


OWASP releases large language model network security and governance checklist
The biggest risk currently faced by artificial intelligence technology is that the development and application speed of large language models (LLM) and generative artificial intelligence technology have far exceeded the speed of security and governance.
The use of generative AI and large language model products from companies like OpenAI, Anthropic, Google, and Microsoft is growing exponentially. At the same time, open source large language model solutions are also growing rapidly. Open source artificial intelligence communities such as HuggingFace provide a large number of open source models, data sets and AI applications.
In order to promote the development of artificial intelligence, industry organizations such as OWASP, OpenSSF, and CISA are actively developing and providing key assets for artificial intelligence security and governance, such as OWASP AI Exchange, AI Security and Privacy Guide, and Big Language Model Ten Big Risk List (LLMTop10).
Recently, OWASP released a large language model network security and governance checklist, filling the gap in generative artificial intelligence security governance. The specific content is as follows:
OWASP’s definition of AI types and threats
OWASP’s Language Model Cybersecurity and Governance Checklist defines the differences between artificial intelligence, machine learning, generative artificial intelligence, and large language models.
For example, OWASP defines generative artificial intelligence as: a type of machine learning focused on creating new data, while large language models are used to process and generate human-like "natural content" Artificial Intelligence Models – They make predictions based on the inputs they are provided, and the output is “natural content” that resembles human-generated content.
Regarding the previously released "Big Language Model Top Ten Threat List", OWASP believes that it can help network security practitioners keep up with the rapidly developing AI technology, identify key threats and ensure that enterprises have basic security controls to Protect and support businesses using generative AI and large language models. However, OWASP believes that this list is not exhaustive and needs to be continuously improved based on the development of generative artificial intelligence.
OWASP divides AI security threats into the following five types:
5. Business case for artificial intelligence projects
Just like cloud computing, most enterprises do not actually develop a coherent strategic business case for the application of new technologies such as generative artificial intelligence and large language models. , it is easy to blindly follow the trend and fall into the hype. Without a sound business case, enterprise AI applications are likely to produce poor results and increase risks.
6. Governance
Without governance, companies cannot establish accountability mechanisms and clear goals for artificial intelligence. The OWASP checklist recommends that enterprises develop a RACI chart (responsibility allocation matrix) for artificial intelligence applications, record and allocate risk responsibilities and governance tasks, and establish enterprise-wide artificial intelligence policies and processes.
7. Legal
With the rapid development of artificial intelligence technology, its legal impact cannot be underestimated and may bring significant financial and reputational risks to enterprises.
Artificial intelligence legal affairs involves a series of activities, such as artificial intelligence product warranty, artificial intelligence end user license agreement (EULA), ownership of code developed using artificial intelligence tools, intellectual property risks and contractual indemnity clauses, etc. In short, make sure your legal team or experts understand the various supporting legal activities your company should undertake when using generative AI and large language models.
8. Supervision
Artificial intelligence regulatory regulations are also developing rapidly, such as the EU's Artificial Intelligence Act, and regulations in other countries and regions will soon be introduced. Businesses should understand their country’s AI compliance requirements, such as employee monitoring, and have a clear understanding of how their AI vendors store and delete data and regulate its use.
9. Use or implement large language model solutions
Using large language model solutions requires specific risks and controls to be considered. The OWASP checklist lists items such as access control, training pipeline security, mapping data workflows, and understanding existing or potential vulnerabilities in large language model models and supply chains. In addition, third-party audits, penetration testing, and even code reviews of vendors are required, both initially and on an ongoing basis.
10. Test, Evaluation, Verification, and Validation (TEVV)
The TEVV process is a process specifically recommended by NIST in its Artificial Intelligence Framework. This involves establishing continuous testing, evaluation, validation, and validation throughout the AI model lifecycle, as well as providing execution metrics on AI model functionality, safety, and reliability.
11. Model Cards and Risk Cards
To ethically deploy large language models, the OWASP checklist requires enterprises to use model and risk cards that can be used to enable users to understand and trust artificial intelligence systems , and publicly address potential negative consequences such as bias and privacy.
These cards can contain items such as model details, architecture, training data methods, and performance metrics. Considerations for responsible AI and concerns about fairness and transparency are also highlighted.
12RAG: Large Language Model Optimization
Retrieval Augmented Generation (RAG) is a method for optimizing the ability of large language models to retrieve relevant data from specific sources. It is one of the ways to optimize pre-trained models or retrain existing models based on new data to improve performance. OWASP recommends that enterprises implement RAG to maximize the value and effectiveness of large language models.
13.AI Red Team
Finally, the OWASP checklist highlights the importance of AI red teaming, which simulates adversarial attacks on AI systems to identify vulnerabilities and validate existing Control and defense. OWASP emphasizes that red teams should be an integral part of a comprehensive security solution with generative AI and large language models.
It is worth noting that enterprises also need to have a clear understanding of the red team services and system requirements and capabilities of external generative AI and large language model vendors to avoid violating policies or even getting into legal trouble.
The above is the detailed content of OWASP releases large language model network security and governance checklist. For more information, please follow other related articles on the PHP Chinese website!

Hot AI Tools

Undresser.AI Undress
AI-powered app for creating realistic nude photos

AI Clothes Remover
Online AI tool for removing clothes from photos.

Undress AI Tool
Undress images for free

Clothoff.io
AI clothes remover

Video Face Swap
Swap faces in any video effortlessly with our completely free AI face swap tool!

Hot Article

Hot Tools

Notepad++7.3.1
Easy-to-use and free code editor

SublimeText3 Chinese version
Chinese version, very easy to use

Zend Studio 13.0.1
Powerful PHP integrated development environment

Dreamweaver CS6
Visual web development tools

SublimeText3 Mac version
God-level code editing software (SublimeText3)

Hot Topics










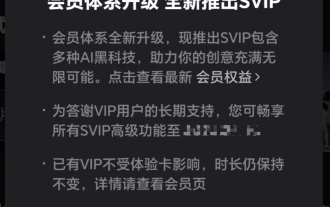
This site reported on June 27 that Jianying is a video editing software developed by FaceMeng Technology, a subsidiary of ByteDance. It relies on the Douyin platform and basically produces short video content for users of the platform. It is compatible with iOS, Android, and Windows. , MacOS and other operating systems. Jianying officially announced the upgrade of its membership system and launched a new SVIP, which includes a variety of AI black technologies, such as intelligent translation, intelligent highlighting, intelligent packaging, digital human synthesis, etc. In terms of price, the monthly fee for clipping SVIP is 79 yuan, the annual fee is 599 yuan (note on this site: equivalent to 49.9 yuan per month), the continuous monthly subscription is 59 yuan per month, and the continuous annual subscription is 499 yuan per year (equivalent to 41.6 yuan per month) . In addition, the cut official also stated that in order to improve the user experience, those who have subscribed to the original VIP
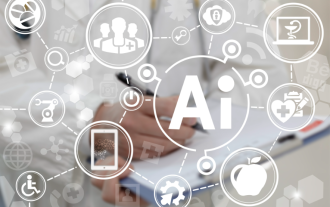
Translator | Bugatti Review | Chonglou This article describes how to use the GroqLPU inference engine to generate ultra-fast responses in JanAI and VSCode. Everyone is working on building better large language models (LLMs), such as Groq focusing on the infrastructure side of AI. Rapid response from these large models is key to ensuring that these large models respond more quickly. This tutorial will introduce the GroqLPU parsing engine and how to access it locally on your laptop using the API and JanAI. This article will also integrate it into VSCode to help us generate code, refactor code, enter documentation and generate test units. This article will create our own artificial intelligence programming assistant for free. Introduction to GroqLPU inference engine Groq
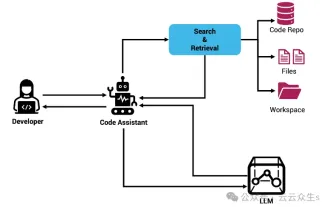
Improve developer productivity, efficiency, and accuracy by incorporating retrieval-enhanced generation and semantic memory into AI coding assistants. Translated from EnhancingAICodingAssistantswithContextUsingRAGandSEM-RAG, author JanakiramMSV. While basic AI programming assistants are naturally helpful, they often fail to provide the most relevant and correct code suggestions because they rely on a general understanding of the software language and the most common patterns of writing software. The code generated by these coding assistants is suitable for solving the problems they are responsible for solving, but often does not conform to the coding standards, conventions and styles of the individual teams. This often results in suggestions that need to be modified or refined in order for the code to be accepted into the application

To learn more about AIGC, please visit: 51CTOAI.x Community https://www.51cto.com/aigc/Translator|Jingyan Reviewer|Chonglou is different from the traditional question bank that can be seen everywhere on the Internet. These questions It requires thinking outside the box. Large Language Models (LLMs) are increasingly important in the fields of data science, generative artificial intelligence (GenAI), and artificial intelligence. These complex algorithms enhance human skills and drive efficiency and innovation in many industries, becoming the key for companies to remain competitive. LLM has a wide range of applications. It can be used in fields such as natural language processing, text generation, speech recognition and recommendation systems. By learning from large amounts of data, LLM is able to generate text
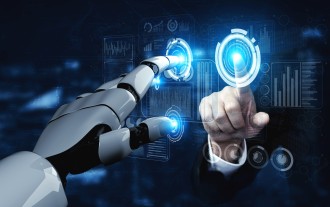
Large Language Models (LLMs) are trained on huge text databases, where they acquire large amounts of real-world knowledge. This knowledge is embedded into their parameters and can then be used when needed. The knowledge of these models is "reified" at the end of training. At the end of pre-training, the model actually stops learning. Align or fine-tune the model to learn how to leverage this knowledge and respond more naturally to user questions. But sometimes model knowledge is not enough, and although the model can access external content through RAG, it is considered beneficial to adapt the model to new domains through fine-tuning. This fine-tuning is performed using input from human annotators or other LLM creations, where the model encounters additional real-world knowledge and integrates it
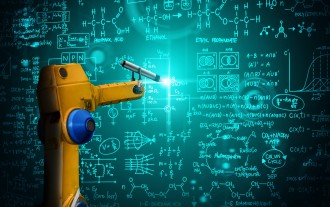
Machine learning is an important branch of artificial intelligence that gives computers the ability to learn from data and improve their capabilities without being explicitly programmed. Machine learning has a wide range of applications in various fields, from image recognition and natural language processing to recommendation systems and fraud detection, and it is changing the way we live. There are many different methods and theories in the field of machine learning, among which the five most influential methods are called the "Five Schools of Machine Learning". The five major schools are the symbolic school, the connectionist school, the evolutionary school, the Bayesian school and the analogy school. 1. Symbolism, also known as symbolism, emphasizes the use of symbols for logical reasoning and expression of knowledge. This school of thought believes that learning is a process of reverse deduction, through existing
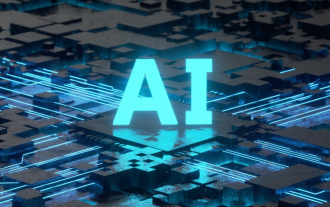
Editor |ScienceAI Question Answering (QA) data set plays a vital role in promoting natural language processing (NLP) research. High-quality QA data sets can not only be used to fine-tune models, but also effectively evaluate the capabilities of large language models (LLM), especially the ability to understand and reason about scientific knowledge. Although there are currently many scientific QA data sets covering medicine, chemistry, biology and other fields, these data sets still have some shortcomings. First, the data form is relatively simple, most of which are multiple-choice questions. They are easy to evaluate, but limit the model's answer selection range and cannot fully test the model's ability to answer scientific questions. In contrast, open-ended Q&A
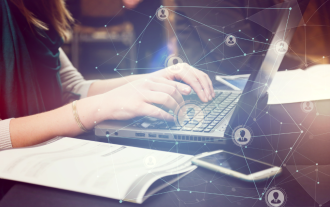
This article will open source the results of "Local Deployment of Large Language Models in OpenHarmony" demonstrated at the 2nd OpenHarmony Technology Conference. Open source address: https://gitee.com/openharmony-sig/tpc_c_cplusplus/blob/master/thirdparty/InferLLM/docs/ hap_integrate.md. The implementation ideas and steps are to transplant the lightweight LLM model inference framework InferLLM to the OpenHarmony standard system, and compile a binary product that can run on OpenHarmony. InferLLM is a simple and efficient L
