


Take a trip to the future, the first multi-view prediction + planning autonomous driving world model arrives
Recently, the concept of world model has triggered a wave of enthusiasm, but how can the field of autonomous driving watch the "fire" from afar. A team from the Institute of Automation, Chinese Academy of Sciences, proposed for the first time a new multi-view world model called Drive-WM, aiming to enhance the safety of end-to-end autonomous driving planning.
Website: https://drive-wm.github.io
Paper URL: https ://arxiv.org/abs/2311.17918
The first multi-view prediction and planning autonomous driving world model
In CVPR2023 Autonomous Driving At the seminar, the two major technology giants Tesla and Wayve showed off their black technology, and a new concept called "generative world model" became popular in the field of autonomous driving. Wayve even released the GAIA-1 generative AI model, demonstrating its stunning video scene generation capabilities. Recently, researchers from the Institute of Automation of the Chinese Academy of Sciences have also proposed a new autonomous driving world model - Drive-WM, which realizes a multi-view predictive world model for the first time and is seamlessly integrated with the current mainstream end-to-end autonomous driving planner. .
Drive-WM leverages the powerful generation capabilities of the Diffusion model to generate realistic video scenes.
Imagine that you are driving, and your on-board system is predicting future developments based on your driving habits and road conditions, and generating corresponding visual feedback to guide the selection of trajectory routes. This ability to foresee the future combined with a planner will greatly improve the safety of autonomous driving!
#Forecasting and planning based on multi-view world models.
#The combination of world model and end-to-end autonomous driving improves driving safety
## The #Drive-WM model combines the world model with end-to-end planning for the first time, opening a new chapter in the development of end-to-end autonomous driving. At each time step, the planner can use the world model to predict possible future scenarios, and then use the image reward function to fully evaluate them.
Adopt the best Estimation method and extended planning tree technology can achieve more effective and safer planning
Drive-WM explores two applications of world models in end-to-end planning through innovative research
1. Demonstrates the use of world models in the face of OOD scene robustness. Through comparative experiments, the author found that the current end-to-end planner's performance is not ideal when facing OOD situations.
The author gives the following picture. When a slight lateral offset is perturbed to the initial position, it is difficult for the current end-to-end planner to output a reasonable planned route.
The end-to-end planner has difficulty outputting a reasonable planned route when facing an OOD situation.
Drive-WM’s powerful generation capability provides new ideas for solving OOD problems. The author uses the generated videos to fine-tune the planner and learn from the OOD data, so that the planner can have better performance when facing such a scenario
2. This shows Introducing the enhanced role of future scenario evaluation in end-to-end planning
How to build a multi-view video generation model
Spatial-temporal consistency of multi-view video generation has always been a challenging problem. Drive-WM expands the capabilities of video generation by introducing temporal layer coding, and achieves multi-view video generation through view decomposition modeling. This generation method of view decomposition can greatly improve the consistency between views
Drive-WM overall model design
High-quality video generation and controllability
Drive-WM achieves high-quality multi-view video generation with excellent Controllability. It provides a variety of control options to control the generation of multi-view videos through text, scene layout, and motion information, and also provides new possibilities for future neural simulators
For example, use text to change weather and lighting:
##For example, pedestrian generation and foreground editing:
Use speed and direction control methods:
Generate rare events , such as turning around at an intersection or driving into the grass on the side
Conclusion
Drive-WM not only demonstrated its powerful multi-view video generation capabilities, but also revealed the world model and terminal There is huge potential in combining end-to-end driving models. We believe that in the future, world models can help achieve a safer, more stable, and more reliable end-to-end autonomous driving system.
The above is the detailed content of Take a trip to the future, the first multi-view prediction + planning autonomous driving world model arrives. For more information, please follow other related articles on the PHP Chinese website!

Hot AI Tools

Undresser.AI Undress
AI-powered app for creating realistic nude photos

AI Clothes Remover
Online AI tool for removing clothes from photos.

Undress AI Tool
Undress images for free

Clothoff.io
AI clothes remover

Video Face Swap
Swap faces in any video effortlessly with our completely free AI face swap tool!

Hot Article

Hot Tools

Notepad++7.3.1
Easy-to-use and free code editor

SublimeText3 Chinese version
Chinese version, very easy to use

Zend Studio 13.0.1
Powerful PHP integrated development environment

Dreamweaver CS6
Visual web development tools

SublimeText3 Mac version
God-level code editing software (SublimeText3)

Hot Topics
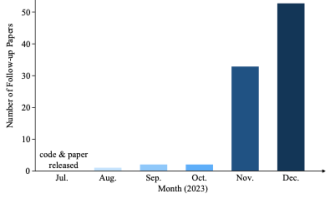
Written above & the author’s personal understanding Three-dimensional Gaussiansplatting (3DGS) is a transformative technology that has emerged in the fields of explicit radiation fields and computer graphics in recent years. This innovative method is characterized by the use of millions of 3D Gaussians, which is very different from the neural radiation field (NeRF) method, which mainly uses an implicit coordinate-based model to map spatial coordinates to pixel values. With its explicit scene representation and differentiable rendering algorithms, 3DGS not only guarantees real-time rendering capabilities, but also introduces an unprecedented level of control and scene editing. This positions 3DGS as a potential game-changer for next-generation 3D reconstruction and representation. To this end, we provide a systematic overview of the latest developments and concerns in the field of 3DGS for the first time.
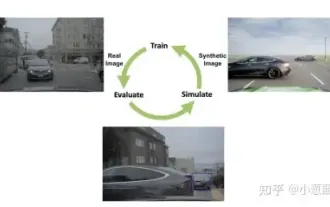
Yesterday during the interview, I was asked whether I had done any long-tail related questions, so I thought I would give a brief summary. The long-tail problem of autonomous driving refers to edge cases in autonomous vehicles, that is, possible scenarios with a low probability of occurrence. The perceived long-tail problem is one of the main reasons currently limiting the operational design domain of single-vehicle intelligent autonomous vehicles. The underlying architecture and most technical issues of autonomous driving have been solved, and the remaining 5% of long-tail problems have gradually become the key to restricting the development of autonomous driving. These problems include a variety of fragmented scenarios, extreme situations, and unpredictable human behavior. The "long tail" of edge scenarios in autonomous driving refers to edge cases in autonomous vehicles (AVs). Edge cases are possible scenarios with a low probability of occurrence. these rare events
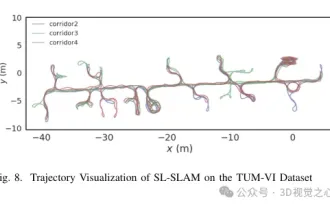
Written previously, today we discuss how deep learning technology can improve the performance of vision-based SLAM (simultaneous localization and mapping) in complex environments. By combining deep feature extraction and depth matching methods, here we introduce a versatile hybrid visual SLAM system designed to improve adaptation in challenging scenarios such as low-light conditions, dynamic lighting, weakly textured areas, and severe jitter. sex. Our system supports multiple modes, including extended monocular, stereo, monocular-inertial, and stereo-inertial configurations. In addition, it also analyzes how to combine visual SLAM with deep learning methods to inspire other research. Through extensive experiments on public datasets and self-sampled data, we demonstrate the superiority of SL-SLAM in terms of positioning accuracy and tracking robustness.
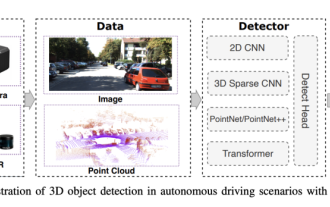
0.Written in front&& Personal understanding that autonomous driving systems rely on advanced perception, decision-making and control technologies, by using various sensors (such as cameras, lidar, radar, etc.) to perceive the surrounding environment, and using algorithms and models for real-time analysis and decision-making. This enables vehicles to recognize road signs, detect and track other vehicles, predict pedestrian behavior, etc., thereby safely operating and adapting to complex traffic environments. This technology is currently attracting widespread attention and is considered an important development area in the future of transportation. one. But what makes autonomous driving difficult is figuring out how to make the car understand what's going on around it. This requires that the three-dimensional object detection algorithm in the autonomous driving system can accurately perceive and describe objects in the surrounding environment, including their locations,
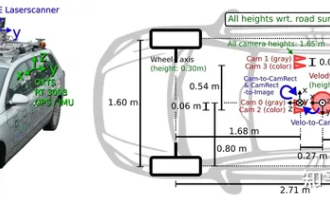
The first pilot and key article mainly introduces several commonly used coordinate systems in autonomous driving technology, and how to complete the correlation and conversion between them, and finally build a unified environment model. The focus here is to understand the conversion from vehicle to camera rigid body (external parameters), camera to image conversion (internal parameters), and image to pixel unit conversion. The conversion from 3D to 2D will have corresponding distortion, translation, etc. Key points: The vehicle coordinate system and the camera body coordinate system need to be rewritten: the plane coordinate system and the pixel coordinate system. Difficulty: image distortion must be considered. Both de-distortion and distortion addition are compensated on the image plane. 2. Introduction There are four vision systems in total. Coordinate system: pixel plane coordinate system (u, v), image coordinate system (x, y), camera coordinate system () and world coordinate system (). There is a relationship between each coordinate system,
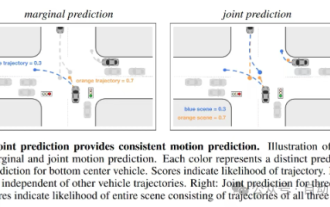
Trajectory prediction plays an important role in autonomous driving. Autonomous driving trajectory prediction refers to predicting the future driving trajectory of the vehicle by analyzing various data during the vehicle's driving process. As the core module of autonomous driving, the quality of trajectory prediction is crucial to downstream planning control. The trajectory prediction task has a rich technology stack and requires familiarity with autonomous driving dynamic/static perception, high-precision maps, lane lines, neural network architecture (CNN&GNN&Transformer) skills, etc. It is very difficult to get started! Many fans hope to get started with trajectory prediction as soon as possible and avoid pitfalls. Today I will take stock of some common problems and introductory learning methods for trajectory prediction! Introductory related knowledge 1. Are the preview papers in order? A: Look at the survey first, p
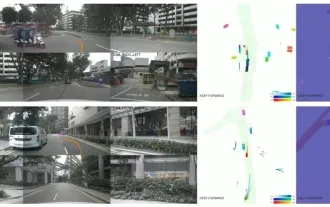
In the past month, due to some well-known reasons, I have had very intensive exchanges with various teachers and classmates in the industry. An inevitable topic in the exchange is naturally end-to-end and the popular Tesla FSDV12. I would like to take this opportunity to sort out some of my thoughts and opinions at this moment for your reference and discussion. How to define an end-to-end autonomous driving system, and what problems should be expected to be solved end-to-end? According to the most traditional definition, an end-to-end system refers to a system that inputs raw information from sensors and directly outputs variables of concern to the task. For example, in image recognition, CNN can be called end-to-end compared to the traditional feature extractor + classifier method. In autonomous driving tasks, input data from various sensors (camera/LiDAR

Original title: SIMPL: ASimpleandEfficientMulti-agentMotionPredictionBaselineforAutonomousDriving Paper link: https://arxiv.org/pdf/2402.02519.pdf Code link: https://github.com/HKUST-Aerial-Robotics/SIMPL Author unit: Hong Kong University of Science and Technology DJI Paper idea: This paper proposes a simple and efficient motion prediction baseline (SIMPL) for autonomous vehicles. Compared with traditional agent-cent
