


Detailed explanation of Python computer vision algorithms: revealing the secrets behind image processing and analysis
Computer vision is a branch of computer science that attempts to build the ability of machines to perceive images and videos. Computer vision algorithms have made tremendous progress in recent years, thanks in large part to python.
Python is a high-level programming language that is simple and easy to learn. It has rich libraries and tools and is very suitable for computer vision research. andDevelop. This article will introduce several Python computer vision algorithms and provide demonstration code to help you understand how these algorithms work.
1. Image processing
Image processing is an important part of computer vision, which includes a series of operations for processing and analyzing images. These operations can be divided into two categories: point operations and area operations.
- Point operation: Point operation refers to an operation performed on each pixel of an image. Common point operations include brightness adjustments, color conversions, and sharpening.
import cv2 # 读取图像 image = cv2.imread("image.jpg") # 调整亮度 bright_image = cv2.addWeighted(image, 1.2, 0, 0) # 转换颜色空间 hsv_image = cv2.cvtColor(image, cv2.COLOR_BGR2HSV) # 锐化图像 sharpened_image = cv2.filter2D(image, -1, np.array([[0, -1, 0], [-1, 5, -1], [0, -1, 0]])) # 显示图像 cv2.imshow("Original Image", image) cv2.imshow("Bright Image", bright_image) cv2.imshow("HSV Image", hsv_image) cv2.imshow("Sharpened Image", sharpened_image) cv2.waiTKEy(0) cv2.destroyAllwindows()
- Region operation: Region operation refers to the operation performed within a certain area of an image. Common region operations include connected component analysis, morphological operations, and segmentation.
import cv2 # 读取图像 image = cv2.imread("image.jpg") # 连通分量分析 _, labels = cv2.connectedComponents(image) # 形态学操作 kernel = np.ones((5, 5), np.uint8) dilated_image = cv2.dilate(image, kernel) eroded_image = cv2.erode(image, kernel) # 分割图像 segmented_image = cv2.watershed(image, labels) # 显示图像 cv2.imshow("Original Image", image) cv2.imshow("Labeled Image", labels) cv2.imshow("Dilated Image", dilated_image) cv2.imshow("Eroded Image", eroded_image) cv2.imshow("Segmented Image", segmented_image) cv2.waitKey(0) cv2.destroyAllWindows()
2. Image analysis
Image analysis is another important component of computer vision, which includes a series of algorithms for extracting information from images. These algorithms can be divided into two categories: feature extraction and pattern recognition.
- Feature extraction: Feature extraction refers to extracting representative features from the image. Common feature extraction algorithms include edge detection, corner detection and texture analysis.
import cv2 # 读取图像 image = cv2.imread("image.jpg") # 边缘检测 edges = cv2.Canny(image, 100, 200) # 角点检测 corners = cv2.GoodFeaturesToTrack(image, 25, 0.01, 10) # 纹理分析 texture = cv2.texture(image) # 显示图像 cv2.imshow("Original Image", image) cv2.imshow("Edges", edges) cv2.imshow("Corners", corners) cv2.imshow("Texture", texture) cv2.waitKey(0) cv2.destroyAllWindows()
- Pattern recognition: Pattern recognition refers to matching features in images with known patterns.
The above is the detailed content of Detailed explanation of Python computer vision algorithms: revealing the secrets behind image processing and analysis. For more information, please follow other related articles on the PHP Chinese website!

Hot AI Tools

Undresser.AI Undress
AI-powered app for creating realistic nude photos

AI Clothes Remover
Online AI tool for removing clothes from photos.

Undress AI Tool
Undress images for free

Clothoff.io
AI clothes remover

Video Face Swap
Swap faces in any video effortlessly with our completely free AI face swap tool!

Hot Article

Hot Tools

Notepad++7.3.1
Easy-to-use and free code editor

SublimeText3 Chinese version
Chinese version, very easy to use

Zend Studio 13.0.1
Powerful PHP integrated development environment

Dreamweaver CS6
Visual web development tools

SublimeText3 Mac version
God-level code editing software (SublimeText3)

Hot Topics
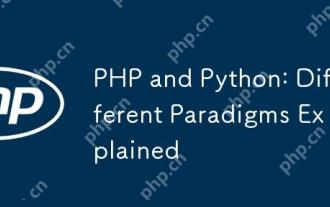
PHP is mainly procedural programming, but also supports object-oriented programming (OOP); Python supports a variety of paradigms, including OOP, functional and procedural programming. PHP is suitable for web development, and Python is suitable for a variety of applications such as data analysis and machine learning.
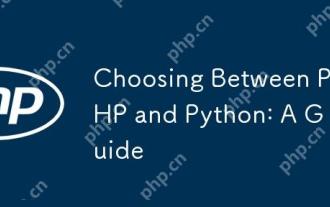
PHP is suitable for web development and rapid prototyping, and Python is suitable for data science and machine learning. 1.PHP is used for dynamic web development, with simple syntax and suitable for rapid development. 2. Python has concise syntax, is suitable for multiple fields, and has a strong library ecosystem.

Photoshop's advanced photo editing and synthesis technologies include: 1. Use layers, masks and adjustment layers for basic operations; 2. Use image pixel values to achieve photo editing effects; 3. Use multiple layers and masks for complex synthesis; 4. Use "liquefaction" tools to adjust facial features; 5. Use "frequency separation" technology to perform delicate photo editing, these technologies can improve image processing level and achieve professional-level effects.
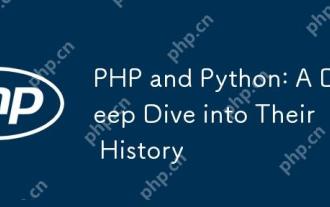
PHP originated in 1994 and was developed by RasmusLerdorf. It was originally used to track website visitors and gradually evolved into a server-side scripting language and was widely used in web development. Python was developed by Guidovan Rossum in the late 1980s and was first released in 1991. It emphasizes code readability and simplicity, and is suitable for scientific computing, data analysis and other fields.
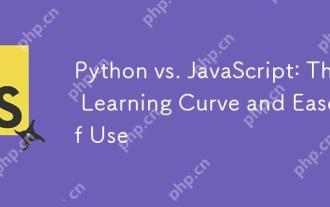
Python is more suitable for beginners, with a smooth learning curve and concise syntax; JavaScript is suitable for front-end development, with a steep learning curve and flexible syntax. 1. Python syntax is intuitive and suitable for data science and back-end development. 2. JavaScript is flexible and widely used in front-end and server-side programming.
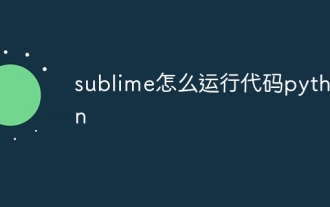
To run Python code in Sublime Text, you need to install the Python plug-in first, then create a .py file and write the code, and finally press Ctrl B to run the code, and the output will be displayed in the console.
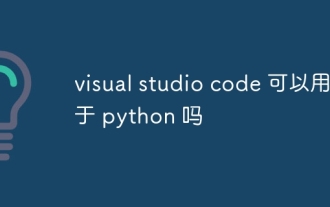
VS Code can be used to write Python and provides many features that make it an ideal tool for developing Python applications. It allows users to: install Python extensions to get functions such as code completion, syntax highlighting, and debugging. Use the debugger to track code step by step, find and fix errors. Integrate Git for version control. Use code formatting tools to maintain code consistency. Use the Linting tool to spot potential problems ahead of time.
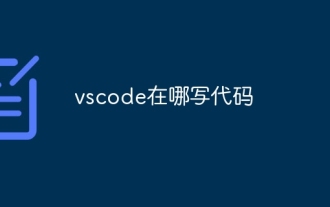
Writing code in Visual Studio Code (VSCode) is simple and easy to use. Just install VSCode, create a project, select a language, create a file, write code, save and run it. The advantages of VSCode include cross-platform, free and open source, powerful features, rich extensions, and lightweight and fast.
