How machine learning improves business intelligence
Machine learning (ML) plays a key role in the continued development of business intelligence (BI). With the advent of ML, enterprises are moving beyond traditional analytics to adopt more sophisticated methods to interpret massive data sets. This article explores the revolution brought about by ML, focusing on the significant shift from pure data analysis to predictive insights and decision-making strategies.
Integration of ML in BI
Integrating ML into BI tools is not just an upgrade, it is a revolution. Traditional BI systems focus on descriptive analytics, which involves analyzing historical data to understand past behavior. However, ML takes this further by integrating predictive analytics, leveraging already existing data to predict future trends. This step allows the company to more accurately predict future trends, customer behavior and potential risks. This advancement allows companies to more accurately predict future trends, customer behavior and potential risks. This advancement allows companies to more accurately predict future trends, customer behavior and potential risks. However, it also increases the company's ability to predict unknown future trends and customer behavior. This step allows the company to more accurately predict future trends, customer behavior and potential risks, leveraging previous data to predict
enhanced data processing
an important advantage Yes, one of the big advantages that ML brings is its ability to process and scrutinize data like never before. Unlike traditional methods, ML algorithms are good at quickly browsing large amounts of data, discovering patterns and connections that are beyond the scope of manual analysis. This enhanced ability to process data quickly allows businesses to react instantly to new trends and insights.
Predictive analysis and forecasting
Change the function of BI from simply reporting events that have occurred to predicting events that will occur next. Using historical data, ML models are able to predict upcoming market trends, consumer demand, and possible disruptions in the supply chain. These forecasts enable businesses to proactively adjust strategies, optimize operations and mitigate risks before they materialize.
Personalization at scale
In the current market, customization plays a vital role in ensuring customer satisfaction and loyalty. Using machine learning, businesses can sift through customer data and behavioral trends to create a personalized experience for each customer. From customized product recommendations to tailored marketing messages, businesses using machine learning-driven BI tools can engage customers in more meaningful and effective ways.
Automation of decision-making processes
Machine learning can automate complex decision-making processes. By training models based on historical data, businesses can delegate day-to-day decisions to algorithms, freeing up human resources to perform more strategic tasks. This automation extends to various fields, including finance and supply chain management. This automation extends to various fields, including finance and supply chain management. Having human resources in finance and supply chain management can perform more strategic tasks. This automation extends to various fields, including finance and supply chain management. This automated
Challenges and considerations
While incorporating machine learning (ML) into business intelligence (BI) systems is transformative, it also comes with Series of challenges and considerations. Enterprises need to deal with it carefully. These challenges stem from the technical complexity of machine learning, but also from the operational realities of integrating advanced analytics into business processes.
Data Privacy and Security
To protect as larger and larger data sets are accumulated and analyzed, the need to maintain data privacy and security becomes even more important. Deploying ML within a BI framework requires access to detailed and often confidential information, which increases the need for strong data protection measures and compliance with regulatory standards such as GDPR, CCPA and others. Protecting the privacy, accuracy, and accessibility of data in the context of ML usage becomes a huge hurdle. Businesses must adopt strict data governance practices and employ advanced security controls to protect data from breaches and unauthorized access.
Data quality and quantity
The reliability of machine learning predictions depends on the quality and quantity of data fed into the algorithm. Incorrect, incomplete or distorted data can lead to misleading conclusions and wrong decisions. Ensuring data quality involves cleaning, validating, and enriching data, processes that can require significant resources. In addition to this, machine learning models often require large data sets for training to achieve high accuracy, which poses a challenge for enterprises to collect enough relevant data.
Skilled Talent Shortage
Successful integration of ML into BI systems requires employees with unique skill sets, including data science, ML algorithms, and business domain knowledge Expertise. However, there is a clear shortage of professionals with these skill sets, making it difficult for enterprises to find and retain the talent they need to drive their ML initiatives. A shortage of skilled professionals will slow down the integration of ML and BI, thereby limiting the full benefits of them.
倫理的およびバイアスに関する考慮事項
ML モデルは、トレーニング データ内の既存のバイアスを誤って強化または悪化させ、偏った結果や不公平な結果をもたらす可能性があります。たとえば、ML を利用し、過去の採用記録を使用してトレーニングされた採用ツールでは、性別や人種に関連したバイアスが表示される可能性があります。企業にとって、バイアス補正などの方法を使用したり、モデルのトレーニングにさまざまなデータセットを活用したりして、ML アルゴリズムのバイアスを積極的に検出して対処することが重要です。倫理的考慮事項は、特に金融やヘルスケアなどの決定が大きな影響を与える業界において、機械学習の決定の透明性と説明可能性にも及びます。
既存システムとの統合
ML モデルを既存の BI システムおよびワークフローに統合することは、技術的に難しい場合があります。
互換性の問題、独立したデータ ストレージ、およびオンザフライ データ処理パイプラインの要件は、頻繁に直面する課題です。企業は統合プロセスを慎重に計画する必要があり、多くの場合、IT インフラストラクチャの大幅なアップグレードや、ML 機能をシームレスに統合できる新しいツールやプラットフォームの導入が必要になります。
継続的なモニタリングとメンテナンス
ML モデルは、精度と有効性を維持するために継続的な監視とメンテナンスを必要とし、計画を単に実装して放置できるソリューションではありません。基礎となるデータ パターン、市場状況、ビジネス目標の変化により、モデルの再トレーニングや調整が必要になる場合があります。この継続的な監視と更新の要件により、BI での ML の使用が複雑になり、専用のリソースと注意が必要になります。
将来の見通し
技術の進歩によりその可能性が広がり続けるため、ビジネス インテリジェンスにおける機械学習の将来は楽観的です。次のフロンティアには、自然言語処理 (NLP) を統合してより直観的なデータ クエリを可能にすること、ディープ ラーニングを使用してより複雑な予測モデルを可能にすることが含まれます。これらのテクノロジーが進化するにつれて、機械学習がビジネス インテリジェンスを強化し、ビジネスの成長を促進する可能性がますます明らかになってきています。
概要
機械学習とビジネス インテリジェンスの組み合わせは、企業がデータを使用して意思決定を行う方法におけるパラダイム シフトを表しています。機械学習は、予測分析、パーソナライゼーション、自動化を可能にすることでビジネス インテリジェンス環境に革命をもたらし、前例のない洞察と機能を企業に提供します。課題はあるものの、ビジネス インテリジェンスに機械学習を導入することで得られる潜在的な利益は非常に大きく、将来のデータドリブンな意思決定は、これまでよりも正確で効率的で影響力のあるものになるでしょう。企業がデジタル時代の複雑さに取り組み続けるにつれて、ビジネス インテリジェンスにおける機械学習の役割は間違いなく成長し続け、ビジネス インテリジェンスの将来を大きく形作っていきます。
The above is the detailed content of How machine learning improves business intelligence. For more information, please follow other related articles on the PHP Chinese website!

Hot AI Tools

Undresser.AI Undress
AI-powered app for creating realistic nude photos

AI Clothes Remover
Online AI tool for removing clothes from photos.

Undress AI Tool
Undress images for free

Clothoff.io
AI clothes remover

Video Face Swap
Swap faces in any video effortlessly with our completely free AI face swap tool!

Hot Article

Hot Tools

Notepad++7.3.1
Easy-to-use and free code editor

SublimeText3 Chinese version
Chinese version, very easy to use

Zend Studio 13.0.1
Powerful PHP integrated development environment

Dreamweaver CS6
Visual web development tools

SublimeText3 Mac version
God-level code editing software (SublimeText3)

Hot Topics










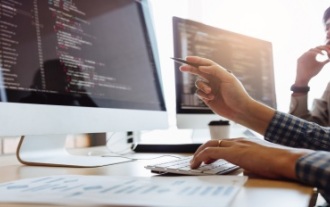
Image annotation is the process of associating labels or descriptive information with images to give deeper meaning and explanation to the image content. This process is critical to machine learning, which helps train vision models to more accurately identify individual elements in images. By adding annotations to images, the computer can understand the semantics and context behind the images, thereby improving the ability to understand and analyze the image content. Image annotation has a wide range of applications, covering many fields, such as computer vision, natural language processing, and graph vision models. It has a wide range of applications, such as assisting vehicles in identifying obstacles on the road, and helping in the detection and diagnosis of diseases through medical image recognition. . This article mainly recommends some better open source and free image annotation tools. 1.Makesens
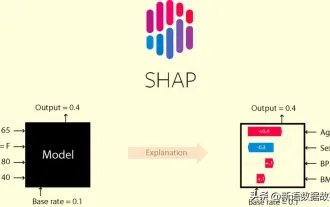
In the fields of machine learning and data science, model interpretability has always been a focus of researchers and practitioners. With the widespread application of complex models such as deep learning and ensemble methods, understanding the model's decision-making process has become particularly important. Explainable AI|XAI helps build trust and confidence in machine learning models by increasing the transparency of the model. Improving model transparency can be achieved through methods such as the widespread use of multiple complex models, as well as the decision-making processes used to explain the models. These methods include feature importance analysis, model prediction interval estimation, local interpretability algorithms, etc. Feature importance analysis can explain the decision-making process of a model by evaluating the degree of influence of the model on the input features. Model prediction interval estimate
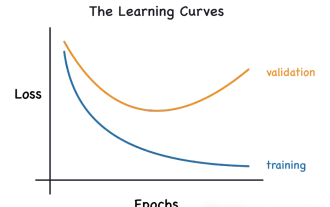
This article will introduce how to effectively identify overfitting and underfitting in machine learning models through learning curves. Underfitting and overfitting 1. Overfitting If a model is overtrained on the data so that it learns noise from it, then the model is said to be overfitting. An overfitted model learns every example so perfectly that it will misclassify an unseen/new example. For an overfitted model, we will get a perfect/near-perfect training set score and a terrible validation set/test score. Slightly modified: "Cause of overfitting: Use a complex model to solve a simple problem and extract noise from the data. Because a small data set as a training set may not represent the correct representation of all data." 2. Underfitting Heru
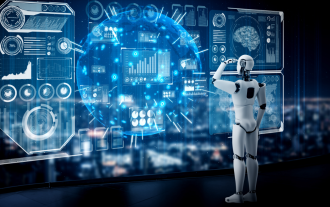
In the 1950s, artificial intelligence (AI) was born. That's when researchers discovered that machines could perform human-like tasks, such as thinking. Later, in the 1960s, the U.S. Department of Defense funded artificial intelligence and established laboratories for further development. Researchers are finding applications for artificial intelligence in many areas, such as space exploration and survival in extreme environments. Space exploration is the study of the universe, which covers the entire universe beyond the earth. Space is classified as an extreme environment because its conditions are different from those on Earth. To survive in space, many factors must be considered and precautions must be taken. Scientists and researchers believe that exploring space and understanding the current state of everything can help understand how the universe works and prepare for potential environmental crises
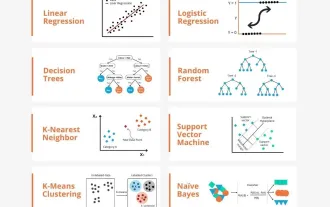
In layman’s terms, a machine learning model is a mathematical function that maps input data to a predicted output. More specifically, a machine learning model is a mathematical function that adjusts model parameters by learning from training data to minimize the error between the predicted output and the true label. There are many models in machine learning, such as logistic regression models, decision tree models, support vector machine models, etc. Each model has its applicable data types and problem types. At the same time, there are many commonalities between different models, or there is a hidden path for model evolution. Taking the connectionist perceptron as an example, by increasing the number of hidden layers of the perceptron, we can transform it into a deep neural network. If a kernel function is added to the perceptron, it can be converted into an SVM. this one
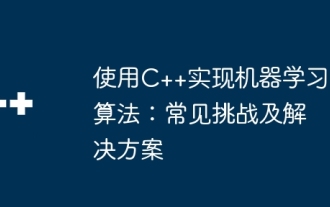
Common challenges faced by machine learning algorithms in C++ include memory management, multi-threading, performance optimization, and maintainability. Solutions include using smart pointers, modern threading libraries, SIMD instructions and third-party libraries, as well as following coding style guidelines and using automation tools. Practical cases show how to use the Eigen library to implement linear regression algorithms, effectively manage memory and use high-performance matrix operations.
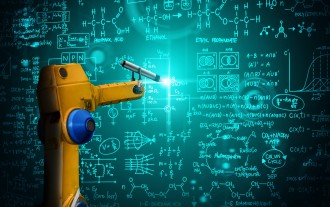
Machine learning is an important branch of artificial intelligence that gives computers the ability to learn from data and improve their capabilities without being explicitly programmed. Machine learning has a wide range of applications in various fields, from image recognition and natural language processing to recommendation systems and fraud detection, and it is changing the way we live. There are many different methods and theories in the field of machine learning, among which the five most influential methods are called the "Five Schools of Machine Learning". The five major schools are the symbolic school, the connectionist school, the evolutionary school, the Bayesian school and the analogy school. 1. Symbolism, also known as symbolism, emphasizes the use of symbols for logical reasoning and expression of knowledge. This school of thought believes that learning is a process of reverse deduction, through existing
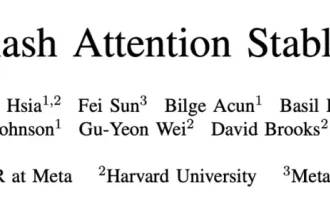
MetaFAIR teamed up with Harvard to provide a new research framework for optimizing the data bias generated when large-scale machine learning is performed. It is known that the training of large language models often takes months and uses hundreds or even thousands of GPUs. Taking the LLaMA270B model as an example, its training requires a total of 1,720,320 GPU hours. Training large models presents unique systemic challenges due to the scale and complexity of these workloads. Recently, many institutions have reported instability in the training process when training SOTA generative AI models. They usually appear in the form of loss spikes. For example, Google's PaLM model experienced up to 20 loss spikes during the training process. Numerical bias is the root cause of this training inaccuracy,
