


StreamingT2V, a long video generator of two minutes and 1200 frames, is here, and the code will be open source
Wide shot of battlefield, stormtroopers running...
prompt: Wide shot of battlefield, stormtroopers running...
This 2-minute video with 1,200 frames was generated by a text-to-video model. Although the traces of AI are still obvious, the characters and scenes show quite good consistency.
How is this done? You should know that although the generation quality and text alignment quality of Vincent video technology have been quite good in recent years, most existing methods focus on generating short videos (usually 16 or 24 frames in length). However, existing methods that work for short videos often fail to work with long videos (≥ 64 frames).
Even generating short sequences often requires expensive training, such as training steps exceeding 260K and batch sizes exceeding 4500. If you do not train on longer videos and use a short video generator to produce long videos, the resulting long videos are often of poor quality. The existing autoregressive method (generating a new short video by using the last few frames of the short video, and then synthesizing the long video) also has some problems such as inconsistent scene switching.
In order to make up for the shortcomings of existing methods, Picsart AI Research and other institutions jointly proposed a new Vincent video method: StreamingT2V. This method uses autoregressive technology and combines it with a long short-term memory module, which enables it to generate long videos with strong temporal coherence.
- Paper title: StreamingT2V: Consistent, Dynamic, and Extendable Long Video Generation from Text
- Paper address: https://arxiv.org/abs/2403.14773
- Project address: https://streamingt2v.github.io/
The following is a 600-frame 1-minute video generation result. You can see that bees and flowers have excellent consistency:
Therefore, the team put forward the conditions Attention Module (CAM). CAM uses its attention mechanism to effectively integrate information from previous frames to generate new frames, and can freely handle motion in new frames without being restricted by the structure or shape of previous frames.
In order to solve the problem of appearance changes of people and objects in the generated video, the team also proposed the Appearance Preservation Module (APM): it can start from an initial image (anchor frame) Extract appearance information of objects or global scenes and use this information to regulate the video generation process for all video patches.
To further improve the quality and resolution of long video generation, the team improved a video enhancement model for the autoregressive generation task. To do this, the team selected a high-resolution Vincent video model and used the SDEdit method to improve the quality of 24 consecutive video blocks (with 8 overlapping frames).
To smooth the video block enhancement transition, they also designed a random blending method that blends overlapping enhanced video blocks in a seamless manner.
Method
First, generate a 5 second long 256 × 256 resolution video (16fps), then enhance it to higher resolution (720 × 720). Figure 2 shows its complete workflow.
The long video generation part consists of the Initialization Stage and the Streaming T2V Stage.
Among them, the initialization stage is to use a pre-trained Vincent video model (for example, you can use Modelscope) to generate the first 16-frame video block; while the streaming Vincent video stage is Generate new content for subsequent frames in an autoregressive manner.
For the autoregressive process (see Figure 3), the team’s newly proposed CAM can utilize the short-term information of the last 8 frames of the previous video block to achieve seamless switching between blocks. In addition, they will also use the newly proposed APM module to extract long-term information of a fixed anchor frame, so that the autoregressive process can robustly cope with changes in things and scene details during the generation process.
After generating long videos (80, 240, 600, 1200 or more frames), they then improve them through the Streaming Refinement Stage Video quality. This process uses a high-resolution Vison short video model (e.g., MS-Vid2Vid-XL) in an autoregressive manner, coupled with a newly proposed stochastic mixing method for seamless video block processing. Furthermore, the latter step does not require additional training, which makes this method less computationally expensive.
Conditional attention module
First, use the pre-trained text ( Short) video model is denoted as Video-LDM. The attention module (CAM) consists of a feature extractor and a feature injector injected into Video-LDM UNet.
The feature extractor uses a frame-by-frame image encoder, followed by the same encoder layer used by Video-LDM UNet until the middle layer (and initialized by the weight of UNet ).
For feature injection, the design here is to let each long-range jump connection in UNet focus on the corresponding features generated by CAM through cross attention.
Appearance Preservation Module
The APM module can fix the information in the anchor frame by using to integrate long-term memory into the video generation process. This helps maintain scene and object characteristics during video patch generation.
In order to allow APM to balance the processing of guidance information given by anchor frames and text instructions, the team has made two improvements: (1) Combine the CLIP image token of the anchor frame with the text The CLIP text tokens of the instructions are mixed; (2) A weight is introduced for each cross-attention layer to use cross-attention.
Autoregressive Video Enhancement
To autoregressively enhance the generated video block of 24 frames, here we use High-resolution (1280x720) Vincent (short) video model (Refiner Video-LDM, see Figure 3). This process is done by first adding a large amount of noise to the input video block, and then using this Vincent video diffusion model to perform denoising processing.
However, this method is not enough to solve the problem of transition mismatch between video blocks.
To this end, the team’s solution is a random hybrid approach. Please refer to the original paper for specific details.
Experiment
In the experiment, the evaluation metrics used by the team include: SCuts score for evaluating temporal consistency, Motion-aware twist error (MAWE) for amount of motion and twist error, CLIP text-image similarity score (CLIP) for evaluating text alignment quality, aesthetic score (AE).
Ablation Study
To evaluate the effectiveness of various new components, the team Ablation studies were performed on 75 prompts randomly sampled from the validation set.
CAM for conditional processing: CAM helps the model generate more consistent videos, with SCuts scores 88% lower than other baseline models in comparison.
Long-term memory: Figure 6 shows that long-term memory can greatly help maintain the stability of the characteristics of objects and scenes during the autoregressive generation process.
On a quantitative evaluation metric (person re-identification score), APM achieved a 20% improvement.
Random mixing for video enhancement: Compared with the other two benchmarks, random mixing can bring significant quality improvements, which can also be seen from Figure 4: StreamingT2V can get Smoother transitions.
##StreamingT2V compared to the baseline model
The The team compared the integration of the above-mentioned improved StreamingT2V with multiple models through quantitative and qualitative evaluations, including the image-to-video method I2VGen-XL, SVD, DynamiCrafter-XL, SEINE using the autoregressive method, the video-to-video method SparseControl, and the text-to-long video MethodFreeNoise.
Quantitative evaluation: As can be seen from Table 8, quantitative evaluation on the test set shows that StreamingT2V performs best in terms of seamless video block transition and motion consistency. The MAWE score of the new method is also significantly better than all other methods - even more than 50% lower than the second-best SEINE. Similar behavior is seen in SCuts scores.
In addition, StreamingT2V is only slightly inferior to SparseCtrl in terms of the single-frame quality of the generated video. This shows that this new method is able to generate high-quality long videos with better temporal consistency and motion dynamics than other comparison methods.
Qualitative evaluation: The following figure shows the comparison of the effects of StreamingT2V with other methods. It can be seen that the new method can maintain better consistency while ensuring the dynamic effect of the video.
For more research details, please refer to the original paper.
The above is the detailed content of StreamingT2V, a long video generator of two minutes and 1200 frames, is here, and the code will be open source. For more information, please follow other related articles on the PHP Chinese website!

Hot AI Tools

Undresser.AI Undress
AI-powered app for creating realistic nude photos

AI Clothes Remover
Online AI tool for removing clothes from photos.

Undress AI Tool
Undress images for free

Clothoff.io
AI clothes remover

Video Face Swap
Swap faces in any video effortlessly with our completely free AI face swap tool!

Hot Article

Hot Tools

Notepad++7.3.1
Easy-to-use and free code editor

SublimeText3 Chinese version
Chinese version, very easy to use

Zend Studio 13.0.1
Powerful PHP integrated development environment

Dreamweaver CS6
Visual web development tools

SublimeText3 Mac version
God-level code editing software (SublimeText3)

Hot Topics










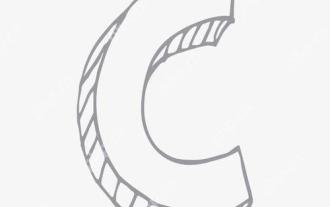
DMA in C refers to DirectMemoryAccess, a direct memory access technology, allowing hardware devices to directly transmit data to memory without CPU intervention. 1) DMA operation is highly dependent on hardware devices and drivers, and the implementation method varies from system to system. 2) Direct access to memory may bring security risks, and the correctness and security of the code must be ensured. 3) DMA can improve performance, but improper use may lead to degradation of system performance. Through practice and learning, we can master the skills of using DMA and maximize its effectiveness in scenarios such as high-speed data transmission and real-time signal processing.
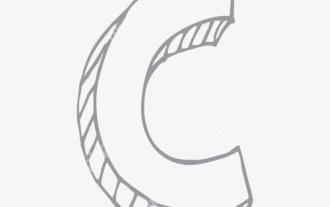
Using the chrono library in C can allow you to control time and time intervals more accurately. Let's explore the charm of this library. C's chrono library is part of the standard library, which provides a modern way to deal with time and time intervals. For programmers who have suffered from time.h and ctime, chrono is undoubtedly a boon. It not only improves the readability and maintainability of the code, but also provides higher accuracy and flexibility. Let's start with the basics. The chrono library mainly includes the following key components: std::chrono::system_clock: represents the system clock, used to obtain the current time. std::chron
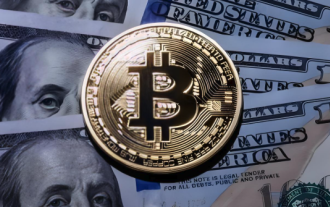
The built-in quantization tools on the exchange include: 1. Binance: Provides Binance Futures quantitative module, low handling fees, and supports AI-assisted transactions. 2. OKX (Ouyi): Supports multi-account management and intelligent order routing, and provides institutional-level risk control. The independent quantitative strategy platforms include: 3. 3Commas: drag-and-drop strategy generator, suitable for multi-platform hedging arbitrage. 4. Quadency: Professional-level algorithm strategy library, supporting customized risk thresholds. 5. Pionex: Built-in 16 preset strategy, low transaction fee. Vertical domain tools include: 6. Cryptohopper: cloud-based quantitative platform, supporting 150 technical indicators. 7. Bitsgap:
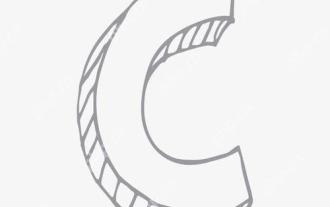
Handling high DPI display in C can be achieved through the following steps: 1) Understand DPI and scaling, use the operating system API to obtain DPI information and adjust the graphics output; 2) Handle cross-platform compatibility, use cross-platform graphics libraries such as SDL or Qt; 3) Perform performance optimization, improve performance through cache, hardware acceleration, and dynamic adjustment of the details level; 4) Solve common problems, such as blurred text and interface elements are too small, and solve by correctly applying DPI scaling.
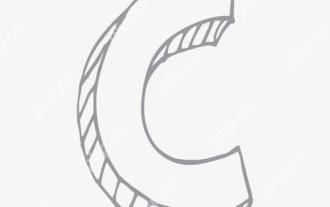
C performs well in real-time operating system (RTOS) programming, providing efficient execution efficiency and precise time management. 1) C Meet the needs of RTOS through direct operation of hardware resources and efficient memory management. 2) Using object-oriented features, C can design a flexible task scheduling system. 3) C supports efficient interrupt processing, but dynamic memory allocation and exception processing must be avoided to ensure real-time. 4) Template programming and inline functions help in performance optimization. 5) In practical applications, C can be used to implement an efficient logging system.
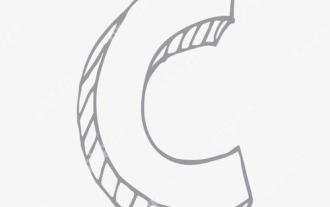
The main steps and precautions for using string streams in C are as follows: 1. Create an output string stream and convert data, such as converting integers into strings. 2. Apply to serialization of complex data structures, such as converting vector into strings. 3. Pay attention to performance issues and avoid frequent use of string streams when processing large amounts of data. You can consider using the append method of std::string. 4. Pay attention to memory management and avoid frequent creation and destruction of string stream objects. You can reuse or use std::stringstream.
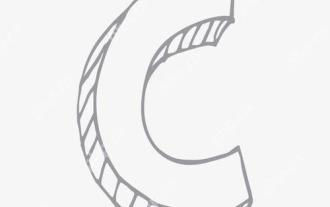
Measuring thread performance in C can use the timing tools, performance analysis tools, and custom timers in the standard library. 1. Use the library to measure execution time. 2. Use gprof for performance analysis. The steps include adding the -pg option during compilation, running the program to generate a gmon.out file, and generating a performance report. 3. Use Valgrind's Callgrind module to perform more detailed analysis. The steps include running the program to generate the callgrind.out file and viewing the results using kcachegrind. 4. Custom timers can flexibly measure the execution time of a specific code segment. These methods help to fully understand thread performance and optimize code.
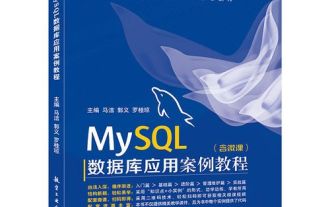
Efficient methods for batch inserting data in MySQL include: 1. Using INSERTINTO...VALUES syntax, 2. Using LOADDATAINFILE command, 3. Using transaction processing, 4. Adjust batch size, 5. Disable indexing, 6. Using INSERTIGNORE or INSERT...ONDUPLICATEKEYUPDATE, these methods can significantly improve database operation efficiency.
