Scalability issues with machine learning models
Scalability issues of machine learning models require specific code examples
Abstract:
With the continuous increase of data scale and the continuous complexity of business requirements , Traditional machine learning models often cannot meet the requirements of large-scale data processing and fast response. Therefore, how to improve the scalability of machine learning models has become an important research direction. This article will introduce the scalability issue of machine learning models and give specific code examples.
- Introduction
The scalability of a machine learning model refers to the model's ability to maintain efficient running speed and accuracy in the face of large-scale data and high concurrency scenarios. Traditional machine learning models often need to traverse the entire data set for training and inference, which can lead to a waste of computing resources and a decrease in processing speed in large-scale data scenarios. Therefore, improving the scalability of machine learning models is a current research hotspot. - Model training based on distributed computing
In order to solve the problem of large-scale data training, distributed computing methods can be used to improve the training speed of the model. The specific code examples are as follows:
import tensorflow as tf from tensorflow import keras from tensorflow.keras import layers # 定义一个分布式的数据集 strategy = tf.distribute.experimental.MultiWorkerMirroredStrategy() # 创建模型 model = keras.Sequential([ layers.Dense(64, activation='relu'), layers.Dense(64, activation='relu'), layers.Dense(10, activation='softmax') ]) # 编译模型 model.compile(optimizer='adam', loss=tf.keras.losses.SparseCategoricalCrossentropy(from_logits=True), metrics=['accuracy']) # 使用分布式计算进行训练 with strategy.scope(): model.fit(train_dataset, epochs=10, validation_data=val_dataset)
The above code examples use TensorFlow’s distributed computing framework to train the model. By distributing training data to multiple computing nodes for calculation, the training speed can be greatly improved.
- Inference acceleration based on model compression
In the inference phase of the model, in order to improve the response speed of the model, the model compression method can be used to reduce the number of parameters and calculation amount of the model. Common model compression methods include pruning, quantization, and distillation. The following is a code example based on pruning:
import tensorflow as tf from tensorflow import keras from tensorflow.keras import layers # 创建模型 model = keras.Sequential([ layers.Dense(64, activation='relu'), layers.Dense(64, activation='relu'), layers.Dense(10, activation='softmax') ]) # 编译模型 model.compile(optimizer='adam', loss=tf.keras.losses.SparseCategoricalCrossentropy(from_logits=True), metrics=['accuracy']) # 训练模型 model.fit(train_dataset, epochs=10, validation_data=val_dataset) # 剪枝模型 pruned_model = tfmot.sparsity.keras.prune_low_magnitude(model) # 推理模型 pruned_model.predict(test_dataset)
The above code example uses the pruning method of TensorFlow Model Optimization Toolkit to reduce the number of parameters and calculation amount of the model. Inference through the pruned model can greatly improve the response speed of the model.
Conclusion:
This article introduces the scalability issue of machine learning models through specific code examples, and provides code examples from two aspects: distributed computing and model compression. Improving the scalability of machine learning models is of great significance to deal with large-scale data and high-concurrency scenarios. I hope the content of this article will be helpful to readers.
The above is the detailed content of Scalability issues with machine learning models. For more information, please follow other related articles on the PHP Chinese website!

Hot AI Tools

Undresser.AI Undress
AI-powered app for creating realistic nude photos

AI Clothes Remover
Online AI tool for removing clothes from photos.

Undress AI Tool
Undress images for free

Clothoff.io
AI clothes remover

Video Face Swap
Swap faces in any video effortlessly with our completely free AI face swap tool!

Hot Article

Hot Tools

Notepad++7.3.1
Easy-to-use and free code editor

SublimeText3 Chinese version
Chinese version, very easy to use

Zend Studio 13.0.1
Powerful PHP integrated development environment

Dreamweaver CS6
Visual web development tools

SublimeText3 Mac version
God-level code editing software (SublimeText3)

Hot Topics
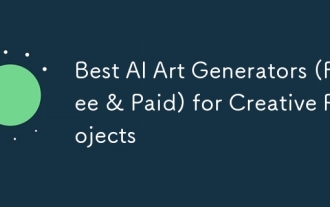
The article reviews top AI art generators, discussing their features, suitability for creative projects, and value. It highlights Midjourney as the best value for professionals and recommends DALL-E 2 for high-quality, customizable art.
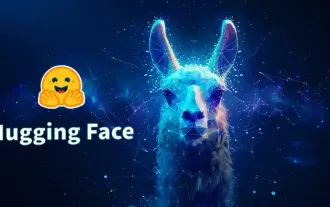
Meta's Llama 3.2: A Leap Forward in Multimodal and Mobile AI Meta recently unveiled Llama 3.2, a significant advancement in AI featuring powerful vision capabilities and lightweight text models optimized for mobile devices. Building on the success o
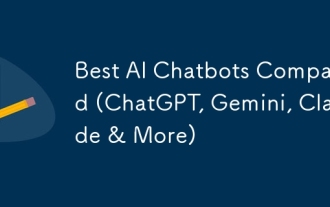
The article compares top AI chatbots like ChatGPT, Gemini, and Claude, focusing on their unique features, customization options, and performance in natural language processing and reliability.
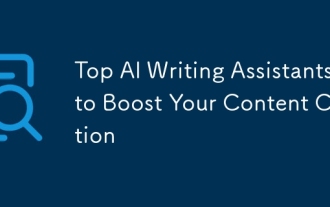
The article discusses top AI writing assistants like Grammarly, Jasper, Copy.ai, Writesonic, and Rytr, focusing on their unique features for content creation. It argues that Jasper excels in SEO optimization, while AI tools help maintain tone consist
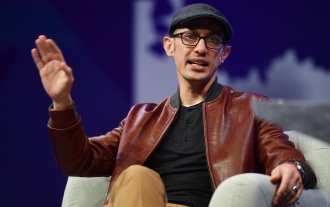
Shopify CEO Tobi Lütke's recent memo boldly declares AI proficiency a fundamental expectation for every employee, marking a significant cultural shift within the company. This isn't a fleeting trend; it's a new operational paradigm integrated into p
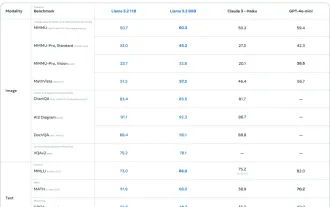
This week's AI landscape: A whirlwind of advancements, ethical considerations, and regulatory debates. Major players like OpenAI, Google, Meta, and Microsoft have unleashed a torrent of updates, from groundbreaking new models to crucial shifts in le
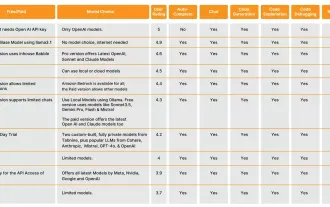
Hey there, Coding ninja! What coding-related tasks do you have planned for the day? Before you dive further into this blog, I want you to think about all your coding-related woes—better list those down. Done? – Let’
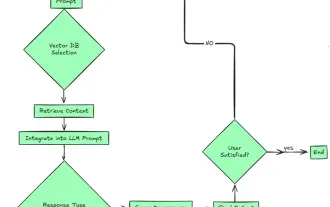
2024 witnessed a shift from simply using LLMs for content generation to understanding their inner workings. This exploration led to the discovery of AI Agents – autonomous systems handling tasks and decisions with minimal human intervention. Buildin
