IntegrationServices架构概述
Integration Services平台包括许多组件,但在最高层次上,它由4个主要部分组成。 1、Integration Services运行时。SSIS运行时提供了运行SSIS包所需的核心功能,包括执行、记录、配置、调试等。 2、数据流引擎。SSIS数据库引擎(也成为管道)提供了将数据从源
Integration Services平台包括许多组件,但在最高层次上,它由4个主要部分组成。
1、Integration Services运行时。SSIS运行时提供了运行SSIS包所需的核心功能,包括执行、记录、配置、调试等。
2、数据流引擎。SSIS数据库引擎(也成为管道)提供了将数据从源移动到SSIS包中的目标所需的核心ETL功能,包括管理管道所基于的内存缓冲区,以及组成包的数据流逻辑的源、转换盒目标。
3、Integration Services对象模型。SSIS对象模型是一个托管.net应用程序编程接口(API),支持工具、使用工具和组件与SSIS运行时和数据流引擎交互。
4、Integration Services服务。SSIS服务是一种 Windows服务,提供了存储和管理SSIS包的功能。
这4个关键组件构成了SSIS的基础,但实际上它们只是SSIS架构的冰山一角。当然,主要的工作单元是SSIS包。

Hot AI Tools

Undresser.AI Undress
AI-powered app for creating realistic nude photos

AI Clothes Remover
Online AI tool for removing clothes from photos.

Undress AI Tool
Undress images for free

Clothoff.io
AI clothes remover

Video Face Swap
Swap faces in any video effortlessly with our completely free AI face swap tool!

Hot Article

Hot Tools

Notepad++7.3.1
Easy-to-use and free code editor

SublimeText3 Chinese version
Chinese version, very easy to use

Zend Studio 13.0.1
Powerful PHP integrated development environment

Dreamweaver CS6
Visual web development tools

SublimeText3 Mac version
God-level code editing software (SublimeText3)

Hot Topics










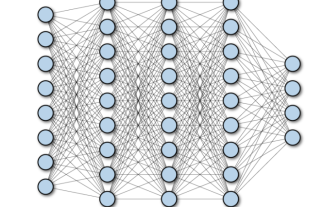
The concept of deep learning originates from the research of artificial neural networks. A multi-layer perceptron containing multiple hidden layers is a deep learning structure. Deep learning combines low-level features to form more abstract high-level representations to represent categories or characteristics of data. It is able to discover distributed feature representations of data. Deep learning is a type of machine learning, and machine learning is the only way to achieve artificial intelligence. So, what are the differences between various deep learning system architectures? 1. Fully Connected Network (FCN) A fully connected network (FCN) consists of a series of fully connected layers, with every neuron in each layer connected to every neuron in another layer. Its main advantage is that it is "structure agnostic", i.e. no special assumptions about the input are required. Although this structural agnostic makes the complete
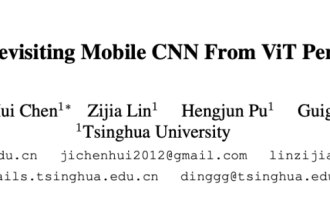
Paper address: https://arxiv.org/abs/2307.09283 Code address: https://github.com/THU-MIG/RepViTRepViT performs well in the mobile ViT architecture and shows significant advantages. Next, we explore the contributions of this study. It is mentioned in the article that lightweight ViTs generally perform better than lightweight CNNs on visual tasks, mainly due to their multi-head self-attention module (MSHA) that allows the model to learn global representations. However, the architectural differences between lightweight ViTs and lightweight CNNs have not been fully studied. In this study, the authors integrated lightweight ViTs into the effective
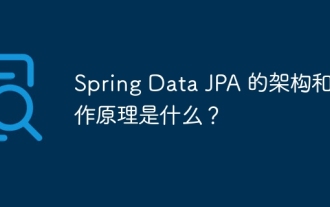
SpringDataJPA is based on the JPA architecture and interacts with the database through mapping, ORM and transaction management. Its repository provides CRUD operations, and derived queries simplify database access. Additionally, it uses lazy loading to only retrieve data when necessary, thus improving performance.
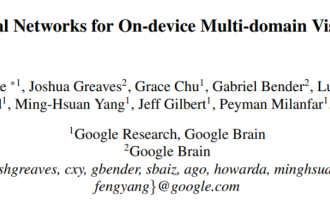
Deep learning models for vision tasks (such as image classification) are usually trained end-to-end with data from a single visual domain (such as natural images or computer-generated images). Generally, an application that completes vision tasks for multiple domains needs to build multiple models for each separate domain and train them independently. Data is not shared between different domains. During inference, each model will handle a specific domain. input data. Even if they are oriented to different fields, some features of the early layers between these models are similar, so joint training of these models is more efficient. This reduces latency and power consumption, and reduces the memory cost of storing each model parameter. This approach is called multi-domain learning (MDL). In addition, MDL models can also outperform single
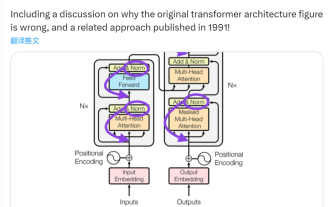
Some time ago, a tweet pointing out the inconsistency between the Transformer architecture diagram and the code in the Google Brain team's paper "AttentionIsAllYouNeed" triggered a lot of discussion. Some people think that Sebastian's discovery was an unintentional mistake, but it is also surprising. After all, considering the popularity of the Transformer paper, this inconsistency should have been mentioned a thousand times. Sebastian Raschka said in response to netizen comments that the "most original" code was indeed consistent with the architecture diagram, but the code version submitted in 2017 was modified, but the architecture diagram was not updated at the same time. This is also the root cause of "inconsistent" discussions.
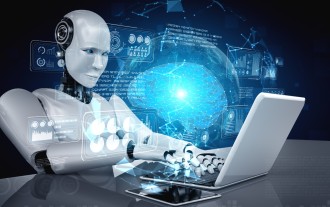
Artificial intelligence (AI) has changed the game in many industries, enabling businesses to improve efficiency, decision-making and customer experience. As AI continues to evolve and become more complex, it is critical that enterprises invest in the right infrastructure to support its development and deployment. A key aspect of this infrastructure is collaboration between IT and data science teams, as both play a critical role in ensuring the success of AI initiatives. The rapid development of artificial intelligence has led to increasing demands for computing power, storage and network capabilities. This demand puts pressure on traditional IT infrastructure, which was not designed to handle the complex and resource-intensive workloads required by AI. As a result, enterprises are now looking to build systems that can support AI workloads.
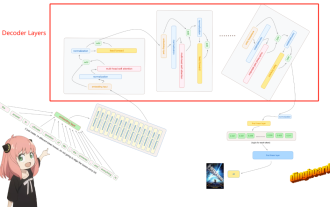
1. Architecture of Llama3 In this series of articles, we implement llama3 from scratch. The overall architecture of Llama3: Picture the model parameters of Llama3: Let's take a look at the actual values of these parameters in the Llama3 model. Picture [1] Context window (context-window) When instantiating the LlaMa class, the variable max_seq_len defines context-window. There are other parameters in the class, but this parameter is most directly related to the transformer model. The max_seq_len here is 8K. Picture [2] Vocabulary-size and AttentionL
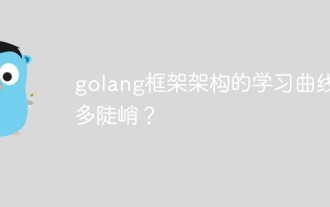
The learning curve of the Go framework architecture depends on familiarity with the Go language and back-end development and the complexity of the chosen framework: a good understanding of the basics of the Go language. It helps to have backend development experience. Frameworks that differ in complexity lead to differences in learning curves.
