


Where there is AI, there is data, and where there is data, there is data storage.
Schumpeter said that innovation is to "establish a new production function", that is, to form a "new combination" of production factors and production conditions that has never been seen before. From this perspective, the combination of data, a new production factor, and the production conditions of artificial intelligence technology is a complete innovation, and the intelligent changes it will bring will be so surging.
In 2023, with the rise of large AI models, we seem to have seen a passionate picture of the AI era accelerating. According to the New Generation Artificial Intelligence Development Research Center of the Ministry of Science and Technology of China, the previously released "China Artificial Intelligence Large Model Map Research Report" shows that China has released 79 large models with a scale of more than 1 billion parameters, and the war of hundreds of models has become a foregone conclusion.
The popularity of large models has once again ignited the flame of the AI era, but at the same time it has also brought us new revelations: data is the "fuel" of artificial intelligence. The intensity and heat of this flame in the AI era completely depend on Whether the value of the data can be released.
In the AI era, the logical relationship between data storage and artificial intelligence must also be a mutually reinforcing and spiral upward model. This also means that the development of the AI era will also drive the development of the data storage industry.
01
The value enhancement of data to AI
As we all know, the significance of data to artificial intelligence is just like electric cars need batteries. If there is not enough storage data, the value that artificial intelligence can achieve will be extremely limited.
In the AI industry, there has always been a consensus of "garbage in, garbage out". That is to say, if there is no high-quality data input, no matter how advanced the algorithm or how huge the calculation is, No effort can produce high-quality results. Therefore, the main reason that determines the height of AI intelligence depends on the quality of data.
Of course, in addition to the quality of the data, the magnitude of the data also determines the height of what a large AI model can achieve.
Because the expressive ability of a model based on small-scale data is limited by the data size, it can only perform coarse-grained simulation and prediction, which is no longer applicable in situations where accuracy requirements are relatively high. If you want to further improve the accuracy of the model, you need to use massive data to generate relevant models.
This shows that the scale of data also determines the value of AI intelligence. Therefore, both the quality of the data and the order of magnitude of the data illustrate the role of data in artificial intelligence, which has become increasingly prominent with the deepening of AI applications.
This is easy to understand. When AI systems have more and better quality data, they can better predict future trends and generate more value.
For example, Tesla uses massive amounts of data to train its powerful artificial intelligence driving model, bringing an extraordinary experience to global users; while the Internet platform uses a large amount of user data to analyze with artificial intelligence, which can Customizing digital advertising based on user portraits is expected to bring global digital advertising revenue of up to US$679.8 billion in 2023.
These cases all prove the importance of data in increasing the value of artificial intelligence and even business model innovation.
02
The AI era drives the rapid development of data storage
This logic, if understood in reverse, is also true: the popularity of artificial intelligence has produced an even larger amount of data, which poses more challenges to the storage and processing of data
With the surge of global digitalization, data centers are being constructed at a geometric speed. A report by Schroders in 2023 shows that the power consumption of data centers will rapidly increase from 17 gigawatts in 2022 to 17 gigawatts in 2030. 35 GW, which means that the total number of data centers is expected to double in the next eight years.
Correspondingly, there is a surge in demand for data storage. "Fortune Business Insights" predicts that the global data storage market is expected to grow from US$247.32 billion in 2023 to US$777.98 billion in 2030. The market size is almost It will be tripled.
The comparison of these two data shows that the growth rate of data storage is much higher than the growth rate of the data center. We can also read two details from it:
First, precisely because of the arrival of the AI era, new requirements have been put forward for new data centers: data storage capabilities have become the focus of construction; second, the demand for data storage expansion has become the main driving force for data center construction.
From this, it is not difficult for us to draw a new conclusion: the development of the AI era will inevitably promote the rapid development of the data storage field. Core manufacturers in the data storage field will have a more promising future in the market, especially owners of core technologies represented by Seagate, who will capture the largest share of business growth amid the surge in market demand.
03
HDD’s unique position in the AI wave
In fact, there has been a common misconception in the industry in the past few years: it is believed that HDDs will be completely replaced by SSDs. But in fact, data center cloud service providers require a large number of high-density and large-capacity HDDs for cloud storage. Realize that the emergence of this market demand has made the growth rate of data center HDD products no less than that of SSD.
In fact, whether it is the big data demand represented by HDD or the fast data demand represented by SSD, the scale of demand is constantly expanding. Especially with the advent of the AI era, the demand for HDDs is increasing day by day.
The "Digital World—From Edge to Core" white paper sponsored by Seagate Technology and released by International Data Corporation (IDC) predicts that cloud data centers are becoming new enterprise data repositories. IDC predicts that by 2025, 49% of the world's stored data will be in public cloud environments. Because the artificial intelligence conversation mainly focuses on processors and cloud storage, while cloud storage relies more on HDD. Data created by artificial intelligence will require more HDDs to store in the future.
We can’t imagine that the hard drive industry has developed so fast in the past 45 years. In the 1980s, a 5.25-inch hard drive could only store 5 million bytes of data, and the latest technology in 2023 , Seagate has provided new products to some customers in July, with storage capacity of up to 30TB per block.
It is the leap in data storage technology that creates more imagination space for the industry. However, the demand for data storage due to the wave of artificial intelligence is growing at an order of magnitude. Perhaps the hard drives that are readily available today will become "scarce" products in the next few months.
Forbes predicted in a recent article that shipments of hard drive products will grow by 900% from 2020 to 2028. This means that if cloud service providers cannot purchase sufficient data storage space, they may even be unable to meet the needs of artificial intelligence growth.
Judging from the current market structure of mechanical hard drives, this is a market with high market concentration. Seagate, Western Digital and Toshiba dominate the world, with Seagate occupying the top spot. Their product innovation capabilities determine the development pace of the entire field of data storage to a certain extent, and will further influence the pace of upgrades and evolution in the AI era.
Where there is artificial intelligence, there is data. Where there is data, there is data storage. As the AI era is about to arrive, we also need to give data storage represented by mechanical hard drives a correct value positioning.
The above is the detailed content of Where there is AI, there is data, and where there is data, there is data storage.. For more information, please follow other related articles on the PHP Chinese website!

Hot AI Tools

Undresser.AI Undress
AI-powered app for creating realistic nude photos

AI Clothes Remover
Online AI tool for removing clothes from photos.

Undress AI Tool
Undress images for free

Clothoff.io
AI clothes remover

Video Face Swap
Swap faces in any video effortlessly with our completely free AI face swap tool!

Hot Article

Hot Tools

Notepad++7.3.1
Easy-to-use and free code editor

SublimeText3 Chinese version
Chinese version, very easy to use

Zend Studio 13.0.1
Powerful PHP integrated development environment

Dreamweaver CS6
Visual web development tools

SublimeText3 Mac version
God-level code editing software (SublimeText3)

Hot Topics










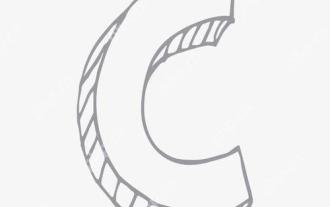
DMA in C refers to DirectMemoryAccess, a direct memory access technology, allowing hardware devices to directly transmit data to memory without CPU intervention. 1) DMA operation is highly dependent on hardware devices and drivers, and the implementation method varies from system to system. 2) Direct access to memory may bring security risks, and the correctness and security of the code must be ensured. 3) DMA can improve performance, but improper use may lead to degradation of system performance. Through practice and learning, we can master the skills of using DMA and maximize its effectiveness in scenarios such as high-speed data transmission and real-time signal processing.
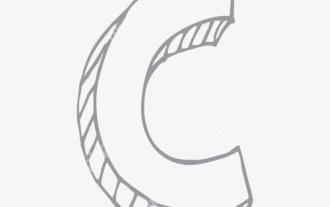
Using the chrono library in C can allow you to control time and time intervals more accurately. Let's explore the charm of this library. C's chrono library is part of the standard library, which provides a modern way to deal with time and time intervals. For programmers who have suffered from time.h and ctime, chrono is undoubtedly a boon. It not only improves the readability and maintainability of the code, but also provides higher accuracy and flexibility. Let's start with the basics. The chrono library mainly includes the following key components: std::chrono::system_clock: represents the system clock, used to obtain the current time. std::chron
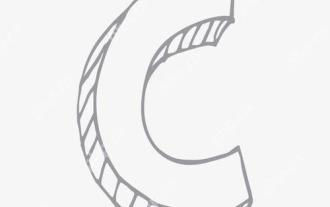
Measuring thread performance in C can use the timing tools, performance analysis tools, and custom timers in the standard library. 1. Use the library to measure execution time. 2. Use gprof for performance analysis. The steps include adding the -pg option during compilation, running the program to generate a gmon.out file, and generating a performance report. 3. Use Valgrind's Callgrind module to perform more detailed analysis. The steps include running the program to generate the callgrind.out file and viewing the results using kcachegrind. 4. Custom timers can flexibly measure the execution time of a specific code segment. These methods help to fully understand thread performance and optimize code.
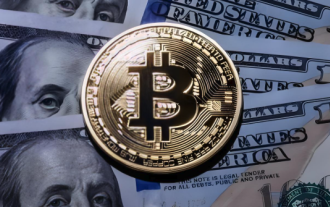
The built-in quantization tools on the exchange include: 1. Binance: Provides Binance Futures quantitative module, low handling fees, and supports AI-assisted transactions. 2. OKX (Ouyi): Supports multi-account management and intelligent order routing, and provides institutional-level risk control. The independent quantitative strategy platforms include: 3. 3Commas: drag-and-drop strategy generator, suitable for multi-platform hedging arbitrage. 4. Quadency: Professional-level algorithm strategy library, supporting customized risk thresholds. 5. Pionex: Built-in 16 preset strategy, low transaction fee. Vertical domain tools include: 6. Cryptohopper: cloud-based quantitative platform, supporting 150 technical indicators. 7. Bitsgap:
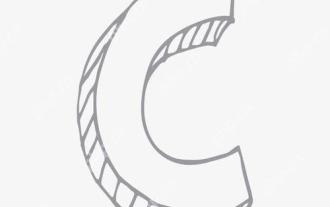
Handling high DPI display in C can be achieved through the following steps: 1) Understand DPI and scaling, use the operating system API to obtain DPI information and adjust the graphics output; 2) Handle cross-platform compatibility, use cross-platform graphics libraries such as SDL or Qt; 3) Perform performance optimization, improve performance through cache, hardware acceleration, and dynamic adjustment of the details level; 4) Solve common problems, such as blurred text and interface elements are too small, and solve by correctly applying DPI scaling.
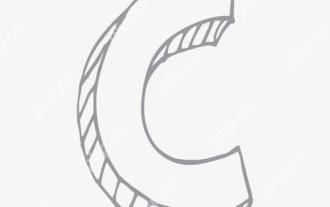
C performs well in real-time operating system (RTOS) programming, providing efficient execution efficiency and precise time management. 1) C Meet the needs of RTOS through direct operation of hardware resources and efficient memory management. 2) Using object-oriented features, C can design a flexible task scheduling system. 3) C supports efficient interrupt processing, but dynamic memory allocation and exception processing must be avoided to ensure real-time. 4) Template programming and inline functions help in performance optimization. 5) In practical applications, C can be used to implement an efficient logging system.
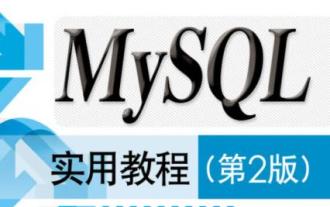
In MySQL, add fields using ALTERTABLEtable_nameADDCOLUMNnew_columnVARCHAR(255)AFTERexisting_column, delete fields using ALTERTABLEtable_nameDROPCOLUMNcolumn_to_drop. When adding fields, you need to specify a location to optimize query performance and data structure; before deleting fields, you need to confirm that the operation is irreversible; modifying table structure using online DDL, backup data, test environment, and low-load time periods is performance optimization and best practice.
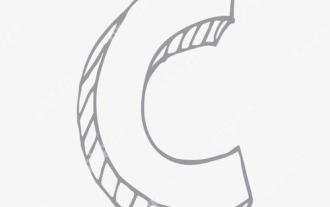
The main steps and precautions for using string streams in C are as follows: 1. Create an output string stream and convert data, such as converting integers into strings. 2. Apply to serialization of complex data structures, such as converting vector into strings. 3. Pay attention to performance issues and avoid frequent use of string streams when processing large amounts of data. You can consider using the append method of std::string. 4. Pay attention to memory management and avoid frequent creation and destruction of string stream objects. You can reuse or use std::stringstream.
