


Transformer model dimensionality reduction reduces, and LLM performance remains unchanged when more than 90% of the components of a specific layer are removed.
In the era of large-scale models, Transformer alone supports the entire scientific research field. Since its release, Transformer-based language models have demonstrated excellent performance on a variety of tasks. The underlying Transformer architecture has become state-of-the-art in natural language modeling and inference, and has also shown promise in fields such as computer vision and reinforcement learning. It shows strong prospects
The current Transformer architecture is very large and usually requires a lot of computing resources for training and inference
This is intentional This is because a Transformer trained with more parameters or data is obviously more capable than other models. Nonetheless, a growing body of work shows that Transformer-based models as well as neural networks do not require all fitted parameters to preserve their learned hypotheses.
Generally speaking, massive overparameterization seems to be helpful when training models, but these models can be pruned heavily before inference; research shows that neural networks can often remove 90 % or more weight without significant performance degradation. This phenomenon prompted researchers to turn to the study of pruning strategies that help model reasoning
Researchers from MIT and Microsoft reported in an article titled "The Truth Is Therein" A surprising discovery was made in the paper: Improving Language Model Inference Power through Layer Selective Ranking Reduction. They found that fine pruning at specific layers of the Transformer model can significantly improve the model's performance on certain tasks
- paper Address: https://arxiv.org/pdf/2312.13558.pdf
- Paper homepage: https://pratyushasharma.github.io/laser/
The study calls this simple intervention LASER (LAyer SElective Rank reduction). It significantly improves the performance of LLM by selectively reducing the high-order components of the learning weight matrix of a specific layer in the Transformer model through singular value decomposition. This operation can be performed after the model training is completed and does not require additional parameters or data
During the operation, the weight reduction is performed by performing model-specific weight matrices and layers of. The study also found that many similar matrices were able to significantly reduce weights, and that performance degradation was generally not observed until more than 90% of the components were removed
The study also found that reducing these factors can significantly improve accuracy. Interestingly, this finding applies not only to natural language, but also to reinforcement learning, which improves performance. Additionally, this study attempts to infer what is stored in higher-order components in order to Improve performance by deleting. The study found that after using LASER to answer questions, the original model mainly responded using high-frequency words (such as "the", "of", etc.). These words do not even match the semantic type of the correct answer, which means that without intervention, these components will cause the model to generate some irrelevant high-frequency words
However, by After some degree of rank reduction, the model's answers can be transformed into correct ones.
To understand this, the study also explored what the remaining components each encode, using only their higher-order singular vectors to approximate the weight matrix. It was found that these components described different responses or common high-frequency words in the same semantic category as the correct answer.
These results suggest that when noisy higher-order components are combined with lower-order components, their conflicting responses produce an average answer that may be incorrect. Figure 1 provides a visual representation of the Transformer architecture and the procedure followed by LASER. Here, the weight matrix of a specific layer of multilayer perceptron (MLP) is replaced by its low-rank approximation.
LASER Overview
The researcher introduced the LASER intervention in detail. Single-step LASER intervention is defined by three parameters (τ, ℓ and ρ). Together these parameters describe the matrix to be replaced by the low-rank approximation and the degree of approximation. The researcher classifies the matrices to be intervened according to parameter types
The researcher focuses on the matrix W = {W_q, W_k, W_v, W_o, U_in, U_out}, which consists of multiple It consists of a layer perceptron (MLP) and a matrix in the attention layer. The number of layers represents the level of researcher intervention, where the index of the first layer is 0. For example, Llama-2 has 32 levels, so it is expressed as ℓ ∈ {0, 1, 2,・・・31}
Ultimately, ρ ∈ [0, 1) describes which part of the maximum rank should be preserved when making low-rank approximations. For example, assuming , the maximum rank of the matrix is d. The researchers replaced it with the ⌊ρ・d⌋- approximation.
The following are required In Figure 1 below, an example of LASER is shown. The symbols τ = U_in and ℓ = L in the figure indicate that the first layer weight matrix of the MLP is updated in the Transformer block of the Lth layer. There is also a parameter used to control the k value in the rank-k approximation
LASER can limit the flow of certain information in the network and unexpectedly Unexpectedly yields significant performance benefits. These interventions can also be easily combined, such as applying a set of interventions in any order.
The LASER method is simply a search for such interventions, modified to deliver the greatest benefit. However, there are many other ways to combine these interventions, which is a direction for future work.
Experimental results
In the experimental part, the researcher used the GPT-J model pre-trained on the PILE data set. The model's The number of layers is 27 and the parameters are 6 billion. The model's behavior is then evaluated on the CounterFact dataset, which contains samples of (topic, relation, and answer) triples, with three paraphrase prompts provided for each question.
First, we analyzed the GPT-J model on the CounterFact dataset. Figure 2 shows the impact on the classification loss of a dataset after applying different amounts of rank reduction to each matrix in the Transformer architecture. Each Transformer layer consists of a two-layer small MLP, with input and output matrices shown separately. Different colors represent different percentages of removed components
Regarding improving the accuracy and robustness of interpretation, as shown in Figure 2 above and Table 1 below, The researchers found that when performing rank reduction on a single layer, the factual accuracy of the GPT-J model on the CounterFact dataset increased from 13.1% to 24.0%. It is important to note that these improvements are only the result of rank reduction and do not involve any further training or fine-tuning of the model.
Which facts will be restored when performing downrank recovery? The researchers found that the facts obtained through rank reduction are likely to appear very rarely in the data set, as shown in Figure 3
High-order What does the component store? The researchers use high-order components to approximate the final weight matrix (instead of using low-order components like LASER), as shown in Figure 5 (a) below. They measured the average cosine similarity of the true answers relative to the predicted answers when approximating the matrix using different numbers of higher-order components, as shown in Figure 5(b) below.
The researchers finally evaluated the generalizability of the three different LLMs they found on multiple language understanding tasks. For each task, they evaluated the performance of the model using three metrics: generation accuracy, classification accuracy, and loss. According to the results in Table 1, even if the rank of the matrix is greatly reduced, it will not cause the accuracy of the model to decrease, but can instead improve the performance of the model
The above is the detailed content of Transformer model dimensionality reduction reduces, and LLM performance remains unchanged when more than 90% of the components of a specific layer are removed.. For more information, please follow other related articles on the PHP Chinese website!

Hot AI Tools

Undresser.AI Undress
AI-powered app for creating realistic nude photos

AI Clothes Remover
Online AI tool for removing clothes from photos.

Undress AI Tool
Undress images for free

Clothoff.io
AI clothes remover

Video Face Swap
Swap faces in any video effortlessly with our completely free AI face swap tool!

Hot Article

Hot Tools

Notepad++7.3.1
Easy-to-use and free code editor

SublimeText3 Chinese version
Chinese version, very easy to use

Zend Studio 13.0.1
Powerful PHP integrated development environment

Dreamweaver CS6
Visual web development tools

SublimeText3 Mac version
God-level code editing software (SublimeText3)

Hot Topics










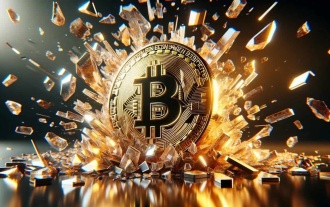
Bitcoin’s price ranges from $20,000 to $30,000. 1. Bitcoin’s price has fluctuated dramatically since 2009, reaching nearly $20,000 in 2017 and nearly $60,000 in 2021. 2. Prices are affected by factors such as market demand, supply, and macroeconomic environment. 3. Get real-time prices through exchanges, mobile apps and websites. 4. Bitcoin price is highly volatile, driven by market sentiment and external factors. 5. It has a certain relationship with traditional financial markets and is affected by global stock markets, the strength of the US dollar, etc. 6. The long-term trend is bullish, but risks need to be assessed with caution.
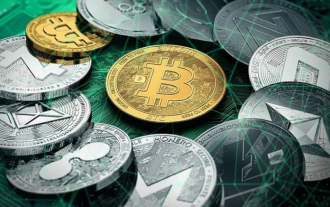
The top ten cryptocurrency trading platforms in the world include Binance, OKX, Gate.io, Coinbase, Kraken, Huobi Global, Bitfinex, Bittrex, KuCoin and Poloniex, all of which provide a variety of trading methods and powerful security measures.
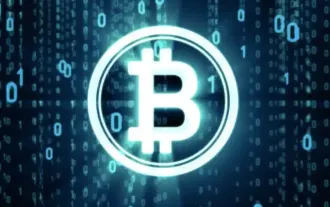
The top ten digital currency exchanges such as Binance, OKX, gate.io have improved their systems, efficient diversified transactions and strict security measures.
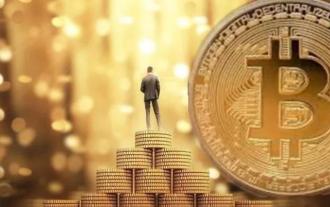
Recommended reliable digital currency trading platforms: 1. OKX, 2. Binance, 3. Coinbase, 4. Kraken, 5. Huobi, 6. KuCoin, 7. Bitfinex, 8. Gemini, 9. Bitstamp, 10. Poloniex, these platforms are known for their security, user experience and diverse functions, suitable for users at different levels of digital currency transactions
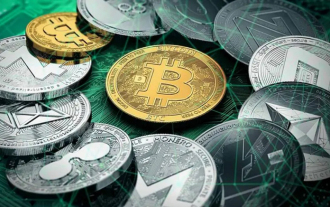
The top ten cryptocurrency exchanges in the world in 2025 include Binance, OKX, Gate.io, Coinbase, Kraken, Huobi, Bitfinex, KuCoin, Bittrex and Poloniex, all of which are known for their high trading volume and security.
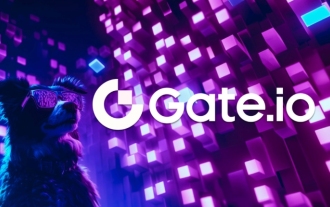
MeMebox 2.0 redefines crypto asset management through innovative architecture and performance breakthroughs. 1) It solves three major pain points: asset silos, income decay and paradox of security and convenience. 2) Through intelligent asset hubs, dynamic risk management and return enhancement engines, cross-chain transfer speed, average yield rate and security incident response speed are improved. 3) Provide users with asset visualization, policy automation and governance integration, realizing user value reconstruction. 4) Through ecological collaboration and compliance innovation, the overall effectiveness of the platform has been enhanced. 5) In the future, smart contract insurance pools, forecast market integration and AI-driven asset allocation will be launched to continue to lead the development of the industry.
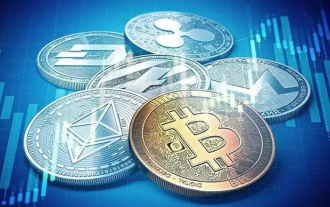
Currently ranked among the top ten virtual currency exchanges: 1. Binance, 2. OKX, 3. Gate.io, 4. Coin library, 5. Siren, 6. Huobi Global Station, 7. Bybit, 8. Kucoin, 9. Bitcoin, 10. bit stamp.
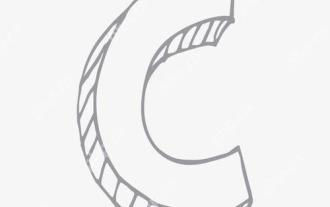
Measuring thread performance in C can use the timing tools, performance analysis tools, and custom timers in the standard library. 1. Use the library to measure execution time. 2. Use gprof for performance analysis. The steps include adding the -pg option during compilation, running the program to generate a gmon.out file, and generating a performance report. 3. Use Valgrind's Callgrind module to perform more detailed analysis. The steps include running the program to generate the callgrind.out file and viewing the results using kcachegrind. 4. Custom timers can flexibly measure the execution time of a specific code segment. These methods help to fully understand thread performance and optimize code.
