


Using AI short videos to 'feed back' long video understanding, Tencent's MovieLLM framework aims at movie-level continuous frame generation
In the field of video understanding, although multi-modal models have made breakthroughs in short video analysis and demonstrated strong understanding capabilities, when they face movie-level long videos, In the video, it seems powerless. Therefore, the analysis and understanding of long videos, especially the understanding of hours-long movie content, has become a huge challenge today.
The difficulty of the model in understanding long videos mainly stems from the lack of long video data resources, which have defects in quality and diversity. Additionally, collecting and labeling this data requires a lot of work.
Faced with such a problem, the research team from Tencent and Fudan University proposed MovieLLM, an innovative AI generation framework. MovieLLM adopts an innovative method that not only generates high-quality and diverse video data, but also automatically generates a large number of related question and answer data sets, greatly enriching the dimension and depth of the data. At the same time, the entire automated process is also extremely Dadi reduces human investment.
- ##Paper address: https://arxiv.org/abs/2403.01422
- Home page address: https://deaddawn.github.io/MovieLLM/
this Important advances not only improve the model's understanding of complex video narratives, but also enhance the model's analytical capabilities when processing hours-long movie content. At the same time, it overcomes the limitations of scarcity and bias of existing data sets and provides a new and effective way to understand ultra-long video content.
MovieLLM cleverly takes advantage of the powerful generation capabilities of GPT-4 and diffusion models, and adopts a "story expanding" continuous frame description generation strategy. The "textual inversion" method is used to guide the diffusion model to generate scene images that are consistent with the text description, thereby creating continuous frames of a complete movie.
Method Overview
MovieLLM combines GPT-4 and diffusion models to improve large models Understanding long videos. This clever combination produces high-quality, diverse long video data and QA questions and answers, helping to enhance the model's generative capabilities.
MovieLLM mainly includes three stages:
1. Movie plot generation.
Rather than relying on the web or existing datasets to generate plots, MovieLLM fully leverages the power of GPT-4 to produce synthetic data. By providing specific elements such as theme, overview, and style, GPT-4 is guided to produce cinematic keyframe descriptions tailored to the subsequent generation process.
#2. Style fixing process.
MovieLLM cleverly uses "textual inversion" technology to fix the style description generated in the script to the latent space of the diffusion model. This method guides the model to generate scenes with a fixed style and maintain diversity while maintaining a unified aesthetic.
#3. Video command data generation.
Based on the first two steps, fixed style embedding and key frame description have been obtained. Based on these, MovieLLM uses style embedding to guide the diffusion model to generate key frames that conform to key frame descriptions and gradually generates various instructional question and answer pairs according to the movie plot.
#After the above steps, MovieLLM creates high-quality, diverse styles, coherent movie frames and corresponding question and answer pair data. The detailed distribution of movie data types is as follows:
Experimental results
By fine-tuning LLaMA-VID, a large model focused on long video understanding, using data constructed based on MovieLLM, this paper significantly enhances The model's understanding of video content of various lengths. For long video understanding, there is currently no work proposing a test benchmark, so this article also proposes a benchmark for testing long video understanding capabilities.
Although MovieLLM did not specifically construct short video data for training, through training, performance improvements on various short video benchmarks were still observed. The results are as follows:
Compared with the baseline model, there is a significant improvement in the two test data sets of MSVD-QA and MSRVTT-QA.
On the performance benchmark based on video generation, performance improvements were achieved in all five evaluation areas.
#In terms of long video understanding, through the training of MovieLLM, the model's understanding of summary, plot and timing has been significantly improved.
In addition, MovieLLM also has better results in terms of generation quality compared to other similar methods of generating images with fixed styles.
In short, the data generation workflow proposed by MovieLLM significantly reduces the challenge of producing movie-level video data for the model and improves the generation of content. control and diversity. At the same time, MovieLLM significantly enhances the multi-modal model's ability to understand movie-level long videos, providing a valuable reference for other fields to adopt similar data generation methods.
Readers who are interested in this research can read the original text of the paper to learn more about the research content.
The above is the detailed content of Using AI short videos to 'feed back' long video understanding, Tencent's MovieLLM framework aims at movie-level continuous frame generation. For more information, please follow other related articles on the PHP Chinese website!

Hot AI Tools

Undresser.AI Undress
AI-powered app for creating realistic nude photos

AI Clothes Remover
Online AI tool for removing clothes from photos.

Undress AI Tool
Undress images for free

Clothoff.io
AI clothes remover

Video Face Swap
Swap faces in any video effortlessly with our completely free AI face swap tool!

Hot Article

Hot Tools

Notepad++7.3.1
Easy-to-use and free code editor

SublimeText3 Chinese version
Chinese version, very easy to use

Zend Studio 13.0.1
Powerful PHP integrated development environment

Dreamweaver CS6
Visual web development tools

SublimeText3 Mac version
God-level code editing software (SublimeText3)

Hot Topics
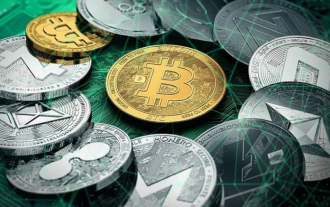
The top ten cryptocurrency trading platforms in the world include Binance, OKX, Gate.io, Coinbase, Kraken, Huobi Global, Bitfinex, Bittrex, KuCoin and Poloniex, all of which provide a variety of trading methods and powerful security measures.
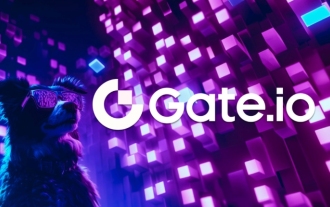
MeMebox 2.0 redefines crypto asset management through innovative architecture and performance breakthroughs. 1) It solves three major pain points: asset silos, income decay and paradox of security and convenience. 2) Through intelligent asset hubs, dynamic risk management and return enhancement engines, cross-chain transfer speed, average yield rate and security incident response speed are improved. 3) Provide users with asset visualization, policy automation and governance integration, realizing user value reconstruction. 4) Through ecological collaboration and compliance innovation, the overall effectiveness of the platform has been enhanced. 5) In the future, smart contract insurance pools, forecast market integration and AI-driven asset allocation will be launched to continue to lead the development of the industry.
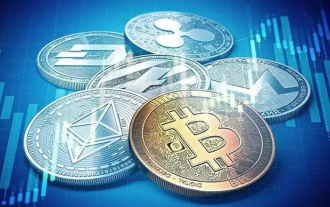
Currently ranked among the top ten virtual currency exchanges: 1. Binance, 2. OKX, 3. Gate.io, 4. Coin library, 5. Siren, 6. Huobi Global Station, 7. Bybit, 8. Kucoin, 9. Bitcoin, 10. bit stamp.
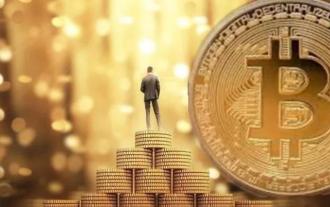
Recommended reliable digital currency trading platforms: 1. OKX, 2. Binance, 3. Coinbase, 4. Kraken, 5. Huobi, 6. KuCoin, 7. Bitfinex, 8. Gemini, 9. Bitstamp, 10. Poloniex, these platforms are known for their security, user experience and diverse functions, suitable for users at different levels of digital currency transactions
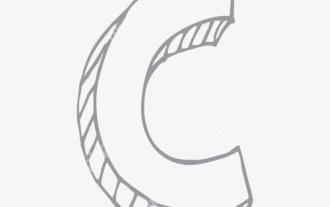
Using the chrono library in C can allow you to control time and time intervals more accurately. Let's explore the charm of this library. C's chrono library is part of the standard library, which provides a modern way to deal with time and time intervals. For programmers who have suffered from time.h and ctime, chrono is undoubtedly a boon. It not only improves the readability and maintainability of the code, but also provides higher accuracy and flexibility. Let's start with the basics. The chrono library mainly includes the following key components: std::chrono::system_clock: represents the system clock, used to obtain the current time. std::chron
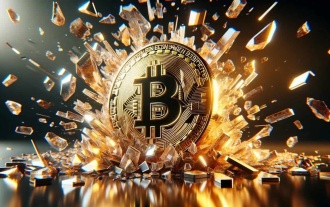
Bitcoin’s price ranges from $20,000 to $30,000. 1. Bitcoin’s price has fluctuated dramatically since 2009, reaching nearly $20,000 in 2017 and nearly $60,000 in 2021. 2. Prices are affected by factors such as market demand, supply, and macroeconomic environment. 3. Get real-time prices through exchanges, mobile apps and websites. 4. Bitcoin price is highly volatile, driven by market sentiment and external factors. 5. It has a certain relationship with traditional financial markets and is affected by global stock markets, the strength of the US dollar, etc. 6. The long-term trend is bullish, but risks need to be assessed with caution.
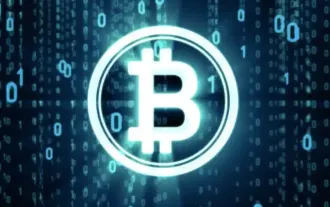
The top ten digital currency exchanges such as Binance, OKX, gate.io have improved their systems, efficient diversified transactions and strict security measures.
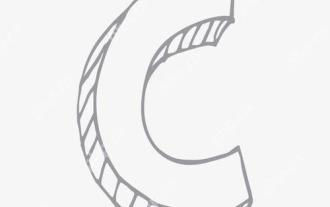
Measuring thread performance in C can use the timing tools, performance analysis tools, and custom timers in the standard library. 1. Use the library to measure execution time. 2. Use gprof for performance analysis. The steps include adding the -pg option during compilation, running the program to generate a gmon.out file, and generating a performance report. 3. Use Valgrind's Callgrind module to perform more detailed analysis. The steps include running the program to generate the callgrind.out file and viewing the results using kcachegrind. 4. Custom timers can flexibly measure the execution time of a specific code segment. These methods help to fully understand thread performance and optimize code.
