


Performance Measurement of Python Natural Language Processing: Assessing Model Accuracy and Efficiency
python Performance measurements of Natural Language Processing (NLP) models in
are useful for evaluating the effectiveness and Efficiency is crucial. The following are the main metrics used to evaluate the accuracy and efficiency of NLP models:
- Accuracy index:
- Precision: Measures the proportion of samples predicted as positive by the model that are actually positive.
- Recall (Recall): Measures the proportion of all actual positive samples predicted by the model that are predicted to be positive by the model.
- F1 score: The weighted average of precision and recall, providing a measure of the overall accuracy of the model.
- Accuracy: Measures the proportion of correct predictions among all samples predicted by the model.
Shows the actual and predicted values predicted by the model and is used to identify false positives and false negatives.
- Efficiency indicators:
- Training time: The time required to train the model.
- Prediction time: The time required to predict new data.
- Memory usage: The amount of memory required to train and predict the model. Complexity: Measures the computational complexity of the model
.
assessment method:
Performance evaluation of NLP models often involves the use of cross-validation to ensure the reliability of the results. Cross-validation divides the data set into multiple subsets, each subset in turn is used as atest set, while the remaining data is used as a training set. The model is trained and evaluated on each subset, and then the average performance metric is calculated across all subsets.
Optimize performance:
In order to- optimize the performance of the
- NLP model, the following aspects can be adjusted: Hyperparameters: Parameters of the model training algorithm, such as
- learning rate and regularization terms.
- Feature Engineering: Preprocess data to improve model performance.
- Model Architecture: Select the model type and configuration appropriate for the specific task.
Use techniques to increase the amount and diversity of training data.
Tools and Libraries: Python
There are many- tools
- and libraries available for performance measurement of NLP models, including: scikit-learn: A
- machine learning library that provides evaluation metrics and cross-validation functions. TensorFlow: A framework for training and evaluating
- deep learning models. Keras: Advanced Neural Networks api based on
- Tensorflow.
Provides pre-trained NLP models and metrics for their evaluation.
Factors affecting performance:
### ###Factors that affect NLP model performance include: ###- Data quality: The quality and size of the training and test data sets.
- Complexity of the model: The size and depth of the model architecture .
- Computing resources: Computing power used to train and predict models.
- Task type: The type and difficulty of the NLP task.
Best Practices:
Best practices when evaluating NLP models include:
- Use multiple accuracy metrics: Don’t rely on just one accuracy metric to evaluate your model’s performance.
- Consider efficiency indicators: Balance the accuracy and efficiency of the model.
- Report cross-validation results: Cross-validation results are provided to demonstrate the reliability of the performance.
- Compare model performance to baselines: Compare a model's performance to existing baselines to evaluate its effectiveness relative to other models.
The above is the detailed content of Performance Measurement of Python Natural Language Processing: Assessing Model Accuracy and Efficiency. For more information, please follow other related articles on the PHP Chinese website!

Hot AI Tools

Undresser.AI Undress
AI-powered app for creating realistic nude photos

AI Clothes Remover
Online AI tool for removing clothes from photos.

Undress AI Tool
Undress images for free

Clothoff.io
AI clothes remover

Video Face Swap
Swap faces in any video effortlessly with our completely free AI face swap tool!

Hot Article

Hot Tools

Notepad++7.3.1
Easy-to-use and free code editor

SublimeText3 Chinese version
Chinese version, very easy to use

Zend Studio 13.0.1
Powerful PHP integrated development environment

Dreamweaver CS6
Visual web development tools

SublimeText3 Mac version
God-level code editing software (SublimeText3)

Hot Topics










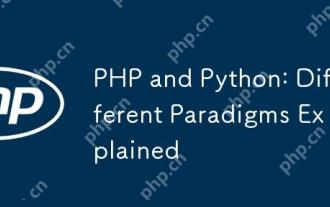
PHP is mainly procedural programming, but also supports object-oriented programming (OOP); Python supports a variety of paradigms, including OOP, functional and procedural programming. PHP is suitable for web development, and Python is suitable for a variety of applications such as data analysis and machine learning.
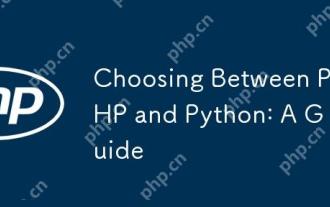
PHP is suitable for web development and rapid prototyping, and Python is suitable for data science and machine learning. 1.PHP is used for dynamic web development, with simple syntax and suitable for rapid development. 2. Python has concise syntax, is suitable for multiple fields, and has a strong library ecosystem.
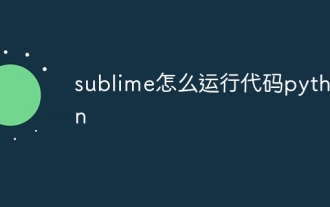
To run Python code in Sublime Text, you need to install the Python plug-in first, then create a .py file and write the code, and finally press Ctrl B to run the code, and the output will be displayed in the console.
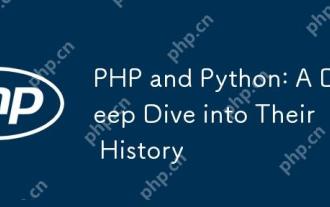
PHP originated in 1994 and was developed by RasmusLerdorf. It was originally used to track website visitors and gradually evolved into a server-side scripting language and was widely used in web development. Python was developed by Guidovan Rossum in the late 1980s and was first released in 1991. It emphasizes code readability and simplicity, and is suitable for scientific computing, data analysis and other fields.
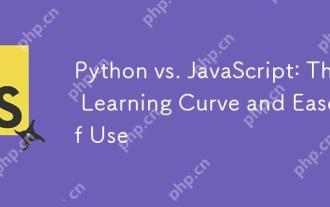
Python is more suitable for beginners, with a smooth learning curve and concise syntax; JavaScript is suitable for front-end development, with a steep learning curve and flexible syntax. 1. Python syntax is intuitive and suitable for data science and back-end development. 2. JavaScript is flexible and widely used in front-end and server-side programming.
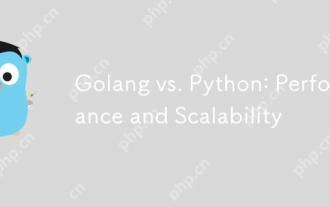
Golang is better than Python in terms of performance and scalability. 1) Golang's compilation-type characteristics and efficient concurrency model make it perform well in high concurrency scenarios. 2) Python, as an interpreted language, executes slowly, but can optimize performance through tools such as Cython.
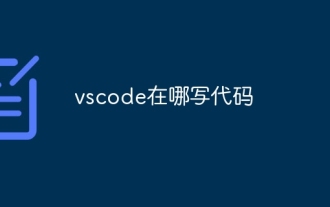
Writing code in Visual Studio Code (VSCode) is simple and easy to use. Just install VSCode, create a project, select a language, create a file, write code, save and run it. The advantages of VSCode include cross-platform, free and open source, powerful features, rich extensions, and lightweight and fast.
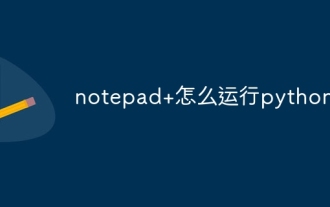
Running Python code in Notepad requires the Python executable and NppExec plug-in to be installed. After installing Python and adding PATH to it, configure the command "python" and the parameter "{CURRENT_DIRECTORY}{FILE_NAME}" in the NppExec plug-in to run Python code in Notepad through the shortcut key "F6".
