


The new attention mechanism Meta makes large models more similar to the human brain, automatically filtering out information irrelevant to the task, thereby increasing the accuracy by 27%
Meta has conducted new research on the attention mechanism of large models
By adjusting the attention mechanism of the model and filtering out the interference of irrelevant information, the new mechanism further improves the accuracy of large models
Moreover, this mechanism does not require fine-tuning or training. Prompt alone can increase the accuracy of large models by 27%.
The author named this attention mechanism "System 2 Attention" (S2A), which originated from the 2002 Nobel Prize winner in Economics Daniel Card The psychological concept mentioned by Niemann in his best-selling book "Thinking, Fast and Slow" - "System 2" in the dual-system thinking model
The so-called System 2 refers to complex conscious reasoning, and The opposite is System 1, which is simple unconscious intuition.
S2A "adjusts" the attention mechanism in Transformer, and uses prompt words to make the overall thinking of the model closer to System 2
Some netizens described this mechanism as like giving AI adds a layer of "goggles".
In addition, the author also said in the title of the paper that not only large models, this thinking mode may also need to be learned by humans themselves.
So, how is this method implemented?
Avoid large models being "misled"
The Transformer architecture commonly used in traditional large models uses a soft attention mechanism - it gives each word (token) are assigned an attention value between 0 and 1.
The corresponding concept is the hard attention mechanism, which only focuses on one or certain subsets of the input sequence and is more commonly used in image processing.
The S2A mechanism can be understood as a combination of two modes - the core is still soft attention, but a "hard" screening process is added to it.
In terms of specific operation, S2A does not need to adjust the model itself, but uses prompt words to allow the model to remove "content that should not be paid attention to" before solving the problem.
In this way, the probability of a large model being misled when processing prompt words with subjective or irrelevant information can be reduced, thereby improving the model's reasoning ability and practical application value.
#We learned that the answers generated by large models are greatly affected by the prompt words. In order to improve accuracy, S2A decided to delete information that may cause interference
For example, if we ask the following question to a large model:
A city is a city in X state, surrounded by clusters of Surrounded by mountains and many parks, it is home to outstanding people. Many celebrities were born in City A.
Where was Mayor Y of City B in X State born?
At this time, the answers given by GPT and Llama are both city A mentioned in the question, but in fact Y’s birthplace is city C.
When initially asked, the model could have accurately answered city C. However, because city A appeared repeatedly in the prompt words, it attracted the model's "attention", resulting in the final The answer becomes A
Another situation where people come up with "possible answers" when asking a question.
In the M band, is there any singer who is also an actor? I think it might be A, but I'm not sure.
As a result, the big model starts to flatter the user, you are right , that’s right. But in fact this person is B.
Similarly, if you directly ask the person's identity, the model can answer accurately
It is because of this feature that the author thought about the new S2A mechanism , and designed a set of prompt words to refine user input
Let’s take a closer look at the effects before and after screening:
Q: Mary has three times as many candies as Megan. Mary took another 10 pieces of candy. Max owns 1,000 more books than Mary. If Megan has 5 pieces of candy, how many pieces does Mary have in total?
A: Max has 1,000 more books than Mary, so Max has 1,000 x 10 pieces of candy. We can make an equation:
1000 3x 10=3(5) 10
……
After S2A processing, the problem becomes the following situation:
Q: Mary has 3 times the number of candies as Megan. Mary took another 10 pieces of candy. If Megan has 5 pieces of candy, how many pieces does Mary have in total?
Question (Question is written directly in the prompt here): How many pieces of candy does Mary have in total?
Rewritten content: A: Meghan had 5 yuan, Mary had three times as much as Meghan, which was 15 yuan, and then took another 10 yuan, so there was a total of 25 yuan
The test results show that compared with general questions, the accuracy and objectivity of S2A after optimization are significantly enhanced, and the accuracy is close to that of manually designed streamlined prompts.
Specifically, S2A applied Llama 2-70B to a modified version of the TriviaQA dataset and improved accuracy by 27.9% from 62.8% to 80.3%. At the same time, the objectivity score also increased from 2.23 points (out of 5 points) to 3.82 points, even surpassing the effect of manual streamlined prompt words
In terms of robustness, the test results It shows that no matter whether the "interference information" is correct or wrong, positive or negative, S2A can allow the model to give more accurate and objective answers.
# Further experimental results of the S2A method show that it is necessary to remove interference information. Simply telling the model to ignore invalid information cannot significantly improve the accuracy, and may even lead to a decrease in accuracy.
On the other hand, as long as the original interference information is isolated, other aspects of S2A No adjustment will significantly reduce its effectiveness.
One More Thing
In fact, improving model performance through the adjustment of the attention mechanism has always been a hot topic in the academic community.
For example, the recently launched "Mistral" is the strongest 7B open source model, which uses a new grouped query attention model
Google's research team also proposed the HyperAttention attention mechanism. It solves the complexity problem of long text processing.
……
Regarding the “System 2” attention model adopted by Meta, AI godfather Bengio put forward a specific point of view:
Towards Artificial Intelligence General Intelligence The only way (AGI) is the transition from System 1 to System 2
Paper address: https://arxiv.org/abs/2311.11829
The above is the detailed content of The new attention mechanism Meta makes large models more similar to the human brain, automatically filtering out information irrelevant to the task, thereby increasing the accuracy by 27%. For more information, please follow other related articles on the PHP Chinese website!

Hot AI Tools

Undresser.AI Undress
AI-powered app for creating realistic nude photos

AI Clothes Remover
Online AI tool for removing clothes from photos.

Undress AI Tool
Undress images for free

Clothoff.io
AI clothes remover

Video Face Swap
Swap faces in any video effortlessly with our completely free AI face swap tool!

Hot Article

Hot Tools

Notepad++7.3.1
Easy-to-use and free code editor

SublimeText3 Chinese version
Chinese version, very easy to use

Zend Studio 13.0.1
Powerful PHP integrated development environment

Dreamweaver CS6
Visual web development tools

SublimeText3 Mac version
God-level code editing software (SublimeText3)

Hot Topics










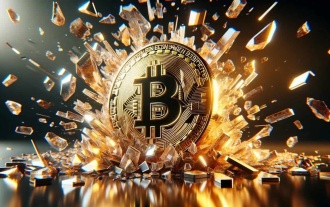
Bitcoin’s price ranges from $20,000 to $30,000. 1. Bitcoin’s price has fluctuated dramatically since 2009, reaching nearly $20,000 in 2017 and nearly $60,000 in 2021. 2. Prices are affected by factors such as market demand, supply, and macroeconomic environment. 3. Get real-time prices through exchanges, mobile apps and websites. 4. Bitcoin price is highly volatile, driven by market sentiment and external factors. 5. It has a certain relationship with traditional financial markets and is affected by global stock markets, the strength of the US dollar, etc. 6. The long-term trend is bullish, but risks need to be assessed with caution.
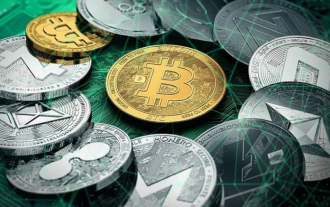
The top ten cryptocurrency trading platforms in the world include Binance, OKX, Gate.io, Coinbase, Kraken, Huobi Global, Bitfinex, Bittrex, KuCoin and Poloniex, all of which provide a variety of trading methods and powerful security measures.
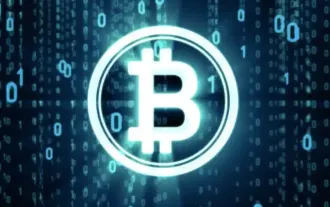
The top ten digital currency exchanges such as Binance, OKX, gate.io have improved their systems, efficient diversified transactions and strict security measures.
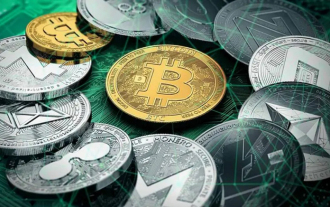
The top ten cryptocurrency exchanges in the world in 2025 include Binance, OKX, Gate.io, Coinbase, Kraken, Huobi, Bitfinex, KuCoin, Bittrex and Poloniex, all of which are known for their high trading volume and security.
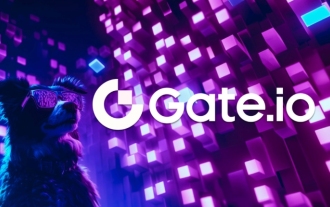
MeMebox 2.0 redefines crypto asset management through innovative architecture and performance breakthroughs. 1) It solves three major pain points: asset silos, income decay and paradox of security and convenience. 2) Through intelligent asset hubs, dynamic risk management and return enhancement engines, cross-chain transfer speed, average yield rate and security incident response speed are improved. 3) Provide users with asset visualization, policy automation and governance integration, realizing user value reconstruction. 4) Through ecological collaboration and compliance innovation, the overall effectiveness of the platform has been enhanced. 5) In the future, smart contract insurance pools, forecast market integration and AI-driven asset allocation will be launched to continue to lead the development of the industry.
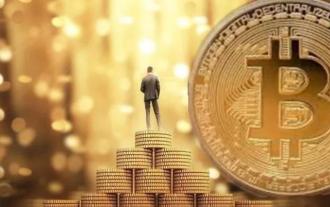
Recommended reliable digital currency trading platforms: 1. OKX, 2. Binance, 3. Coinbase, 4. Kraken, 5. Huobi, 6. KuCoin, 7. Bitfinex, 8. Gemini, 9. Bitstamp, 10. Poloniex, these platforms are known for their security, user experience and diverse functions, suitable for users at different levels of digital currency transactions
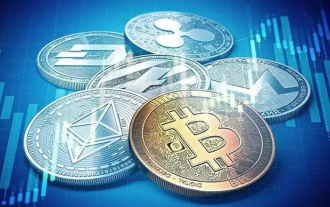
Currently ranked among the top ten virtual currency exchanges: 1. Binance, 2. OKX, 3. Gate.io, 4. Coin library, 5. Siren, 6. Huobi Global Station, 7. Bybit, 8. Kucoin, 9. Bitcoin, 10. bit stamp.
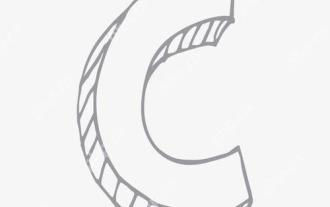
Measuring thread performance in C can use the timing tools, performance analysis tools, and custom timers in the standard library. 1. Use the library to measure execution time. 2. Use gprof for performance analysis. The steps include adding the -pg option during compilation, running the program to generate a gmon.out file, and generating a performance report. 3. Use Valgrind's Callgrind module to perform more detailed analysis. The steps include running the program to generate the callgrind.out file and viewing the results using kcachegrind. 4. Custom timers can flexibly measure the execution time of a specific code segment. These methods help to fully understand thread performance and optimize code.
