Algorithm selection issues in reinforcement learning
The problem of algorithm selection in reinforcement learning requires specific code examples
Reinforcement learning is a field of machine learning that learns optimal strategies through the interaction between the agent and the environment. . In reinforcement learning, choosing an appropriate algorithm is crucial to the learning effect. In this article, we explore algorithm selection issues in reinforcement learning and provide concrete code examples.
There are many algorithms to choose from in reinforcement learning, such as Q-Learning, Deep Q Network (DQN), Actor-Critic, etc. Choosing an appropriate algorithm depends on factors such as the complexity of the problem, the size of the state space and action space, and the availability of computing resources.
First, let’s look at a simple reinforcement learning problem, the maze problem. In this problem, the agent needs to find the shortest path from the starting point to the end point. We can use the Q-Learning algorithm to solve this problem. The following is a sample code:
import numpy as np # 创建迷宫 maze = np.array([ [1, 1, 1, 1, 1, 1, 1, 1, 1, 1], [1, 0, 0, 1, 0, 0, 0, 1, 0, 1], [1, 0, 0, 1, 0, 0, 0, 1, 0, 1], [1, 0, 0, 0, 0, 1, 1, 0, 0, 1], [1, 0, 1, 1, 1, 0, 0, 0, 0, 1], [1, 0, 0, 0, 1, 0, 0, 0, 1, 1], [1, 1, 1, 1, 1, 1, 1, 1, 1, 1] ]) # 定义Q表格 Q = np.zeros((maze.shape[0], maze.shape[1], 4)) # 设置超参数 epochs = 5000 epsilon = 0.9 alpha = 0.1 gamma = 0.6 # Q-Learning算法 for episode in range(epochs): state = (1, 1) # 设置起点 while state != (6, 8): # 终点 x, y = state possible_actions = np.where(maze[x, y] == 0)[0] # 可能的动作 action = np.random.choice(possible_actions) # 选择动作 next_state = None if action == 0: next_state = (x - 1, y) elif action == 1: next_state = (x + 1, y) elif action == 2: next_state = (x, y - 1) elif action == 3: next_state = (x, y + 1) reward = -1 if next_state == (6, 8) else 0 # 终点奖励为0,其他状态奖励为-1 Q[x, y, action] = (1 - alpha) * Q[x, y, action] + alpha * (reward + gamma * np.max(Q[next_state])) state = next_state print(Q)
The Q-Learning algorithm in the above code learns the optimal strategy by updating the Q table. The dimensions of the Q table correspond to the dimensions of the maze, where each element represents the benefit of the agent performing different actions in a specific state.
In addition to Q-Learning, other algorithms can also be used to solve more complex reinforcement learning problems. For example, when the state space and action space of the problem are large, deep reinforcement learning algorithms such as DQN can be used. The following is a simple DQN example code:
import torch import torch.nn as nn import torch.optim as optim import random # 创建神经网络 class DQN(nn.Module): def __init__(self, input_size, output_size): super(DQN, self).__init__() self.fc1 = nn.Linear(input_size, 16) self.fc2 = nn.Linear(16, output_size) def forward(self, x): x = torch.relu(self.fc1(x)) x = self.fc2(x) return x # 定义超参数 input_size = 4 output_size = 2 epochs = 1000 batch_size = 128 gamma = 0.99 epsilon = 0.2 # 创建经验回放内存 memory = [] capacity = 10000 # 创建神经网络和优化器 model = DQN(input_size, output_size) optimizer = optim.Adam(model.parameters(), lr=0.001) # 定义经验回放函数 def append_memory(state, action, next_state, reward): memory.append((state, action, next_state, reward)) if len(memory) > capacity: del memory[0] # 定义训练函数 def train(): if len(memory) < batch_size: return batch = random.sample(memory, batch_size) state_batch, action_batch, next_state_batch, reward_batch = zip(*batch) state_batch = torch.tensor(state_batch, dtype=torch.float) action_batch = torch.tensor(action_batch, dtype=torch.long) next_state_batch = torch.tensor(next_state_batch, dtype=torch.float) reward_batch = torch.tensor(reward_batch, dtype=torch.float) current_q = model(state_batch).gather(1, action_batch.unsqueeze(1)) next_q = model(next_state_batch).max(1)[0].detach() target_q = reward_batch + gamma * next_q loss = nn.MSELoss()(current_q, target_q.unsqueeze(1)) optimizer.zero_grad() loss.backward() optimizer.step() # DQN算法 for episode in range(epochs): state = env.reset() total_reward = 0 while True: if random.random() < epsilon: action = env.action_space.sample() else: action = model(torch.tensor(state, dtype=torch.float)).argmax().item() next_state, reward, done, _ = env.step(action) append_memory(state, action, next_state, reward) train() state = next_state total_reward += reward if done: break if episode % 100 == 0: print("Episode: ", episode, " Total Reward: ", total_reward) print("Training finished.")
The DQN algorithm in the above code uses a neural network to approximate the Q function, and trains the network by interacting in the environment to learn the optimal policy.
Through the above code examples, we can see that in reinforcement learning, different algorithms can be selected to solve the problem according to the characteristics of the problem. Q-Learning is suitable for problems where the state space is small and the action space is small, while DQN is suitable for complex problems where the state space and action space are large.
However, in practical applications, choosing an algorithm is not an easy task. Depending on the characteristics of the problem, we can try different algorithms and choose the most suitable algorithm based on the results. When selecting an algorithm, you also need to pay attention to factors such as the convergence, stability, and computational complexity of the algorithm, and make trade-offs based on specific needs.
In short, in reinforcement learning, algorithm selection is a key part. By choosing the algorithm rationally and tuning and improving it according to specific problems, we can achieve better reinforcement learning results in practical applications.
The above is the detailed content of Algorithm selection issues in reinforcement learning. For more information, please follow other related articles on the PHP Chinese website!

Hot AI Tools

Undresser.AI Undress
AI-powered app for creating realistic nude photos

AI Clothes Remover
Online AI tool for removing clothes from photos.

Undress AI Tool
Undress images for free

Clothoff.io
AI clothes remover

Video Face Swap
Swap faces in any video effortlessly with our completely free AI face swap tool!

Hot Article

Hot Tools

Notepad++7.3.1
Easy-to-use and free code editor

SublimeText3 Chinese version
Chinese version, very easy to use

Zend Studio 13.0.1
Powerful PHP integrated development environment

Dreamweaver CS6
Visual web development tools

SublimeText3 Mac version
God-level code editing software (SublimeText3)

Hot Topics
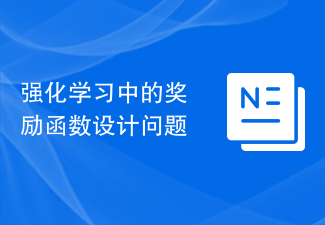
Reward function design issues in reinforcement learning Introduction Reinforcement learning is a method that learns optimal strategies through the interaction between an agent and the environment. In reinforcement learning, the design of the reward function is crucial to the learning effect of the agent. This article will explore reward function design issues in reinforcement learning and provide specific code examples. The role of the reward function and the target reward function are an important part of reinforcement learning and are used to evaluate the reward value obtained by the agent in a certain state. Its design helps guide the agent to maximize long-term fatigue by choosing optimal actions.
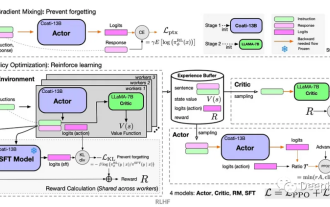
Reinforcement learning (RL) is a machine learning method that allows an agent to learn how to behave in its environment through trial and error. Agents are rewarded or punished for taking actions that lead to desired outcomes. Over time, the agent learns to take actions that maximize its expected reward. RL agents are typically trained using a Markov decision process (MDP), a mathematical framework for modeling sequential decision problems. MDP consists of four parts: State: a set of possible states of the environment. Action: A set of actions that an agent can take. Transition function: A function that predicts the probability of transitioning to a new state given the current state and action. Reward function: A function that assigns a reward to the agent for each conversion. The agent's goal is to learn a policy function,
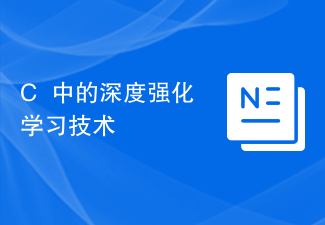
Deep reinforcement learning technology is a branch of artificial intelligence that has attracted much attention. It has won multiple international competitions and is also widely used in personal assistants, autonomous driving, game intelligence and other fields. In the process of realizing deep reinforcement learning, C++, as an efficient and excellent programming language, is especially important when hardware resources are limited. Deep reinforcement learning, as the name suggests, combines technologies from the two fields of deep learning and reinforcement learning. To simply understand, deep learning refers to learning features from data and making decisions by building a multi-layer neural network.
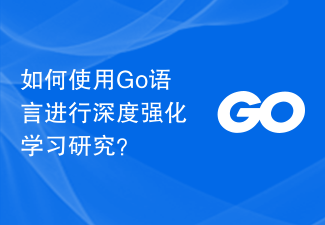
Deep Reinforcement Learning (DeepReinforcementLearning) is an advanced technology that combines deep learning and reinforcement learning. It is widely used in speech recognition, image recognition, natural language processing and other fields. As a fast, efficient and reliable programming language, Go language can provide help for deep reinforcement learning research. This article will introduce how to use Go language to conduct deep reinforcement learning research. 1. Install Go language and related libraries and start using Go language for deep reinforcement learning.
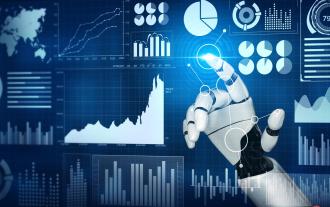
In this article, we will introduce training intelligent agents to control a dual-jointed robotic arm in the Reacher environment, a Unity-based simulation program developed using the UnityML-Agents toolkit. Our goal is to reach the target position with high accuracy, so here we can use the state-of-the-art DeepDeterministicPolicyGradient (DDPG) algorithm designed for continuous state and action spaces. Real-World Applications Robotic arms play critical roles in manufacturing, production facilities, space exploration, and search and rescue operations. It is very important to control the robot arm with high precision and flexibility. By employing reinforcement learning techniques, these robotic systems can be enabled to learn and adjust their behavior in real time.
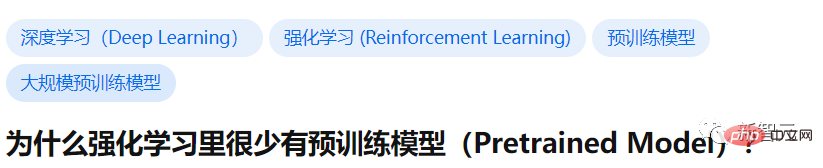
In current sequence modeling tasks, Transformer can be said to be the most powerful neural network architecture, and the pre-trained Transformer model can use prompts as conditions or in-context learning to adapt to different downstream tasks. The generalization ability of large-scale pre-trained Transformer models has been verified in multiple fields, such as text completion, language understanding, image generation, etc. Since last year, there has been relevant work proving that by treating offline reinforcement learning (offline RL) as a sequence prediction problem, the model can learn policies from offline data. But current approaches either learn policies from data that does not contain learning
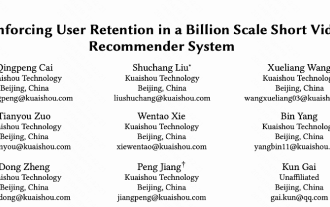
The core goal of the short video recommendation system is to drive DAU growth by improving user retention. Therefore, retention is one of the core business optimization indicators of each APP. However, retention is long-term feedback after multiple interactions between users and the system, and it is difficult to decompose it into a single item or a single list. Therefore, it is difficult to directly optimize retention using traditional point-wise and list-wise models. Reinforcement learning (RL) methods optimize long-term rewards by interacting with the environment, and are suitable for directly optimizing user retention. This work models the retention optimization problem as a Markov decision process (MDP) with infinite horizon request granularity. Each time the user requests the recommendation system to decide an action, it is used to aggregate multiple different short-term feedback estimates (watch duration,
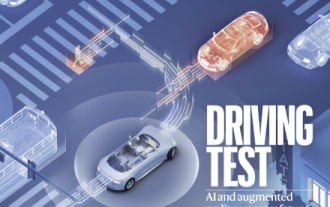
Introduce dense reinforcement learning and use AI to verify AI. Rapid advances in autonomous vehicle (AV) technology have us on the cusp of a transportation revolution on a scale not seen since the advent of the automobile a century ago. Autonomous driving technology has the potential to significantly improve traffic safety, mobility, and sustainability, and therefore has attracted the attention of industry, government agencies, professional organizations, and academic institutions. The development of self-driving cars has come a long way over the past 20 years, especially with the advent of deep learning. By 2015, companies were starting to announce that they would be mass-producing AVs by 2020. But so far, there is no level 4 AV available in the market
