


Logical consistency issues in text generation based on semantic analysis
Logical consistency issues in text generation based on semantic analysis
In recent years, with the continuous development of natural language processing technology, text generation models have been widely used Machine translation, dialogue generation, sentiment analysis and other fields. However, in the text generation process, there is an important problem - the logical consistency problem. That is, the generated text must not only be grammatically and semantically correct, but also conform to logical rules, so that the generated sentences conform to the logic of human understanding.
The logical consistency problem is actually a very complex challenge. Traditional text generation models usually regard text generation as a sequence generation problem, which generates a series of words, but does not consider the logical relationship between words. The text generated in this way may lack logic, making the generated text difficult to understand or even wrong. For example, in machine translation, if the model translates "I like eating apples" into "I like eating glasses", it is obviously the result of a lack of logic.
In order to solve the problem of logical consistency, a common method is to combine semantic analysis technology. Semantic analysis is a technology that extracts semantic information from text and converts text into semantic representations. By converting the generated text into a semantic representation and comparing it with the target semantics, the logical consistency of the generated text can be effectively enhanced.
The following uses an example of dialogue generation to illustrate how to apply semantic analysis technology to solve the problem of logical consistency.
Suppose we have a conversation generation model that can generate responses from given questions. In the traditional model, the generated answers may be generated according to certain rules and patterns, but the logic of the answers is not checked.
We can use semantic analysis technology to analyze the generated answers. First, the generated answers are converted into semantic representations through the semantic analysis model. Then, the target semantic representation is compared with the generated semantic representation.
For example, if the question is "What kind of fruit do you like?" the generated answer is "I like to eat glasses." The answer is obviously wrong. Through semantic analysis, we can convert the answer "I like to eat glasses" into a semantic representation, such as "I like to eat apples". Then, compare it with the target semantic "I like to eat apples". If the matching degree between the two is higher than the set threshold, we can judge that the generated answer is reasonable. If the matching degree is lower than the threshold, it means that the generated answer lacks logic and may need to be regenerated.
The code example is as follows:
import semantics def generate_answer(question): answer = model.generate(question) semantic_answer = semantics.parse(answer) target_semantics = semantics.parse_target(question) similarity = semantic_similarity(semantic_answer, target_semantics) if similarity > threshold: return answer else: return generate_answer(question)
In this example, we first get the answer through the generation model, and then convert the answer into a semantic representation through the semantic analysis model. Next, we compare the target semantic representation with the generated semantic representation to obtain the similarity. If the similarity exceeds the set threshold, the answer is reasonable and can be returned; otherwise, we need to regenerate the answer.
By introducing semantic analysis technology, we can effectively solve the problem of logical consistency in text generation. However, it should be noted that semantic analysis technology itself also has certain challenges and limitations, such as ambiguity issues and accuracy of semantic representation. Therefore, in practical applications, we need to comprehensively consider different text generation models and semantic analysis technologies, as well as logical consistency detection methods suitable for specific task requirements, to improve the quality and accuracy of generated text.
In short, the logical consistency problem is an important challenge in text generation. By combining semantic analysis technology, we can improve the logical consistency of the generated text and effectively solve this problem. With the continuous development of natural language processing technology, I believe that the problem of logical consistency will be better solved, and text generation models will be able to generate human-understandable text more accurately and logically.
The above is the detailed content of Logical consistency issues in text generation based on semantic analysis. For more information, please follow other related articles on the PHP Chinese website!

Hot AI Tools

Undresser.AI Undress
AI-powered app for creating realistic nude photos

AI Clothes Remover
Online AI tool for removing clothes from photos.

Undress AI Tool
Undress images for free

Clothoff.io
AI clothes remover

Video Face Swap
Swap faces in any video effortlessly with our completely free AI face swap tool!

Hot Article

Hot Tools

Notepad++7.3.1
Easy-to-use and free code editor

SublimeText3 Chinese version
Chinese version, very easy to use

Zend Studio 13.0.1
Powerful PHP integrated development environment

Dreamweaver CS6
Visual web development tools

SublimeText3 Mac version
God-level code editing software (SublimeText3)

Hot Topics
![[Python NLTK] Semantic analysis to easily understand the meaning of text](https://img.php.cn/upload/article/000/465/014/170882647177099.jpg?x-oss-process=image/resize,m_fill,h_207,w_330)
The NLTK library provides a variety of tools and algorithms for semantic analysis, which can help us understand the meaning of text. Some of these tools and algorithms include: POStagging: POStagging is the process of tagging words into their parts of speech. Part-of-speech tagging can help us understand the relationship between words in a sentence and determine the subject, predicate, object and other components in the sentence. NLTK provides a variety of part-of-speech taggers that we can use to perform part-of-speech tagging on text. Stemming: Stemming is the process of reducing words to their roots. Stemming can help us find the relationship between words and determine the basic meaning of the words. NLTK provides a variety of stemmers, I
![[Python NLTK] Tutorial: Get started easily and have fun with natural language processing](https://img.php.cn/upload/article/000/465/014/170882721469561.jpg?x-oss-process=image/resize,m_fill,h_207,w_330)
1. Introduction to NLTK NLTK is a natural language processing toolkit for the Python programming language, created in 2001 by Steven Bird and Edward Loper. NLTK provides a wide range of text processing tools, including text preprocessing, word segmentation, part-of-speech tagging, syntactic analysis, semantic analysis, etc., which can help developers easily process natural language data. 2.NLTK installation NLTK can be installed through the following command: fromnltk.tokenizeimportWord_tokenizetext="Hello, world!Thisisasampletext."tokens=word_tokenize(te
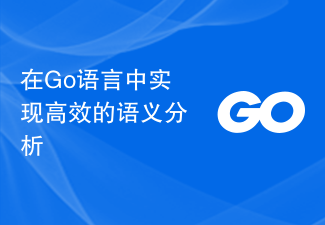
With the development of artificial intelligence and natural language processing, semantic analysis has become an increasingly important research field. In computer science, semantic analysis refers to converting natural language into machine-processable representations, which requires understanding the intent, emotion, context, etc. of the text. In this area, the efficiency and concurrency performance of the Go language have given us strong support. This article will introduce some technologies and methods to achieve efficient semantic analysis in Go language. To implement efficient semantic analysis in Go language using natural language processing library, we
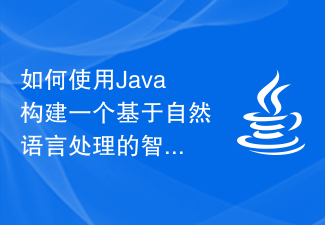
With the rapid development of artificial intelligence technology, natural language processing (Natural Language Processing) has been widely used in various fields. In the field of text generation, natural language processing technology can be used to automatically create high-quality text content, thereby improving work efficiency and text quality. This article will introduce how to use Java to build an intelligent text generation application based on natural language processing. 1. Understanding natural language processing technology Natural language processing technology refers to enabling computers to recognize, understand
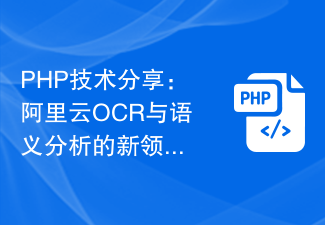
PHP technology sharing: Alibaba Cloud explores new areas of OCR and semantic analysis. With the continuous advancement of artificial intelligence technology and the widespread promotion of applications, text recognition (OCR) and semantic analysis are becoming increasingly important technical fields. As the leading cloud computing platform in China, Alibaba Cloud provides powerful OCR and semantic analysis APIs, providing developers with more convenient and faster development tools. This article will combine PHP language to deeply explore the application of Alibaba Cloud OCR and semantic analysis in actual projects, and attach corresponding code examples. 1. Alibaba Cloud
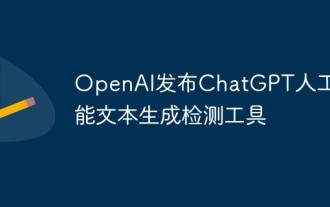
ChatGPTCChatGPT is an interactive natural language dialogue model launched by OpenAI in November 2022. OpenAI uses RLHF (Reinforcement Learning from Human Feedback) to train ChatGPT, which is the same method used by InstructGPT, but there are obvious differences in the data collection settings. Use supervised tuning (parameter) when training the initial model: Sessions provided by a human AI trainer. Trainers have access to model suggestions to help the trainer complete responses better. The newly generated conversational dataset is then mixed with the InstructGPT dataset to form a conversational format. ChatGPT caused a shock in the industry after its release, and was subsequently widely trialed by all parties, including students.
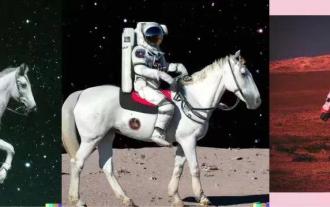
This article is reproduced from Lei Feng.com. If you need to reprint, please go to the official website of Lei Feng.com to apply for authorization. Since the DALL-E 2 came out, many people have considered AI capable of drawing realistic images to be a big step towards artificial general intelligence (AGI). OpenAI CEO Sam Altman once declared that "AGI is going to be wild" when DALL-E 2 was released, and the media are also exaggerating the significance of these systems for the progress of general intelligence. But is it really so? Gary Marcus, a well-known AI scholar and enthusiast who pours cold water on AI, expressed his "reservations." Recently, he suggested that when assessing progress in AGI, it is critical to
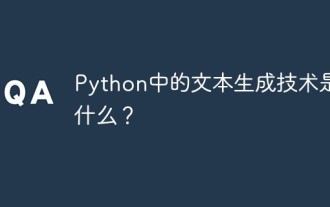
With the continuous development of machine learning and artificial intelligence technology, text generation technology has become an increasingly important technology. In this field, Python has become one of the most popular languages. Below, this article will explore text generation technology in Python. 1. Text generation technology in Python Text generation technology in Python mainly includes text classification, text clustering, text generation and text summarization. Among them, text generation is the most important technology. Text generation refers to the use of computers to generate text
