


Research on solutions to data aggregation problems encountered in development using MongoDB technology
Title: Research on solutions to data aggregation problems under MongoDB technology
Abstract: This article will discuss the data aggregation problems encountered in development using MongoDB technology, and provide Provide specific solutions and code examples. MongoDB is an open source NoSQL database that can more effectively implement data aggregation operations and improve query efficiency. The article will expand from two aspects: aggregation pipeline and aggregation operator, providing readers with practical development guidance.
- Introduction
As a powerful NoSQL database, MongoDB provides flexible document storage functions. In practical applications, we often need to aggregate large amounts of data to meet complex query requirements. However, when performing data aggregation, developers often encounter problems such as data grouping, data filtering, and data calculation. To solve these problems, MongoDB provides powerful aggregation pipelines and aggregation operators. - Aggregation Pipeline
Aggregation pipeline is a concept used in MongoDB to handle data aggregation. It consists of a series of aggregation operations, which are executed in sequence and the results are passed to the next operation. The aggregation pipeline can implement various complex aggregation operations by using different aggregation operators. The following are several examples of commonly used aggregation operators:
(1) $match: used to filter documents that meet conditions.
For example, we need to filter out users who are 18 years or older:
db.users.aggregate([ { $match: { age: { $gte: 18 } } } ])
(2) $group: used to group documents.
For example, we need to count the number of users in each city:
db.users.aggregate([ { $group: { _id: "$city", count: { $sum: 1 } } } ])
(3) $sort: used to sort documents.
For example, we need to sort the users according to their age:
db.users.aggregate([ { $sort: { age: 1 } } ])
(4) $project: used to project the document.
For example, we only need to return the user's name and age:
db.users.aggregate([ { $project: { name: 1, age: 1 } } ])
By using these operators of the aggregation pipeline, we can implement functions such as data filtering, grouping, sorting, projection, etc.
- Solution Exploration
In practical applications, we often need to use multiple aggregation operators in combination to achieve more complex data aggregation requirements. The following is an example of a comprehensive application that shows how to use the aggregation pipeline to solve common data aggregation problems:
Suppose we have a collection of orders that stores user shopping records. Each document contains the field: userId ( User ID), amount (shopping amount), date (shopping date) and other information. We need to calculate the total shopping amount of each user in 2021.
const pipeline = [ { $match: { date: { $gte: new Date("2021-01-01"), $lt: new Date("2022-01-01") } } }, { $group: { _id: "$userId", totalAmount: { $sum: "$amount" } } } ]; db.orders.aggregate(pipeline);
In the above code, we first use the $match operator to filter out the shopping records in 2021, and then use the $group operator to group by user ID and calculate the total shopping amount of each user. Finally, by calling the db.orders.aggregate method to execute the aggregation pipeline, the total shopping amount of each user in 2021 can be obtained.
- Summary
This article first introduces the advantages and application scenarios of MongoDB as a NoSQL database through the introduction. Then, the problem of data aggregation in MongoDB is discussed in detail, and specific solutions and code examples are given. Through the flexible use of aggregation pipelines and aggregation operators, we can better process and analyze big data and meet complex data requirements.
Reference:
- MongoDB Documentation. "Aggregation Pipeline Operators". https://docs.mongodb.com/manual/reference/operator/aggregation-pipeline/
(Note: This article is a virtual creation, and the code examples are for reference only. Specific practical applications need to be adjusted according to the actual situation)
The above is the detailed content of Research on solutions to data aggregation problems encountered in development using MongoDB technology. For more information, please follow other related articles on the PHP Chinese website!

Hot AI Tools

Undresser.AI Undress
AI-powered app for creating realistic nude photos

AI Clothes Remover
Online AI tool for removing clothes from photos.

Undress AI Tool
Undress images for free

Clothoff.io
AI clothes remover

Video Face Swap
Swap faces in any video effortlessly with our completely free AI face swap tool!

Hot Article

Hot Tools

Notepad++7.3.1
Easy-to-use and free code editor

SublimeText3 Chinese version
Chinese version, very easy to use

Zend Studio 13.0.1
Powerful PHP integrated development environment

Dreamweaver CS6
Visual web development tools

SublimeText3 Mac version
God-level code editing software (SublimeText3)

Hot Topics
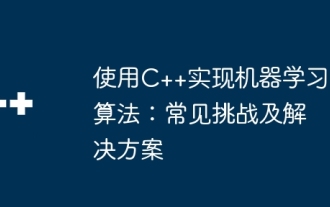
Common challenges faced by machine learning algorithms in C++ include memory management, multi-threading, performance optimization, and maintainability. Solutions include using smart pointers, modern threading libraries, SIMD instructions and third-party libraries, as well as following coding style guidelines and using automation tools. Practical cases show how to use the Eigen library to implement linear regression algorithms, effectively manage memory and use high-performance matrix operations.
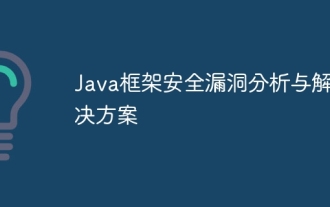
Analysis of Java framework security vulnerabilities shows that XSS, SQL injection and SSRF are common vulnerabilities. Solutions include: using security framework versions, input validation, output encoding, preventing SQL injection, using CSRF protection, disabling unnecessary features, setting security headers. In actual cases, the ApacheStruts2OGNL injection vulnerability can be solved by updating the framework version and using the OGNL expression checking tool.
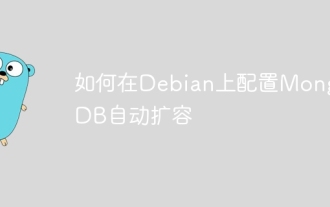
This article introduces how to configure MongoDB on Debian system to achieve automatic expansion. The main steps include setting up the MongoDB replica set and disk space monitoring. 1. MongoDB installation First, make sure that MongoDB is installed on the Debian system. Install using the following command: sudoaptupdatesudoaptinstall-ymongodb-org 2. Configuring MongoDB replica set MongoDB replica set ensures high availability and data redundancy, which is the basis for achieving automatic capacity expansion. Start MongoDB service: sudosystemctlstartmongodsudosys
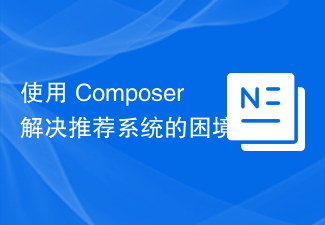
When developing an e-commerce website, I encountered a difficult problem: how to provide users with personalized product recommendations. Initially, I tried some simple recommendation algorithms, but the results were not ideal, and user satisfaction was also affected. In order to improve the accuracy and efficiency of the recommendation system, I decided to adopt a more professional solution. Finally, I installed andres-montanez/recommendations-bundle through Composer, which not only solved my problem, but also greatly improved the performance of the recommendation system. You can learn composer through the following address:
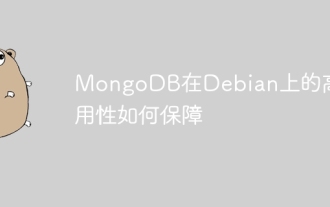
This article describes how to build a highly available MongoDB database on a Debian system. We will explore multiple ways to ensure data security and services continue to operate. Key strategy: ReplicaSet: ReplicaSet: Use replicasets to achieve data redundancy and automatic failover. When a master node fails, the replica set will automatically elect a new master node to ensure the continuous availability of the service. Data backup and recovery: Regularly use the mongodump command to backup the database and formulate effective recovery strategies to deal with the risk of data loss. Monitoring and Alarms: Deploy monitoring tools (such as Prometheus, Grafana) to monitor the running status of MongoDB in real time, and
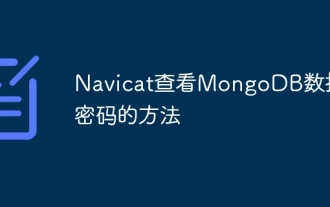
It is impossible to view MongoDB password directly through Navicat because it is stored as hash values. How to retrieve lost passwords: 1. Reset passwords; 2. Check configuration files (may contain hash values); 3. Check codes (may hardcode passwords).
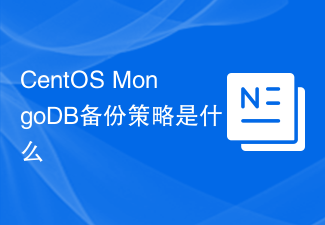
Detailed explanation of MongoDB efficient backup strategy under CentOS system This article will introduce in detail the various strategies for implementing MongoDB backup on CentOS system to ensure data security and business continuity. We will cover manual backups, timed backups, automated script backups, and backup methods in Docker container environments, and provide best practices for backup file management. Manual backup: Use the mongodump command to perform manual full backup, for example: mongodump-hlocalhost:27017-u username-p password-d database name-o/backup directory This command will export the data and metadata of the specified database to the specified backup directory.
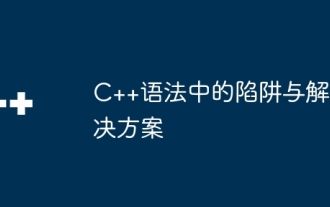
Pitfalls and Solutions in C++ Syntax C++ is a powerful programming language, but its syntax also makes it easy for programmers to fall into traps. This article will discuss some common pitfalls in C++ syntax and provide solutions to avoid or resolve them. Trap 1: Reference misuse problem: Using a pointer incorrectly as a reference. Code example: int&ref=*ptr;//Error: ptr is a pointer and cannot be dereferenced to a reference. Solution: Use a pointer to a pointer or dereference the pointer to a non-reference type. int*ptr2=&*ptr;//Use pointer pointer intval=*ptr;//Dereference to non-reference type Trap 2: Default behavior in conditional statements
