


Detailed steps to implement stack in-order traversal of a binary tree using Python
使用堆栈无需递归就能遍历二叉树,下面是一个使用堆栈中序遍历二叉树的算法。
算法思路
1)创建一个空栈S。
2)将当前节点初始化为root
3)将当前节点推入S并设置current=current->left直到current为NULL
4)如果current为NULL且堆栈不为空,则
a)从堆栈中弹出顶部项目。
b)输出弹出的项目,设置current=popped_item->right
c)转到步骤3)。
5)如果current为NULL并且stack为空,那么算法结束。
算法实现步骤
1
/\
2 3
/\
4 5
步骤1创建一个空堆栈:S=NULL
步骤2将current设置为root的地址:current->1
步骤3推送当前节点并设置current=current->left
直到当前为NULL
当前->1
推1:堆栈S->1
当前->2
推2:堆栈>2,1
当前->4
推4:堆栈S>4、2、1
当前=NULL
步骤4从S弹出
a)弹出4:堆栈S->2,1
b)打印“4”
c)current=NULL/*right of 4*/并转到步骤3
由于current is NULL step 3没有做任何事情。
步骤4再次弹出。
a)弹出2:堆栈S->1
b)打印“2”
c)current->;5/*right of 2*/并转到步骤3
第3步将5推入堆栈并使当前为NULL
堆栈S->5,1
当前=NULL
步骤4从S弹出
a)弹出5:堆栈S->1
b)打印“5”
c)current=NULL/*right of 5*/并转到步骤3
由于current is NULL step 3没有做任何事情
步骤4再次弹出。
a)弹出1:堆栈S->NULL
b)打印“1”
c)当前->3/*1的右边*/
第3步将3推入堆栈并使当前为NULL
堆栈S->3
当前=NULL
步骤4从S弹出
a)弹出3:堆栈S->NULL
b)打印“3”
c)current=NULL/*3的右边*/
由于堆栈S为空且当前为NULL,因此遍历已完成。
Python实现堆栈中序遍历二叉树
class Node: def __init__(self,data): self.data=data self.left=None self.right=None def inOrder(root): current=root stack=[] while True: if current is not None: stack.append(current) current=current.left elif(stack): current=stack.pop() print(current.data,end="") current=current.right else: break print() root=Node(1) root.left=Node(2) root.right=Node(3) root.left.left=Node(4) root.left.right=Node(5) inOrder(root)
The above is the detailed content of Detailed steps to implement stack in-order traversal of a binary tree using Python. For more information, please follow other related articles on the PHP Chinese website!

Hot AI Tools

Undresser.AI Undress
AI-powered app for creating realistic nude photos

AI Clothes Remover
Online AI tool for removing clothes from photos.

Undress AI Tool
Undress images for free

Clothoff.io
AI clothes remover

Video Face Swap
Swap faces in any video effortlessly with our completely free AI face swap tool!

Hot Article

Hot Tools

Notepad++7.3.1
Easy-to-use and free code editor

SublimeText3 Chinese version
Chinese version, very easy to use

Zend Studio 13.0.1
Powerful PHP integrated development environment

Dreamweaver CS6
Visual web development tools

SublimeText3 Mac version
God-level code editing software (SublimeText3)

Hot Topics
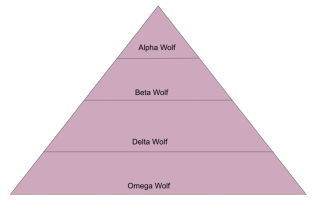
The Gray Wolf Optimization Algorithm (GWO) is a population-based metaheuristic algorithm that simulates the leadership hierarchy and hunting mechanism of gray wolves in nature. Gray Wolf Algorithm Inspiration 1. Gray wolves are considered to be apex predators and are at the top of the food chain. 2. Gray wolves like to live in groups (living in groups), with an average of 5-12 wolves in each pack. 3. Gray wolves have a very strict social dominance hierarchy, as shown below: Alpha wolf: Alpha wolf occupies a dominant position in the entire gray wolf group and has the right to command the entire gray wolf group. In the application of algorithms, Alpha Wolf is one of the best solutions, the optimal solution produced by the optimization algorithm. Beta wolf: Beta wolf reports to Alpha wolf regularly and helps Alpha wolf make the best decisions. In algorithm applications, Beta Wolf can
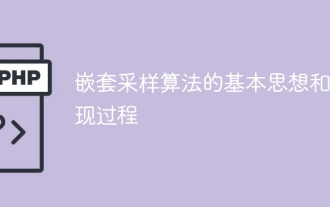
The nested sampling algorithm is an efficient Bayesian statistical inference algorithm used to calculate the integral or summation under complex probability distributions. It works by decomposing the parameter space into multiple hypercubes of equal volume, and gradually and iteratively "pushing out" one of the smallest volume hypercubes, and then filling the hypercube with random samples to better estimate the integral value of the probability distribution. . Through continuous iteration, the nested sampling algorithm can obtain high-precision integral values and boundaries of parameter space, which can be applied to statistical problems such as model comparison, parameter estimation, and model selection. The core idea of this algorithm is to transform complex integration problems into a series of simple integration problems, and approach the real integral value by gradually reducing the volume of the parameter space. Each iteration step randomly samples from the parameter space
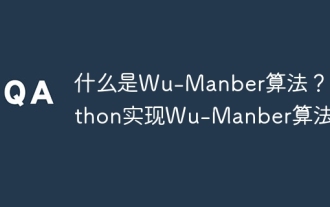
The Wu-Manber algorithm is a string matching algorithm used to search strings efficiently. It is a hybrid algorithm that combines the advantages of Boyer-Moore and Knuth-Morris-Pratt algorithms to provide fast and accurate pattern matching. Wu-Manber algorithm step 1. Create a hash table that maps each possible substring of the pattern to the pattern position where that substring occurs. 2. This hash table is used to quickly identify potential starting locations of patterns in text. 3. Iterate through the text and compare each character to the corresponding character in the pattern. 4. If the characters match, you can move to the next character and continue the comparison. 5. If the characters do not match, you can use a hash table to determine the next potential character in the pattern.
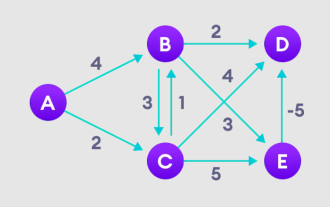
Bellman Ford algorithm can find the shortest path from the target node to other nodes in the weighted graph. This is very similar to the Dijkstra algorithm. The Bellman-Ford algorithm can handle graphs with negative weights and is relatively simple in terms of implementation. Detailed explanation of the principle of Bellman Ford algorithm The Bellman Ford algorithm iteratively finds new paths shorter than the overestimated paths by overestimating the path lengths from the starting vertex to all other vertices. Because we want to record the path distance of each node, we can store it in an array of size n, where n also represents the number of nodes. Example Figure 1. Select the starting node, assign it to all other vertices infinitely, and record the path value. 2. Visit each edge and perform relaxation operations to continuously update the shortest path. 3. We need
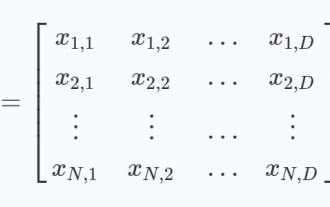
The Sparrow Search Algorithm (SSA) is a meta-heuristic optimization algorithm based on the anti-predation and foraging behavior of sparrows. The foraging behavior of sparrows can be divided into two main types: producers and scavengers. Producers actively search for food, while scavengers compete for food from producers. Principle of Sparrow Search Algorithm (SSA) In Sparrow Search Algorithm (SSA), each sparrow pays close attention to the behavior of its neighbors. By employing different foraging strategies, individuals are able to efficiently use retained energy to pursue more food. Additionally, birds are more vulnerable to predators in their search space, so they need to find safer locations. Birds at the center of a colony can minimize their own range of danger by staying close to their neighbors. When a bird spots a predator, it makes an alarm call to
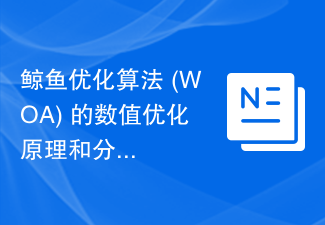
The Whale Optimization Algorithm (WOA) is a nature-inspired metaheuristic optimization algorithm that simulates the hunting behavior of humpback whales and is used for the optimization of numerical problems. The Whale Optimization Algorithm (WOA) starts with a set of random solutions and optimizes based on a randomly selected search agent or the best solution so far through position updates of the search agent in each iteration. Whale Optimization Algorithm Inspiration The Whale Optimization Algorithm is inspired by the hunting behavior of humpback whales. Humpback whales prefer food found near the surface, such as krill and schools of fish. Therefore, humpback whales gather food together to form a bubble network by blowing bubbles in a bottom-up spiral when hunting. In an "upward spiral" maneuver, the humpback whale dives about 12m, then begins to form a spiral bubble around its prey and swims upward
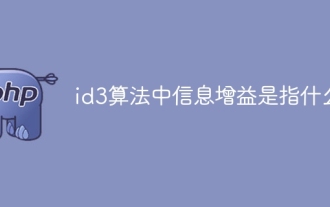
The ID3 algorithm is one of the basic algorithms in decision tree learning. It selects the best split point by calculating the information gain of each feature to generate a decision tree. Information gain is an important concept in the ID3 algorithm, which is used to measure the contribution of features to the classification task. This article will introduce in detail the concept, calculation method and application of information gain in the ID3 algorithm. 1. The concept of information entropy Information entropy is a concept in information theory, which measures the uncertainty of random variables. For a discrete random variable number, and p(x_i) represents the probability that the random variable X takes the value x_i. letter
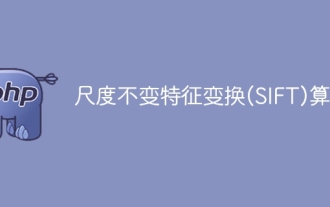
The Scale Invariant Feature Transform (SIFT) algorithm is a feature extraction algorithm used in the fields of image processing and computer vision. This algorithm was proposed in 1999 to improve object recognition and matching performance in computer vision systems. The SIFT algorithm is robust and accurate and is widely used in image recognition, three-dimensional reconstruction, target detection, video tracking and other fields. It achieves scale invariance by detecting key points in multiple scale spaces and extracting local feature descriptors around the key points. The main steps of the SIFT algorithm include scale space construction, key point detection, key point positioning, direction assignment and feature descriptor generation. Through these steps, the SIFT algorithm can extract robust and unique features, thereby achieving efficient image processing.
