In the selection process of data processing tools, PHP is becoming more and more popular among developers as a language that is easy to develop and maintain. In this article, we will explore how to leverage PHP for large-scale data processing and how
