Hermes与开源的Solr、ElasticSearch的不同
Hermes与开源的Solr、ElasticSearch的不同 谈到Hermes的索引技术,相信很多同学都会想到Solr、ElasticSearch。Solr、ElasticSearch在真可谓是大名鼎鼎,是两个顶级项目,最近有些同学经常问我,开源世界有Solr、ElasticSearch为什么还要使用Hermes? 在回答这
Hermes与开源的Solr、ElasticSearch的不同谈到Hermes的索引技术,相信很多同学都会想到Solr、ElasticSearch。Solr、ElasticSearch在真可谓是大名鼎鼎,是两个顶级项目,最近有些同学经常问我,“开源世界有Solr、ElasticSearch为什么还要使用Hermes?”
在回答这个问题之前,大家可以思考一个问题,既然已经有了Oracle、MySQL等数据库为什么大家还要使用Hadoo[下的Hive、Spark? Oracle和MySQL也有集群版,也可以分布式,那Hadoop与Hive的出现是不是多余的?
Hermes的出现,并不是为了替代Solr、ES的,就像Hadoop的出现并不是为了干掉Oracle和MySQL一样。而是为了满足不同层面的需求。
一、Hermes与Solr,ES定位不同
Solr\ES :偏重于为小规模的数据提供全文检索服务;Hermes:则更倾向于为大规模的数据仓库提供索引支持,为大规模数据仓库提供即席分析的解决方案,并降低数据仓库的成本,Hermes数据量更“大”。
Solr、ES的使用特点如下:
1. 源自搜索引擎,侧重搜索与全文检索。
2. 数据规模从几百万到千万不等,数据量过亿的集群特别少。
Ps:有可能存在个别系统数据量过亿,但这并不是普遍现象(就像Oracle的表里的数据规模有可能超过Hive里一样,但需要小型机)。
Hermes:的使用特点如下:
1. 一个基于大索引技术的海量数据实时检索分析平台。侧重数据分析。
2. 数据规模从几亿到万亿不等。最小的表也是千万级别。
在 腾讯17 台TS5机器,就可以处理每天450亿的数据(每条数据1kb左右),数据可以保存一个月之久。
二、Hermes与Solr,ES在技术实现上也会有一些区别
Solr、ES在大索引上存在的问题:
1. 一级跳跃表是完全Load在内存中的。
这种方式需要消耗很多内存不说,首次打开索引的加载速度会特别慢.
在Solr\ES中的索引是一直处于打开状态的,不会频繁的打开与关闭;
这种模式会制约一台机器的索引数量与索引规模,通常一台机器固定负责某个业务的索引。
2. 为了排序,将列的全部值Load到放到内存里。
排序和统计(sum,max,min)的时候,是通过遍历倒排表,将某一列的全部值都Load到内存里,然后基于内存数据进行统计,即使一次查询只会用到其中的一条记录,也会将整列的全部值都Load到内存里,太浪费资源,首次查询的性能太差。
数据规模受物理内存限制很大,索引规模上千万后OOM是常事。
3. 索引存储在本地硬盘,恢复难
一旦机器损坏,数据即使没有丢失,一个几T的索引,仅仅数据copy时间就需要好几个小时才能搞定。
4. 集群规模太小
支持Master/Slave模式,但是跟传统MySQL数据库一样,集群规模并没有特别大的(百台以内)。这种模式处理集群规模受限外,每次扩容的数据迁移将是一件非常痛苦的事情,数据迁移时间太久。
5. 数据倾斜问题
倒排检索即使某个词语存在数据倾斜,因数据量比较小,也可以将全部的doc list都读取过来(比如说男、女),这个doc list会占用较大的内存进行Cache,当然在数据规模较小的情况下占用内存不是特别多,查询命中率很高,会提升检索速度,但是数据规模上来后,这里的内存问题越来越严重。
6. 节点和数据规模受限
Merger Server只能是一个,制约了查询的节点数量;数据不能进行动态分区,数据规模上来后单个索引太大。
7. 高并发导入的情况下, GC占用CPU太高,多线程并发性能上不去。
AttributeSource使用了WeakHashMap来管理类的实例化,并使用了全局锁,无论加了多大的线程,导入性能上不去。
AttributeSource与NumbericField,使用了大量的LinkHashMap以及很多无用的对象,导致每一条记录都要在内存中创建很多无用的对象,造成了JVM要频繁的回收这些对象,CPU消耗过高。
FieldCacheImpl使用的WeakHashMap有BUG,大数据的情况下有OOM的风险。
单机导入性能在笔者的环境下(1kb的记录每台机器想突破2w/s 很难)
Solr与ES小结
并不是说Solr与ES的这种方式不好,在数据规模较小的情况下,Solr的这种处理方式表现优越,并发性能较好,Cache利用率较高,事实证明在生产领域Solr和ES是非常稳定的,并且性能也很卓越;但是在数据规模较大,并且数据在频繁的实时导入的情况下,就需要进行一些优化。
Hermes在索引上的改进:
1. 索引按需加载
大部分的索引处于关闭状态,只有真正用到索引才会去打开;一级跳跃表采用按需Load,并不会Load整个跳跃表,用来节省内存和提高打开索引的速度。Hermes经常会根据业务的不同动态的打开不同的索引,关闭那些不经常使用的索引,这样同样一台机器,可以被多种不同的业务所使用,机器利用率高。
2. 排序和统计按需加载
排序和统计并不会使用数据的真实值,而是通过标签技术将大数据转换成占用内存很小的数据标签,占用内存是原先的几十分之一。
另外不会将这个列的全部值都Load到内存里,而是用到哪些数据Load哪些数据,依然是按需Load。不用了的数据会从内存里移除。
3. 索引存储在HDFS中
理论上只要HDFS有空间,就可以不断的添加索引,索引规模不在严重受机器的物理内存和物理磁盘的限制。容灾和数据迁移容易得多。
4. 采用Gaia进行进程管理(腾讯版的Yarn)
数据在HDFS中,集群规模和扩容都是一件很容易的事情,Gaia在腾讯集群规模已达万台)。
5. 采用多条件组合跳跃降低数据倾斜
如果某个词语存在数据倾斜,则会与其他条件组合进行跳跃合并(参考doclist的skip list资料)。
6. 多级Merger与自定义分区
7. GC上进行了一些优化
自己进行内存管理,关键地方的内存对象的创建和释放java内部自己控制,减少GC的压力(类似Hbase的Block Buffer Cache)。
不使用WeakHashMap和全局锁,WeakHashMap使用不当容易内存泄露,而且性能太差。
用于分词的相关对象是共用的,减少反复的创建对象和释放对象。
1kb大小的数据,在笔者的环境下,一台机器每秒能处理4~8W条记录.

Hot AI Tools

Undresser.AI Undress
AI-powered app for creating realistic nude photos

AI Clothes Remover
Online AI tool for removing clothes from photos.

Undress AI Tool
Undress images for free

Clothoff.io
AI clothes remover

Video Face Swap
Swap faces in any video effortlessly with our completely free AI face swap tool!

Hot Article

Hot Tools

Notepad++7.3.1
Easy-to-use and free code editor

SublimeText3 Chinese version
Chinese version, very easy to use

Zend Studio 13.0.1
Powerful PHP integrated development environment

Dreamweaver CS6
Visual web development tools

SublimeText3 Mac version
God-level code editing software (SublimeText3)

Hot Topics
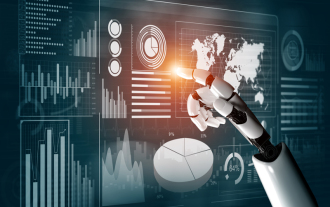
Text annotation is the work of corresponding labels or tags to specific content in text. Its main purpose is to provide additional information to the text for deeper analysis and processing, especially in the field of artificial intelligence. Text annotation is crucial for supervised machine learning tasks in artificial intelligence applications. It is used to train AI models to help more accurately understand natural language text information and improve the performance of tasks such as text classification, sentiment analysis, and language translation. Through text annotation, we can teach AI models to recognize entities in text, understand context, and make accurate predictions when new similar data appears. This article mainly recommends some better open source text annotation tools. 1.LabelStudiohttps://github.com/Hu
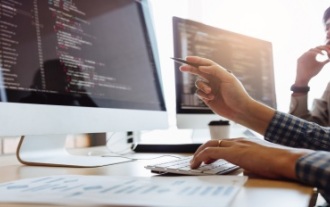
Image annotation is the process of associating labels or descriptive information with images to give deeper meaning and explanation to the image content. This process is critical to machine learning, which helps train vision models to more accurately identify individual elements in images. By adding annotations to images, the computer can understand the semantics and context behind the images, thereby improving the ability to understand and analyze the image content. Image annotation has a wide range of applications, covering many fields, such as computer vision, natural language processing, and graph vision models. It has a wide range of applications, such as assisting vehicles in identifying obstacles on the road, and helping in the detection and diagnosis of diseases through medical image recognition. . This article mainly recommends some better open source and free image annotation tools. 1.Makesens
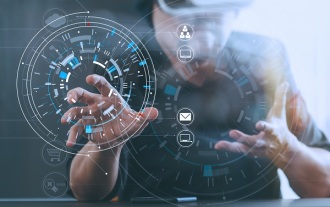
Face detection and recognition technology is already a relatively mature and widely used technology. Currently, the most widely used Internet application language is JS. Implementing face detection and recognition on the Web front-end has advantages and disadvantages compared to back-end face recognition. Advantages include reducing network interaction and real-time recognition, which greatly shortens user waiting time and improves user experience; disadvantages include: being limited by model size, the accuracy is also limited. How to use js to implement face detection on the web? In order to implement face recognition on the Web, you need to be familiar with related programming languages and technologies, such as JavaScript, HTML, CSS, WebRTC, etc. At the same time, you also need to master relevant computer vision and artificial intelligence technologies. It is worth noting that due to the design of the Web side
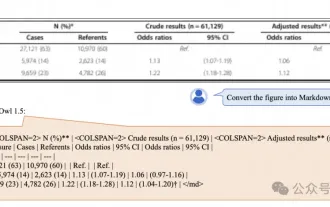
New SOTA for multimodal document understanding capabilities! Alibaba's mPLUG team released the latest open source work mPLUG-DocOwl1.5, which proposed a series of solutions to address the four major challenges of high-resolution image text recognition, general document structure understanding, instruction following, and introduction of external knowledge. Without further ado, let’s look at the effects first. One-click recognition and conversion of charts with complex structures into Markdown format: Charts of different styles are available: More detailed text recognition and positioning can also be easily handled: Detailed explanations of document understanding can also be given: You know, "Document Understanding" is currently An important scenario for the implementation of large language models. There are many products on the market to assist document reading. Some of them mainly use OCR systems for text recognition and cooperate with LLM for text processing.
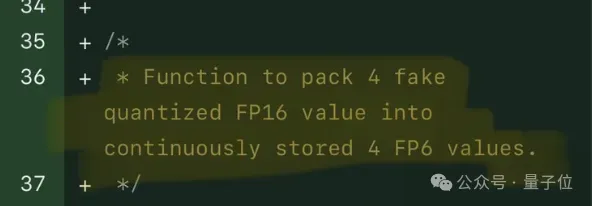
FP8 and lower floating point quantification precision are no longer the "patent" of H100! Lao Huang wanted everyone to use INT8/INT4, and the Microsoft DeepSpeed team started running FP6 on A100 without official support from NVIDIA. Test results show that the new method TC-FPx's FP6 quantization on A100 is close to or occasionally faster than INT4, and has higher accuracy than the latter. On top of this, there is also end-to-end large model support, which has been open sourced and integrated into deep learning inference frameworks such as DeepSpeed. This result also has an immediate effect on accelerating large models - under this framework, using a single card to run Llama, the throughput is 2.65 times higher than that of dual cards. one
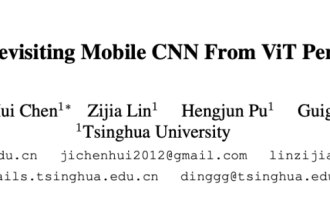
Paper address: https://arxiv.org/abs/2307.09283 Code address: https://github.com/THU-MIG/RepViTRepViT performs well in the mobile ViT architecture and shows significant advantages. Next, we explore the contributions of this study. It is mentioned in the article that lightweight ViTs generally perform better than lightweight CNNs on visual tasks, mainly due to their multi-head self-attention module (MSHA) that allows the model to learn global representations. However, the architectural differences between lightweight ViTs and lightweight CNNs have not been fully studied. In this study, the authors integrated lightweight ViTs into the effective
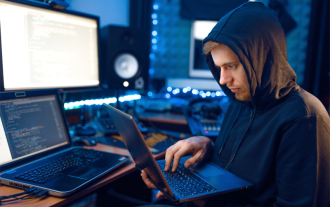
Let me introduce to you the latest AIGC open source project-AnimagineXL3.1. This project is the latest iteration of the anime-themed text-to-image model, aiming to provide users with a more optimized and powerful anime image generation experience. In AnimagineXL3.1, the development team focused on optimizing several key aspects to ensure that the model reaches new heights in performance and functionality. First, they expanded the training data to include not only game character data from previous versions, but also data from many other well-known anime series into the training set. This move enriches the model's knowledge base, allowing it to more fully understand various anime styles and characters. AnimagineXL3.1 introduces a new set of special tags and aesthetics
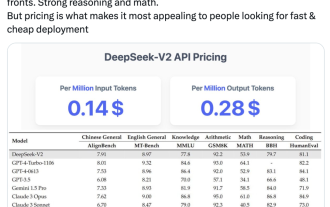
The latest large-scale domestic open source MoE model has become popular just after its debut. The performance of DeepSeek-V2 reaches GPT-4 level, but it is open source, free for commercial use, and the API price is only one percent of GPT-4-Turbo. Therefore, as soon as it was released, it immediately triggered a lot of discussion. Judging from the published performance indicators, DeepSeekV2's comprehensive Chinese capabilities surpass those of many open source models. At the same time, closed source models such as GPT-4Turbo and Wenkuai 4.0 are also in the first echelon. The comprehensive English ability is also in the same first echelon as LLaMA3-70B, and surpasses Mixtral8x22B, which is also a MoE. It also shows good performance in knowledge, mathematics, reasoning, programming, etc. And supports 128K context. Picture this
