


Semantic relationship recognition issues in text semantic understanding technology
The problem of semantic relationship identification in text semantic understanding technology requires specific code examples
With the continuous development of artificial intelligence technology, text semantic understanding has become a key factor in the field of natural language processing. plays an important role. Semantic relationship recognition is one of the key issues. In this article, we will explore the challenge of semantic relationship recognition and a deep learning-based solution, and give concrete code examples.
The identification of semantic relationships is one of the key links in text understanding. It involves identifying the types of relationships between entities in the text, such as "person relationships", "time relationships", "place relationships", etc. By accurately identifying semantic relationships, it can provide basic support for subsequent text analysis tasks, such as question and answer systems, information extraction, etc.
However, there are a series of challenges in semantic relationship recognition. First of all, the semantic relationship itself is diverse and complex. There may be multiple relationship types between different entities, and the same relationship type may have different expressions, such as "Li Ming is Mary's friend" and "Mary and Li Ming "Are friends" means the same relationship. Second, the identification of semantic relationships requires an in-depth understanding of the semantics of sentences, which is a challenge for traditional rule-based or statistical methods. Therefore, seeking a solution based on deep learning is an effective approach.
In order to solve the problem of semantic relationship recognition, we can use deep learning-based methods, combining word vector representation and neural network models. The following is a code example based on Convolutional Neural Network (CNN):
import torch import torch.nn as nn import torch.nn.functional as F class RelationClassifier(nn.Module): def __init__(self, embedding_dim, num_classes): super(RelationClassifier, self).__init__() self.embedding_dim = embedding_dim self.num_classes = num_classes self.embedding = nn.Embedding(vocab_size, embedding_dim) self.conv = nn.Conv1d(embedding_dim, 256, kernel_size=3, padding=1) self.fc = nn.Linear(256, num_classes) def forward(self, x): embedded = self.embedding(x) embedded = embedded.permute(0, 2, 1) conv_out = F.relu(self.conv(embedded)) pooled = F.max_pool1d(conv_out, conv_out.size(2)) flattened = pooled.view(pooled.size(0), -1) output = self.fc(flattened) return output # 定义模型超参数 embedding_dim = 100 num_classes = 10 vocab_size = 10000 # 初始化模型 model = RelationClassifier(embedding_dim, num_classes) # 加载训练数据,数据格式示例: # texts = ['李明是玛丽的朋友', '玛丽和李明是朋友'] # labels = [1, 1] train_data = load_data() # 定义损失函数和优化器 criterion = nn.CrossEntropyLoss() optimizer = torch.optim.Adam(model.parameters(), lr=0.001) # 开始训练 for epoch in range(num_epochs): total_loss = 0 for texts, labels in train_data: optimizer.zero_grad() inputs = preprocess(texts) outputs = model(inputs) loss = criterion(outputs, labels) loss.backward() optimizer.step() total_loss += loss.item() print('Epoch {}, Loss: {}'.format(epoch, total_loss))
In the above code example, we first define a model based on Convolutional Neural Network, which includes an embedding layer (embedding), convolutional layer and fully connected layer. Then, we loaded the training data and defined the loss function and optimizer. Next, we train the model using the training data and update the parameters based on the loss function and optimizer. Finally, we print the training loss for each epoch.
It should be noted that the above code example is only a simple demonstration. In actual applications, more complex model design and training processes may be required based on data and actual tasks.
To sum up, semantic relationship recognition is an important issue in text semantic understanding technology. The problem of semantic relationship recognition can be effectively solved through methods based on deep learning, such as convolutional neural networks. This article gives a code example based on deep learning, hoping to provide some help to readers in understanding and applying related technologies.
The above is the detailed content of Semantic relationship recognition issues in text semantic understanding technology. For more information, please follow other related articles on the PHP Chinese website!

Hot AI Tools

Undresser.AI Undress
AI-powered app for creating realistic nude photos

AI Clothes Remover
Online AI tool for removing clothes from photos.

Undress AI Tool
Undress images for free

Clothoff.io
AI clothes remover

Video Face Swap
Swap faces in any video effortlessly with our completely free AI face swap tool!

Hot Article

Hot Tools

Notepad++7.3.1
Easy-to-use and free code editor

SublimeText3 Chinese version
Chinese version, very easy to use

Zend Studio 13.0.1
Powerful PHP integrated development environment

Dreamweaver CS6
Visual web development tools

SublimeText3 Mac version
God-level code editing software (SublimeText3)

Hot Topics










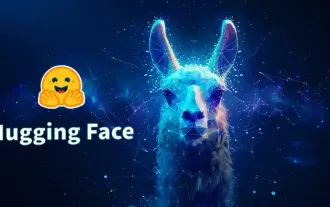
Meta's Llama 3.2: A Leap Forward in Multimodal and Mobile AI Meta recently unveiled Llama 3.2, a significant advancement in AI featuring powerful vision capabilities and lightweight text models optimized for mobile devices. Building on the success o
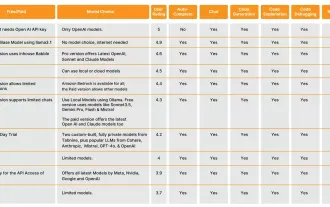
Hey there, Coding ninja! What coding-related tasks do you have planned for the day? Before you dive further into this blog, I want you to think about all your coding-related woes—better list those down. Done? – Let’
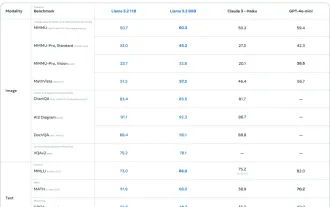
This week's AI landscape: A whirlwind of advancements, ethical considerations, and regulatory debates. Major players like OpenAI, Google, Meta, and Microsoft have unleashed a torrent of updates, from groundbreaking new models to crucial shifts in le
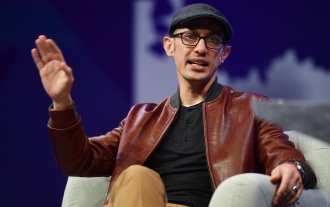
Shopify CEO Tobi Lütke's recent memo boldly declares AI proficiency a fundamental expectation for every employee, marking a significant cultural shift within the company. This isn't a fleeting trend; it's a new operational paradigm integrated into p
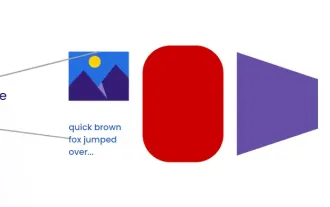
Introduction Imagine walking through an art gallery, surrounded by vivid paintings and sculptures. Now, what if you could ask each piece a question and get a meaningful answer? You might ask, “What story are you telling?
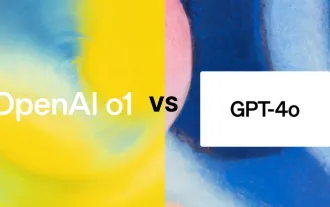
Introduction OpenAI has released its new model based on the much-anticipated “strawberry” architecture. This innovative model, known as o1, enhances reasoning capabilities, allowing it to think through problems mor
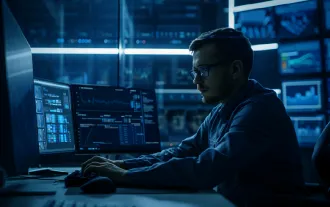
SQL's ALTER TABLE Statement: Dynamically Adding Columns to Your Database In data management, SQL's adaptability is crucial. Need to adjust your database structure on the fly? The ALTER TABLE statement is your solution. This guide details adding colu
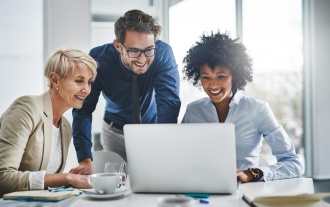
For those of you who might be new to my column, I broadly explore the latest advances in AI across the board, including topics such as embodied AI, AI reasoning, high-tech breakthroughs in AI, prompt engineering, training of AI, fielding of AI, AI re
