In-depth analysis of the BERT model
1. What the BERT model can do
The BERT model is a natural language processing model based on the Transformer model, used to process text classification , question answering system, named entity recognition and semantic similarity calculation and other tasks. Due to its excellent performance in multiple natural language processing tasks, the BERT model has become one of the most advanced pre-trained language models and has received widespread attention and application.
The full name of the BERT model is Bidirectional Encoder Representations from Transformers, that is, bidirectional encoder converter representation. Compared with traditional natural language processing models, the BERT model has the following significant advantages: First, the BERT model can simultaneously consider the context information of the surrounding context to better understand semantics and context. Secondly, the BERT model uses the Transformer architecture to enable the model to process input sequences in parallel, speeding up training and inference. In addition, the BERT model can achieve better results on various tasks through pre-training and fine-tuning, and has better transfer learning
The BERT model is a two-way The encoder can synthesize the context information of the text and understand the meaning of the text more accurately.
The BERT model learns richer text representations and improves downstream task performance through pre-training on unlabeled text data.
Fine-tuning: The BERT model can be fine-tuned to adapt to specific tasks, which allows it to be applied in multiple natural language processing tasks and perform well.
The BERT model is improved on the basis of the Transformer model, mainly in the following aspects:
1.Masked Language Model (MLM) : The BERT model uses the MLM method in the pre-training stage, that is, randomly covering the input text, and then letting the model predict what the covered words are. This approach forces the model to learn contextual information and can effectively reduce data sparsity problems.
2.Next Sentence Prediction (NSP): The BERT model also uses the NSP method, which allows the model to determine whether two sentences are adjacent during the pre-training stage. This approach can help the model learn the relationship between texts and thus better understand the meaning of the text.
3.Transformer Encoder: The BERT model uses the Transformer Encoder as the basic model. Through the stacking of multiple layers of Transformer Encoder, a deep neural network structure is constructed to obtain a richer feature representation. ability.
4.Fine-tuning: The BERT model also uses Fine-tuning to adapt to specific tasks. By fine-tuning the model based on the pre-trained model, it can better adapt to different tasks. This method has shown good results in multiple natural language processing tasks.
2. How long does it take to train the BERT model?
Generally speaking, the pre-training of the BERT model takes several days to weeks. , depending on the influence of the following factors:
1. Data set size: The BERT model requires a large amount of unlabeled text data for pre-training. The larger the data set, the longer the training time. The longer.
2. Model scale: The larger the BERT model, the more computing resources and training time it requires.
3. Computing resources: The training of the BERT model requires the use of large-scale computing resources, such as GPU clusters, etc. The quantity and quality of computing resources will affect the training time.
4. Training strategy: The training of the BERT model also requires the use of some efficient training strategies, such as gradient accumulation, dynamic learning rate adjustment, etc. These strategies will also affect the training time.
3. Parameter structure of BERT model
The parameter structure of BERT model can be divided into the following parts:
1) Word Embedding Layer (Embedding Layer): Convert the input text into word vectors. Generally, algorithms such as WordPiece or BPE are used for word segmentation and encoding.
2) Transformer Encoder layer: The BERT model uses multi-layer Transformer Encoder for feature extraction and representation learning. Each Encoder contains multiple Self-Attention and Feed-Forward sub-layers.
3) Pooling Layer: Pool the outputs of multiple Transformer Encoder layers to generate a fixed-length vector as the representation of the entire sentence.
4) Output layer: Designed according to specific tasks, it can be a single classifier, sequence annotator, regressor, etc.
The BERT model has a very large number of parameters. It is generally trained through pre-training, and then fine-tuned on specific tasks through Fine-tuning.
4. BERT model tuning skills
The tuning skills of the BERT model can be divided into the following aspects:
1) Learning rate adjustment: The training of the BERT model requires learning rate adjustment. Generally, warmup and decay are used to adjust the model so that the model can converge better.
2) Gradient accumulation: Since the number of parameters of the BERT model is very large, the calculation amount of updating all parameters at one time is very large, so the gradient accumulation method can be used for optimization, that is, multiple calculations The obtained gradients are accumulated, and then the model is updated in one go.
3) Model compression: The BERT model is large in scale and requires a large amount of computing resources for training and inference. Therefore, model compression can be used to reduce the model size and calculation amount. Commonly used model compression techniques include model pruning, quantization, and distillation.
4) Data enhancement: In order to improve the generalization ability of the model, data enhancement methods can be used, such as random masking, data repetition, word exchange, etc., to expand the training data set.
5) Hardware optimization: The training and inference of the BERT model require a large amount of computing resources, so high-performance hardware such as GPU or TPU can be used to accelerate the training and inference process, thereby improving the performance of the model. Training efficiency and inference speed.
6) Fine-tuning strategy: For different tasks, different Fine-tuning strategies can be used to optimize the performance of the model, such as fine-tuning levels, learning rate adjustment, gradient accumulation, etc. .
In general, the BERT model is a pre-trained language model based on the Transformer model. Through the stacking of multi-layer Transformer Encoder and improvements such as MLM and NSP, it can be used in natural language. Impressive performance in handling. At the same time, the BERT model also provides new ideas and methods for the research of other natural language processing tasks.
The above is the detailed content of In-depth analysis of the BERT model. For more information, please follow other related articles on the PHP Chinese website!

Hot AI Tools

Undresser.AI Undress
AI-powered app for creating realistic nude photos

AI Clothes Remover
Online AI tool for removing clothes from photos.

Undress AI Tool
Undress images for free

Clothoff.io
AI clothes remover

Video Face Swap
Swap faces in any video effortlessly with our completely free AI face swap tool!

Hot Article

Hot Tools

Notepad++7.3.1
Easy-to-use and free code editor

SublimeText3 Chinese version
Chinese version, very easy to use

Zend Studio 13.0.1
Powerful PHP integrated development environment

Dreamweaver CS6
Visual web development tools

SublimeText3 Mac version
God-level code editing software (SublimeText3)

Hot Topics










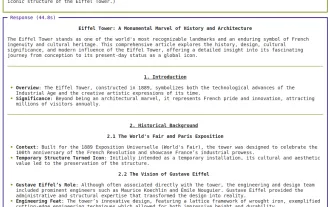
While working on Agentic AI, developers often find themselves navigating the trade-offs between speed, flexibility, and resource efficiency. I have been exploring the Agentic AI framework and came across Agno (earlier it was Phi-
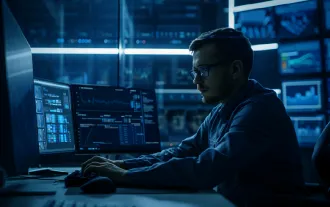
SQL's ALTER TABLE Statement: Dynamically Adding Columns to Your Database In data management, SQL's adaptability is crucial. Need to adjust your database structure on the fly? The ALTER TABLE statement is your solution. This guide details adding colu
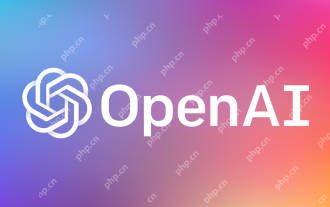
The release includes three distinct models, GPT-4.1, GPT-4.1 mini and GPT-4.1 nano, signaling a move toward task-specific optimizations within the large language model landscape. These models are not immediately replacing user-facing interfaces like
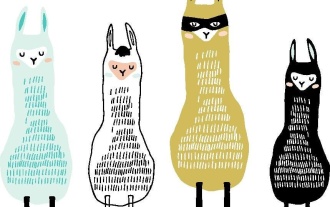
Troubled Benchmarks: A Llama Case Study In early April 2025, Meta unveiled its Llama 4 suite of models, boasting impressive performance metrics that positioned them favorably against competitors like GPT-4o and Claude 3.5 Sonnet. Central to the launc
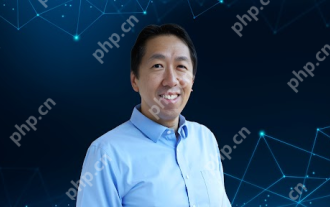
Unlock the Power of Embedding Models: A Deep Dive into Andrew Ng's New Course Imagine a future where machines understand and respond to your questions with perfect accuracy. This isn't science fiction; thanks to advancements in AI, it's becoming a r
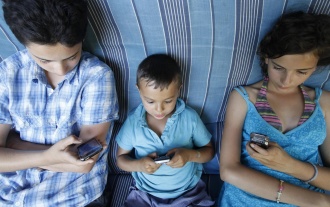
Can a video game ease anxiety, build focus, or support a child with ADHD? As healthcare challenges surge globally — especially among youth — innovators are turning to an unlikely tool: video games. Now one of the world’s largest entertainment indus
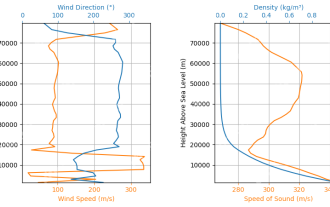
Simulate Rocket Launches with RocketPy: A Comprehensive Guide This article guides you through simulating high-power rocket launches using RocketPy, a powerful Python library. We'll cover everything from defining rocket components to analyzing simula
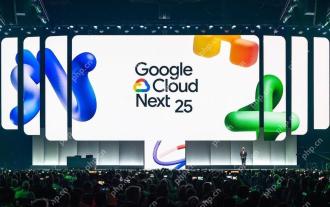
Gemini as the Foundation of Google’s AI Strategy Gemini is the cornerstone of Google’s AI agent strategy, leveraging its advanced multimodal capabilities to process and generate responses across text, images, audio, video and code. Developed by DeepM
