


How to process and forecast time series data using data analysis libraries in Python
How to use the data analysis library in Python to process and predict time series data
Time series data refers to data arranged in chronological order, which is characterized by temporal relevance and trends. In many fields, time series data analysis plays an important role, such as stock market forecast, weather forecast, sales forecast, etc. There are many powerful data analysis libraries in Python, such as NumPy, Pandas and Statsmodels, which can help us process and predict time series data. This article will introduce how to use these libraries in Python to process and forecast time series data, and provide specific code examples.
1. Data preprocessing
Before processing time series data, we usually need to preprocess the data, including data cleaning, processing missing values and outliers, etc. Pandas is a very useful library that simplifies the process of preprocessing time series data.
First, we need to import the required libraries:
import pandas as pd import numpy as np
Next, we can use Pandas to read the time series data, where the date column will be parsed into a DatetimeIndex type:
data = pd.read_csv('data.csv', parse_dates=['date'], index_col='date')
Once the data is loaded into the Pandas DataFrame, we can clean the data, handle missing values and outliers, etc. For example, we can use the dropna()
method to delete missing values:
data = data.dropna()
We can also use the fillna()
method to fill in missing values:
data = data.fillna(method='ffill')
2. Data Exploration
After processing time series data, we usually need to visualize and explore the data. This can help us understand characteristics such as trends, seasonality, and outliers in the data. Matplotlib and Seaborn are commonly used data visualization libraries in Python that can help us achieve this goal.
First, we need to import the required libraries:
import matplotlib.pyplot as plt import seaborn as sns
Next, we can use Matplotlib to draw a line chart of time series data:
plt.plot(data.index, data['value']) plt.xlabel('Date') plt.ylabel('Value') plt.title('Time series data') plt.show()
We can also use Seaborn Draw box plots of time series data:
sns.boxplot(data=data) plt.xlabel('Variable') plt.ylabel('Value') plt.title('Boxplot of time series data') plt.show()
3. Data modeling and prediction
After exploring time series data, we usually need to model and predict the data. Statsmodels is a commonly used statistical model library in Python that can help us implement time series modeling and forecasting.
First, we need to import the required libraries:
import statsmodels.api as sm
Next, we can use the ARIMA model in Statsmodels to model and forecast time series data. ARIMA (Autoregressive Moving Average Model) is a commonly used time series forecasting model that predicts future values by fitting autocorrelation and partial autocorrelation functions of time series data.
The following is an example code for using the ARIMA model for time series forecasting:
model = sm.tsa.ARIMA(data['value'], order=(1, 0, 0)) model_fit = model.fit(disp=False) forecast = model_fit.forecast(steps=10)
In the above code, we use the ARIMA(1, 0, 0) model to model the time series data , and then use the forecast()
method to predict the value of the next 10 time points.
4. Results evaluation and visualization
After performing time series prediction, we need to evaluate and visualize the results. This can help us judge the accuracy and reliability of the model.
We can use Pandas and Matplotlib to draw a line chart of the prediction results:
plt.plot(forecast.index, forecast.values, label='Forecast') plt.plot(data.index, data['value'], label='Actual') plt.xlabel('Date') plt.ylabel('Value') plt.title('Time series forecast') plt.legend() plt.show()
We can also use Pandas to calculate the root mean square error (RMSE) of the prediction results:
rmse = np.sqrt(np.mean((forecast.values - data['value'].values[-10:])**2)) print('RMSE: ', rmse)
The above is the basic process for processing and predicting time series data using the data analysis library in Python, including data preprocessing, data exploration, data modeling and prediction, and result evaluation and visualization. I hope the sample code in this article can help readers better understand and apply these libraries to process and predict time series data.
The above is the detailed content of How to process and forecast time series data using data analysis libraries in Python. For more information, please follow other related articles on the PHP Chinese website!

Hot AI Tools

Undresser.AI Undress
AI-powered app for creating realistic nude photos

AI Clothes Remover
Online AI tool for removing clothes from photos.

Undress AI Tool
Undress images for free

Clothoff.io
AI clothes remover

Video Face Swap
Swap faces in any video effortlessly with our completely free AI face swap tool!

Hot Article

Hot Tools

Notepad++7.3.1
Easy-to-use and free code editor

SublimeText3 Chinese version
Chinese version, very easy to use

Zend Studio 13.0.1
Powerful PHP integrated development environment

Dreamweaver CS6
Visual web development tools

SublimeText3 Mac version
God-level code editing software (SublimeText3)

Hot Topics










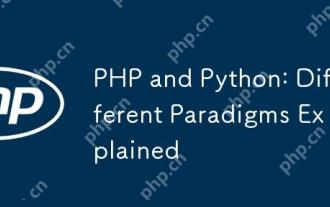
PHP is mainly procedural programming, but also supports object-oriented programming (OOP); Python supports a variety of paradigms, including OOP, functional and procedural programming. PHP is suitable for web development, and Python is suitable for a variety of applications such as data analysis and machine learning.
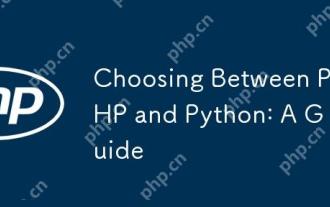
PHP is suitable for web development and rapid prototyping, and Python is suitable for data science and machine learning. 1.PHP is used for dynamic web development, with simple syntax and suitable for rapid development. 2. Python has concise syntax, is suitable for multiple fields, and has a strong library ecosystem.
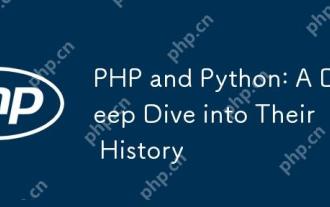
PHP originated in 1994 and was developed by RasmusLerdorf. It was originally used to track website visitors and gradually evolved into a server-side scripting language and was widely used in web development. Python was developed by Guidovan Rossum in the late 1980s and was first released in 1991. It emphasizes code readability and simplicity, and is suitable for scientific computing, data analysis and other fields.
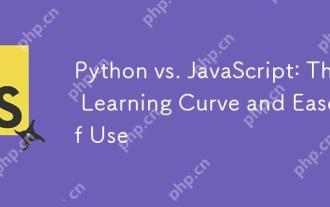
Python is more suitable for beginners, with a smooth learning curve and concise syntax; JavaScript is suitable for front-end development, with a steep learning curve and flexible syntax. 1. Python syntax is intuitive and suitable for data science and back-end development. 2. JavaScript is flexible and widely used in front-end and server-side programming.
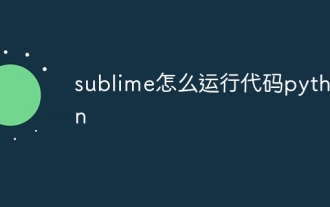
To run Python code in Sublime Text, you need to install the Python plug-in first, then create a .py file and write the code, and finally press Ctrl B to run the code, and the output will be displayed in the console.
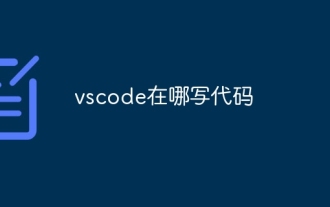
Writing code in Visual Studio Code (VSCode) is simple and easy to use. Just install VSCode, create a project, select a language, create a file, write code, save and run it. The advantages of VSCode include cross-platform, free and open source, powerful features, rich extensions, and lightweight and fast.
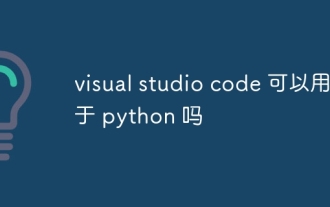
VS Code can be used to write Python and provides many features that make it an ideal tool for developing Python applications. It allows users to: install Python extensions to get functions such as code completion, syntax highlighting, and debugging. Use the debugger to track code step by step, find and fix errors. Integrate Git for version control. Use code formatting tools to maintain code consistency. Use the Linting tool to spot potential problems ahead of time.
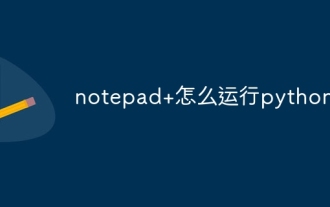
Running Python code in Notepad requires the Python executable and NppExec plug-in to be installed. After installing Python and adding PATH to it, configure the command "python" and the parameter "{CURRENT_DIRECTORY}{FILE_NAME}" in the NppExec plug-in to run Python code in Notepad through the shortcut key "F6".
