


Google launches BIG-Bench Mistake data set to help AI improve error correction capabilities
Google Research recently conducted an evaluation study on popular language models, using its own BIG-Bench benchmark and the newly established "BIG-Bench Mistake" data set. They mainly focused on the error probability and error correction ability of the language model. This study provides valuable data to better understand the performance of language models on the market.
Google researchers said they created a special benchmark data set called "BIG-Bench Mistake" to evaluate the "error probability" and "self-correction ability" of large language models. This is due to the lack of corresponding data sets in the past to effectively evaluate and test these key indicators.
The researchers used the PaLM language model to run 5 tasks in their own BIG-Bench benchmark task, and added the generated "Chain-of-Thought" trajectory to the "Logic Error" part. Retest model accuracy.
In order to improve the accuracy of the data set, Google researchers repeatedly performed the above process and finally created a benchmark data set specifically for evaluation, which contains 255 logical errors, called "BIG-Bench Mistake" .
The researchers pointed out that the logical errors in the "BIG-Bench Mistake" data set are very obvious and therefore can be used as a good standard for language model testing. This dataset helps the model learn from simple errors and gradually improve its ability to identify errors.
The researchers used this data set to test models on the market and found that although most language models can identify logical errors in the reasoning process and correct themselves, this process is not very ideal. Often, human intervention is also required to correct what the model outputs.
▲ Picture source Google Research Press Release
According to the report, Google claims that it is considered the most advanced large language model currently, but its self-correction ability is relatively limited. In tests, the best-performing model found only 52.9% of logical errors.
Google researchers also claimed that this BIG-Bench Mistake data set is conducive to improving the self-correction ability of the model. After fine-tuning the model on relevant test tasks, "even small model performance is usually better than that of large models with zero sample prompts." better".
According to this, Google believes that in terms of model error correction, proprietary small models can be used to "supervise" large models. Instead of letting large language models learn to "correct self-errors", deploying small dedicated models dedicated to supervising large models has the advantage of This will help improve efficiency, reduce related AI deployment costs, and make fine-tuning easier.
The above is the detailed content of Google launches BIG-Bench Mistake data set to help AI improve error correction capabilities. For more information, please follow other related articles on the PHP Chinese website!

Hot AI Tools

Undresser.AI Undress
AI-powered app for creating realistic nude photos

AI Clothes Remover
Online AI tool for removing clothes from photos.

Undress AI Tool
Undress images for free

Clothoff.io
AI clothes remover

Video Face Swap
Swap faces in any video effortlessly with our completely free AI face swap tool!

Hot Article

Hot Tools

Notepad++7.3.1
Easy-to-use and free code editor

SublimeText3 Chinese version
Chinese version, very easy to use

Zend Studio 13.0.1
Powerful PHP integrated development environment

Dreamweaver CS6
Visual web development tools

SublimeText3 Mac version
God-level code editing software (SublimeText3)

Hot Topics










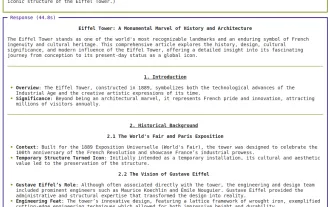
While working on Agentic AI, developers often find themselves navigating the trade-offs between speed, flexibility, and resource efficiency. I have been exploring the Agentic AI framework and came across Agno (earlier it was Phi-
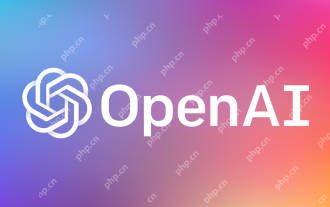
The release includes three distinct models, GPT-4.1, GPT-4.1 mini and GPT-4.1 nano, signaling a move toward task-specific optimizations within the large language model landscape. These models are not immediately replacing user-facing interfaces like
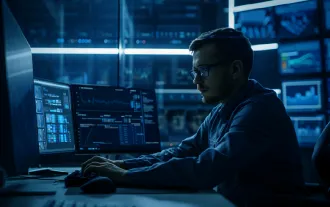
SQL's ALTER TABLE Statement: Dynamically Adding Columns to Your Database In data management, SQL's adaptability is crucial. Need to adjust your database structure on the fly? The ALTER TABLE statement is your solution. This guide details adding colu
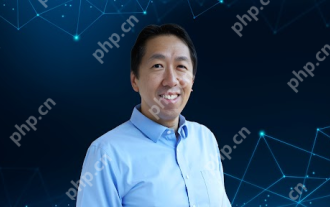
Unlock the Power of Embedding Models: A Deep Dive into Andrew Ng's New Course Imagine a future where machines understand and respond to your questions with perfect accuracy. This isn't science fiction; thanks to advancements in AI, it's becoming a r
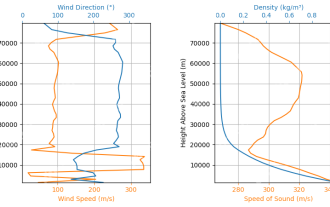
Simulate Rocket Launches with RocketPy: A Comprehensive Guide This article guides you through simulating high-power rocket launches using RocketPy, a powerful Python library. We'll cover everything from defining rocket components to analyzing simula
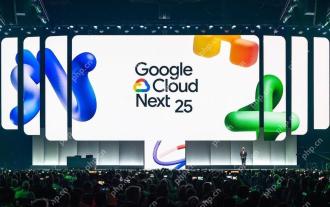
Gemini as the Foundation of Google’s AI Strategy Gemini is the cornerstone of Google’s AI agent strategy, leveraging its advanced multimodal capabilities to process and generate responses across text, images, audio, video and code. Developed by DeepM
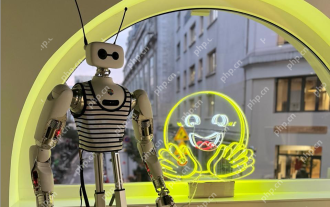
“Super happy to announce that we are acquiring Pollen Robotics to bring open-source robots to the world,” Hugging Face said on X. “Since Remi Cadene joined us from Tesla, we’ve become the most widely used software platform for open robotics thanks to
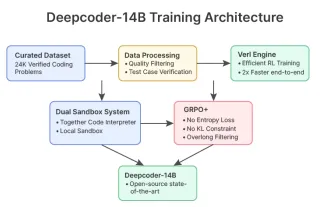
In a significant development for the AI community, Agentica and Together AI have released an open-source AI coding model named DeepCoder-14B. Offering code generation capabilities on par with closed-source competitors like OpenAI
