


Analysis of Python's underlying technology: how to implement neural networks
Analysis of Python's underlying technology: How to implement neural networks requires specific code examples
In the field of modern artificial intelligence, neural networks are one of the most commonly used and important technologies. . It simulates the working principle of the human brain and realizes complex tasks through the connection of multiple layers of neurons. As a powerful and easy-to-use programming language, Python provides extensive support and convenience for implementing neural networks. This article will delve into the underlying technology of neural networks and demonstrate its implementation through detailed code examples.
1. Structure of neural network
Neural network consists of three main parts: input layer, hidden layer and output layer. The input layer receives raw data or feature vectors, the hidden layer converts the input into a more abstract representation through a series of weights and activation functions, and finally the output layer produces the final prediction result.
2. Basic steps for implementing neural networks in Python
1. Import the necessary libraries
In Python, we can use NumPy for numerical calculations and Matplotlib for visualization operations . Therefore, these two libraries need to be imported first.
import numpy as np import matplotlib.pyplot as plt
2. Define the class of neural network
In the code, we implement this by defining a class of neural network. This class contains initialization function, forward propagation function and back propagation function.
class NeuralNetwork: def __init__(self, input_size, hidden_size, output_size): self.input_size = input_size self.hidden_size = hidden_size self.output_size = output_size self.W1 = np.random.randn(self.input_size, self.hidden_size) self.W2 = np.random.randn(self.hidden_size, self.output_size) def forward(self, X): self.z1 = np.dot(X, self.W1) self.a1 = self.sigmoid(self.z1) self.z2 = np.dot(self.a1, self.W2) self.output = self.sigmoid(self.z2) def backward(self, X, y): self.error = y - self.output self.delta_output = self.error * self.sigmoid_derivative(self.output) self.error_hidden = self.delta_output.dot(self.W2.T) self.delta_hidden = self.error_hidden * self.sigmoid_derivative(self.a1) self.W2 += self.a1.T.dot(self.delta_output) self.W1 += X.T.dot(self.delta_hidden)
3. Define the activation function and its derivatives
Commonly used activation functions include the sigmoid function and the ReLU function. The derivative of the activation function plays a key role in the backpropagation process. Below is sample code for these two functions.
def sigmoid(x): return 1 / (1 + np.exp(-x)) def sigmoid_derivative(x): return x * (1 - x) def relu(x): return np.maximum(0, x)
4. Training neural network model
During the training process, we need to prepare training data and target labels. By continuously calling the forward propagation and back propagation functions, the weight parameters are gradually optimized so that the output of the neural network is as close as possible to the target label.
X = np.array([[0, 0], [0, 1], [1, 0], [1, 1]]) y = np.array([[0], [1], [1], [0]]) nn = NeuralNetwork(2, 4, 1) for i in range(10000): nn.forward(X) nn.backward(X, y) print("Output after training:") print(nn.output)
5. Results visualization
Finally, we can use the Matplotlib library to visualize the prediction results.
plt.scatter(X[:, 0], X[:, 1], c=nn.output.flatten(), cmap='viridis') plt.title("Neural Network") plt.xlabel("Input1") plt.ylabel("Input2") plt.show()
By running the above code, we can see the classification results of the input data by the neural network.
This article shows how to use Python's underlying technology to implement neural networks through detailed code examples. Using these technologies, we are able to build and train a variety of complex neural network models to solve various artificial intelligence tasks. I hope this article will help you understand the underlying implementation of neural networks and improve your Python programming skills. Let’s explore the power of neural networks together!
The above is the detailed content of Analysis of Python's underlying technology: how to implement neural networks. For more information, please follow other related articles on the PHP Chinese website!

Hot AI Tools

Undresser.AI Undress
AI-powered app for creating realistic nude photos

AI Clothes Remover
Online AI tool for removing clothes from photos.

Undress AI Tool
Undress images for free

Clothoff.io
AI clothes remover

Video Face Swap
Swap faces in any video effortlessly with our completely free AI face swap tool!

Hot Article

Hot Tools

Notepad++7.3.1
Easy-to-use and free code editor

SublimeText3 Chinese version
Chinese version, very easy to use

Zend Studio 13.0.1
Powerful PHP integrated development environment

Dreamweaver CS6
Visual web development tools

SublimeText3 Mac version
God-level code editing software (SublimeText3)

Hot Topics










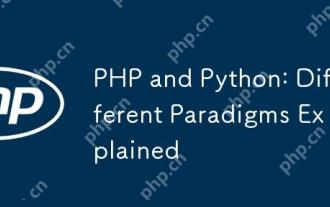
PHP is mainly procedural programming, but also supports object-oriented programming (OOP); Python supports a variety of paradigms, including OOP, functional and procedural programming. PHP is suitable for web development, and Python is suitable for a variety of applications such as data analysis and machine learning.
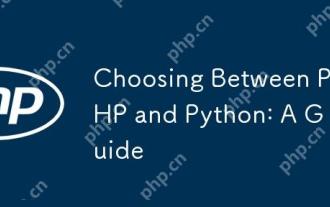
PHP is suitable for web development and rapid prototyping, and Python is suitable for data science and machine learning. 1.PHP is used for dynamic web development, with simple syntax and suitable for rapid development. 2. Python has concise syntax, is suitable for multiple fields, and has a strong library ecosystem.
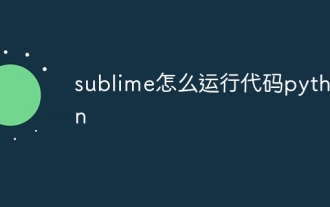
To run Python code in Sublime Text, you need to install the Python plug-in first, then create a .py file and write the code, and finally press Ctrl B to run the code, and the output will be displayed in the console.
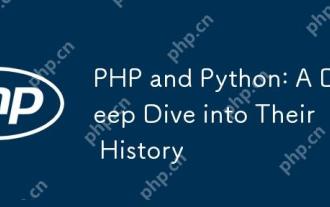
PHP originated in 1994 and was developed by RasmusLerdorf. It was originally used to track website visitors and gradually evolved into a server-side scripting language and was widely used in web development. Python was developed by Guidovan Rossum in the late 1980s and was first released in 1991. It emphasizes code readability and simplicity, and is suitable for scientific computing, data analysis and other fields.
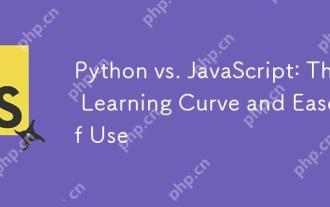
Python is more suitable for beginners, with a smooth learning curve and concise syntax; JavaScript is suitable for front-end development, with a steep learning curve and flexible syntax. 1. Python syntax is intuitive and suitable for data science and back-end development. 2. JavaScript is flexible and widely used in front-end and server-side programming.
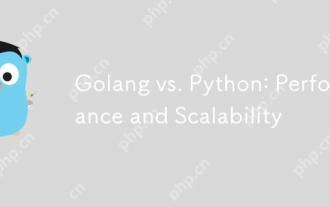
Golang is better than Python in terms of performance and scalability. 1) Golang's compilation-type characteristics and efficient concurrency model make it perform well in high concurrency scenarios. 2) Python, as an interpreted language, executes slowly, but can optimize performance through tools such as Cython.
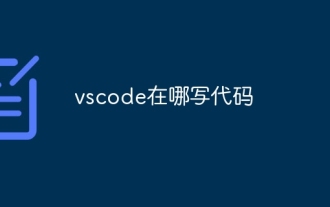
Writing code in Visual Studio Code (VSCode) is simple and easy to use. Just install VSCode, create a project, select a language, create a file, write code, save and run it. The advantages of VSCode include cross-platform, free and open source, powerful features, rich extensions, and lightweight and fast.
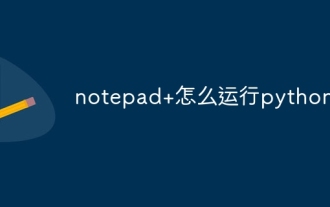
Running Python code in Notepad requires the Python executable and NppExec plug-in to be installed. After installing Python and adding PATH to it, configure the command "python" and the parameter "{CURRENT_DIRECTORY}{FILE_NAME}" in the NppExec plug-in to run Python code in Notepad through the shortcut key "F6".
