


Go language in artificial intelligence: from machine learning to deep learning
Go language is widely used in artificial intelligence, including machine learning and deep learning. The article introduces the libraries of Go language in machine learning, such as gonum and scikit-go, and shows a practical case of using Go language to train a logistic regression model. In addition, the Go language provides deep learning frameworks such as TensorFlow Lite and xgboost, but performance and scalability need to be considered. In summary, the Go language is powerful, efficient, and easy to use, making it suitable for artificial intelligence projects, including machine learning and deep learning.
Go language in artificial intelligence: from machine learning to deep learning
Go language has gained widespread attention in the field of artificial intelligence (AI) in recent years. Because it stands out with its features such as concurrency, strong typing, and portability. This article will outline the application of Go language in machine learning and deep learning, and introduce a practical case.
Machine Learning
Machine learning is a field of AI that involves training computers to automatically learn from data without explicit programming instructions. Go language provides many machine learning libraries, including:
- [gonum](https://pkg.go.dev/gonum.org/v1/gonum): Provides mathematical and scientific computing Advanced numerical package.
- [scikit-go](https://github.com/scikit-go/scikit-go): Inspired by the Python scikit-learn library, it provides various machine learning algorithms.
- [goml](https://github.com/skelterjohn/goml): Contains a set of commonly used machine learning algorithms, such as logistic regression, support vector machines and decision trees.
Practical case: Using Go language to train a logistic regression model
Now, let us use Go language to build a simple logistic regression model to predict whether a customer will buy a product.
package main import ( "gonum.org/v1/gonum/stat/distuv" "math" "time" ) func main() { // 准备数据 features := [][]float64{{0.1, 0.2}, {0.3, 0.4}, {0.5, 0.6}, {0.7, 0.8}} labels := []float64{0, 1, 1, 0} // 训练逻辑回归模型 w := []float64{0.1, 0.2} b := 0.3 lr := 0.01 for i := 0; i < 100; i++ { //计算预测值 var pred []float64 for _, feature := range features { p := logistic(w[0]*feature[0] + w[1]*feature[1] + b) pred = append(pred, p) } // 更新权重和偏差 for j := 0; j < len(w); j++ { var grad float64 for k := 0; k < len(features); k++ { grad += (pred[k] - labels[k]) * features[k][j] } w[j] -= lr * grad } b -= lr * math.Sum((pred, labels)) / float64(len(features)) } // 预测新数据 feature := []float64{0.4, 0.5} p := logistic(w[0]*feature[0] + w[1]*feature[1] + b) if p >= 0.5 { fmt.Println("预测为 1") } else { fmt.Println("预测为 0") } } //逻辑函数 func logistic(x float64) float64 { return 1 / (1 + math.Exp(-x)) }
Running this code will output the prediction of the new feature, i.e. buy or not buy.
Deep Learning
Deep learning is another area of AI that uses multi-layered neural networks to learn complex patterns. The Go language also provides many deep learning frameworks, including:
- [TensorFlow Lite](https://www.tensorflow.org/lite/go): TensorFlow’s lightweight mobile and embedded version Deployment version.
- [ONNX Runtime](https://github.com/microsoft/onnxruntime): A cross-platform inference engine used to deploy trained ONNX models.
- [xgboost](https://github.com/dmlc/xgboost-go): Provides a library for implementing gradient boosting machines.
Deep learning models usually require a large amount of data and computing resources for training, so performance and scalability need to be considered when using the Go language for deep learning.
Conclusion
The Go language provides developers with a set of valuable tools and libraries in the field of artificial intelligence. It's powerful, efficient, and easy to use, making it ideal for machine learning and deep learning projects. As artificial intelligence technology continues to develop, the Go language is likely to continue to play an important role in this field.
The above is the detailed content of Go language in artificial intelligence: from machine learning to deep learning. For more information, please follow other related articles on the PHP Chinese website!

Hot AI Tools

Undresser.AI Undress
AI-powered app for creating realistic nude photos

AI Clothes Remover
Online AI tool for removing clothes from photos.

Undress AI Tool
Undress images for free

Clothoff.io
AI clothes remover

Video Face Swap
Swap faces in any video effortlessly with our completely free AI face swap tool!

Hot Article

Hot Tools

Notepad++7.3.1
Easy-to-use and free code editor

SublimeText3 Chinese version
Chinese version, very easy to use

Zend Studio 13.0.1
Powerful PHP integrated development environment

Dreamweaver CS6
Visual web development tools

SublimeText3 Mac version
God-level code editing software (SublimeText3)

Hot Topics










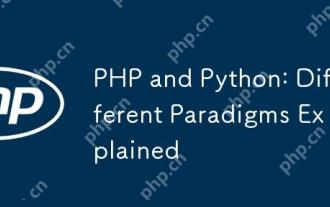
PHP is mainly procedural programming, but also supports object-oriented programming (OOP); Python supports a variety of paradigms, including OOP, functional and procedural programming. PHP is suitable for web development, and Python is suitable for a variety of applications such as data analysis and machine learning.
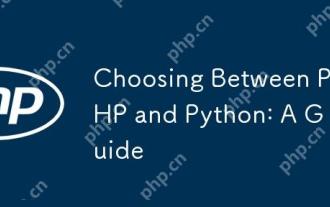
PHP is suitable for web development and rapid prototyping, and Python is suitable for data science and machine learning. 1.PHP is used for dynamic web development, with simple syntax and suitable for rapid development. 2. Python has concise syntax, is suitable for multiple fields, and has a strong library ecosystem.
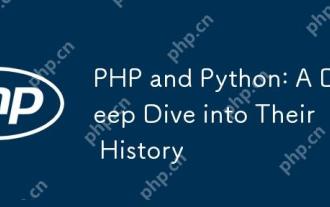
PHP originated in 1994 and was developed by RasmusLerdorf. It was originally used to track website visitors and gradually evolved into a server-side scripting language and was widely used in web development. Python was developed by Guidovan Rossum in the late 1980s and was first released in 1991. It emphasizes code readability and simplicity, and is suitable for scientific computing, data analysis and other fields.
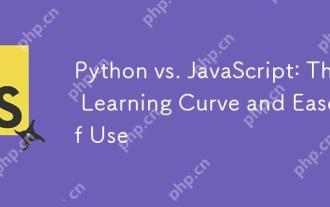
Python is more suitable for beginners, with a smooth learning curve and concise syntax; JavaScript is suitable for front-end development, with a steep learning curve and flexible syntax. 1. Python syntax is intuitive and suitable for data science and back-end development. 2. JavaScript is flexible and widely used in front-end and server-side programming.
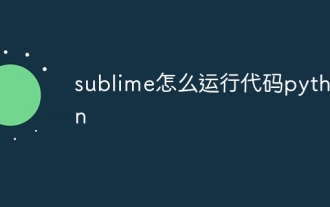
To run Python code in Sublime Text, you need to install the Python plug-in first, then create a .py file and write the code, and finally press Ctrl B to run the code, and the output will be displayed in the console.
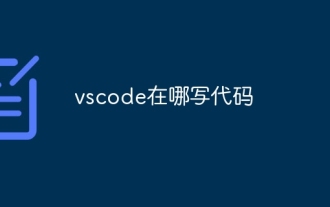
Writing code in Visual Studio Code (VSCode) is simple and easy to use. Just install VSCode, create a project, select a language, create a file, write code, save and run it. The advantages of VSCode include cross-platform, free and open source, powerful features, rich extensions, and lightweight and fast.
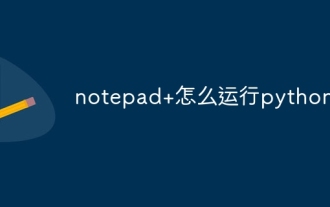
Running Python code in Notepad requires the Python executable and NppExec plug-in to be installed. After installing Python and adding PATH to it, configure the command "python" and the parameter "{CURRENT_DIRECTORY}{FILE_NAME}" in the NppExec plug-in to run Python code in Notepad through the shortcut key "F6".
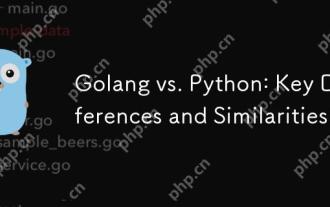
Golang and Python each have their own advantages: Golang is suitable for high performance and concurrent programming, while Python is suitable for data science and web development. Golang is known for its concurrency model and efficient performance, while Python is known for its concise syntax and rich library ecosystem.
