How to develop a simple machine learning system using MongoDB
How to use MongoDB to develop a simple machine learning system
With the development of artificial intelligence and machine learning, more and more developers are beginning to use MongoDB as their database selection. MongoDB is a popular NoSQL document database that provides powerful data management and query capabilities and is ideal for storing and processing machine learning data sets. This article will introduce how to use MongoDB to develop a simple machine learning system and give specific code examples.
- Install and configure MongoDB
First, we need to install and configure MongoDB. You can download the latest version from the official website (https://www.mongodb.com/) and follow the instructions to install it. After the installation is complete, you need to start the MongoDB service and create a database.
The method of starting the MongoDB service varies depending on the operating system. In most Linux systems, you can start the service with the following command:
sudo service mongodb start
In Windows systems, you can enter the following command in the command line:
mongod
To create a database, you can use MongoDB The command line tool mongo. Enter the following command at the command line:
mongo use mydb
- Import and process the data set
To develop a machine learning system, you first need to have a data set. MongoDB can store and process many types of data, including structured and unstructured data. Here, we take a simple iris dataset as an example.
We first save the iris data set as a csv file, and then use MongoDB's import tool mongodump to import the data. Enter the following command at the command line:
mongoimport --db mydb --collection flowers --type csv --headerline --file iris.csv
This will create a collection named flowers and import the iris dataset into it.
Now, we can use MongoDB’s query language to process the dataset. The following are some commonly used query operations:
- Query all data:
db.flowers.find()
- Query the value of a specific attribute:
db.flowers.find({ species: "setosa" })
- Query a certain range of attribute values:
db.flowers.find({ sepal_length: { $gt: 5.0, $lt: 6.0 } })
- Build a machine learning model
MongoDB provides many tools and APIs for operating data. We can use these tools and APIs to build our machine learning models. Here we will develop our machine learning system using the Python programming language and pymongo, the Python driver for MongoDB.
We first need to install pymongo. You can use the pip command to install:
pip install pymongo
Then, we can write Python code to connect to MongoDB and perform related operations. The following is a simple code example:
from pymongo import MongoClient # 连接MongoDB数据库 client = MongoClient() db = client.mydb # 查询数据集 flowers = db.flowers.find() # 打印结果 for flower in flowers: print(flower)
This code will connect to the database named mydb and query the data set as flowers. Then, print the query results.
- Data preprocessing and feature extraction
In machine learning, it is usually necessary to preprocess data and extract features. MongoDB can provide us with some functions to assist in these operations.
For example, we can use MongoDB's aggregation operation to calculate the statistical characteristics of the data. The following is a sample code:
from pymongo import MongoClient # 连接MongoDB数据库 client = MongoClient() db = client.mydb # 计算数据集的平均值 average_sepal_length = db.flowers.aggregate([ { "$group": { "_id": None, "avg_sepal_length": { "$avg": "$sepal_length" } }} ]) # 打印平均值 for result in average_sepal_length: print(result["avg_sepal_length"])
This code will calculate the average of the sepal_length attribute in the data set and print the result.
- Training and evaluating machine learning models
Finally, we can use MongoDB to save and load machine learning models for training and evaluation.
The following is a sample code:
from pymongo import MongoClient from sklearn.linear_model import LogisticRegression import pickle # 连接MongoDB数据库 client = MongoClient() db = client.mydb # 查询数据集 flowers = db.flowers.find() # 准备数据集 X = [] y = [] for flower in flowers: X.append([flower["sepal_length"], flower["sepal_width"], flower["petal_length"], flower["petal_width"]]) y.append(flower["species"]) # 训练模型 model = LogisticRegression() model.fit(X, y) # 保存模型 pickle.dump(model, open("model.pkl", "wb")) # 加载模型 loaded_model = pickle.load(open("model.pkl", "rb")) # 评估模型 accuracy = loaded_model.score(X, y) print(accuracy)
This code will load the data set from MongoDB and prepare training data. Then, use the logistic regression model to train and save the model locally. Finally, the model is loaded and evaluated using the dataset.
Summary:
This article introduces how to use MongoDB to develop a simple machine learning system and gives specific code examples. By combining the power of MongoDB with machine learning technology, we can develop more powerful and intelligent systems more efficiently. Hope this article helps you!
The above is the detailed content of How to develop a simple machine learning system using MongoDB. For more information, please follow other related articles on the PHP Chinese website!

Hot AI Tools

Undresser.AI Undress
AI-powered app for creating realistic nude photos

AI Clothes Remover
Online AI tool for removing clothes from photos.

Undress AI Tool
Undress images for free

Clothoff.io
AI clothes remover

Video Face Swap
Swap faces in any video effortlessly with our completely free AI face swap tool!

Hot Article

Hot Tools

Notepad++7.3.1
Easy-to-use and free code editor

SublimeText3 Chinese version
Chinese version, very easy to use

Zend Studio 13.0.1
Powerful PHP integrated development environment

Dreamweaver CS6
Visual web development tools

SublimeText3 Mac version
God-level code editing software (SublimeText3)

Hot Topics










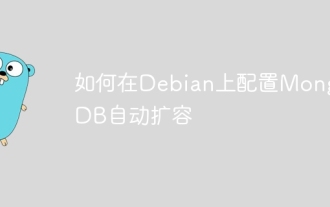
This article introduces how to configure MongoDB on Debian system to achieve automatic expansion. The main steps include setting up the MongoDB replica set and disk space monitoring. 1. MongoDB installation First, make sure that MongoDB is installed on the Debian system. Install using the following command: sudoaptupdatesudoaptinstall-ymongodb-org 2. Configuring MongoDB replica set MongoDB replica set ensures high availability and data redundancy, which is the basis for achieving automatic capacity expansion. Start MongoDB service: sudosystemctlstartmongodsudosys
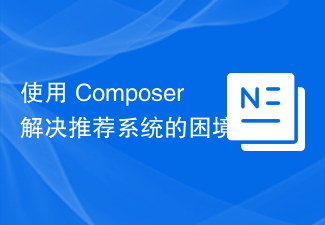
When developing an e-commerce website, I encountered a difficult problem: how to provide users with personalized product recommendations. Initially, I tried some simple recommendation algorithms, but the results were not ideal, and user satisfaction was also affected. In order to improve the accuracy and efficiency of the recommendation system, I decided to adopt a more professional solution. Finally, I installed andres-montanez/recommendations-bundle through Composer, which not only solved my problem, but also greatly improved the performance of the recommendation system. You can learn composer through the following address:
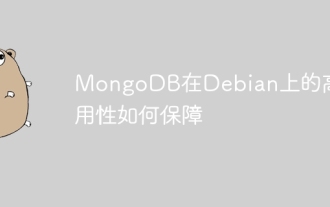
This article describes how to build a highly available MongoDB database on a Debian system. We will explore multiple ways to ensure data security and services continue to operate. Key strategy: ReplicaSet: ReplicaSet: Use replicasets to achieve data redundancy and automatic failover. When a master node fails, the replica set will automatically elect a new master node to ensure the continuous availability of the service. Data backup and recovery: Regularly use the mongodump command to backup the database and formulate effective recovery strategies to deal with the risk of data loss. Monitoring and Alarms: Deploy monitoring tools (such as Prometheus, Grafana) to monitor the running status of MongoDB in real time, and
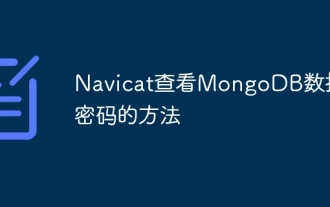
It is impossible to view MongoDB password directly through Navicat because it is stored as hash values. How to retrieve lost passwords: 1. Reset passwords; 2. Check configuration files (may contain hash values); 3. Check codes (may hardcode passwords).
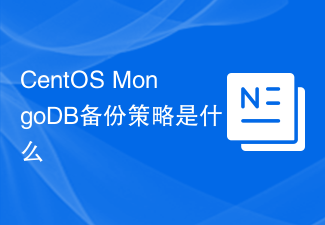
Detailed explanation of MongoDB efficient backup strategy under CentOS system This article will introduce in detail the various strategies for implementing MongoDB backup on CentOS system to ensure data security and business continuity. We will cover manual backups, timed backups, automated script backups, and backup methods in Docker container environments, and provide best practices for backup file management. Manual backup: Use the mongodump command to perform manual full backup, for example: mongodump-hlocalhost:27017-u username-p password-d database name-o/backup directory This command will export the data and metadata of the specified database to the specified backup directory.
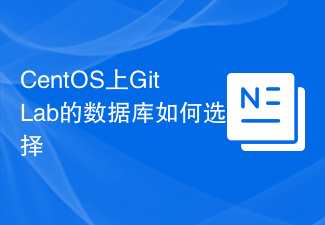
GitLab Database Deployment Guide on CentOS System Selecting the right database is a key step in successfully deploying GitLab. GitLab is compatible with a variety of databases, including MySQL, PostgreSQL, and MongoDB. This article will explain in detail how to select and configure these databases. Database selection recommendation MySQL: a widely used relational database management system (RDBMS), with stable performance and suitable for most GitLab deployment scenarios. PostgreSQL: Powerful open source RDBMS, supports complex queries and advanced features, suitable for handling large data sets. MongoDB: Popular NoSQL database, good at handling sea
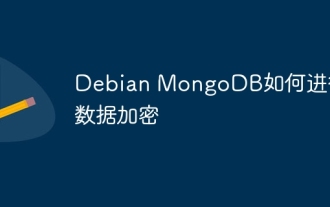
Encrypting MongoDB database on a Debian system requires following the following steps: Step 1: Install MongoDB First, make sure your Debian system has MongoDB installed. If not, please refer to the official MongoDB document for installation: https://docs.mongodb.com/manual/tutorial/install-mongodb-on-debian/Step 2: Generate the encryption key file Create a file containing the encryption key and set the correct permissions: ddif=/dev/urandomof=/etc/mongodb-keyfilebs=512
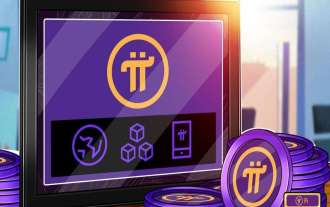
PiNetwork is about to launch PiBank, a revolutionary mobile banking platform! PiNetwork today released a major update on Elmahrosa (Face) PIMISRBank, referred to as PiBank, which perfectly integrates traditional banking services with PiNetwork cryptocurrency functions to realize the atomic exchange of fiat currencies and cryptocurrencies (supports the swap between fiat currencies such as the US dollar, euro, and Indonesian rupiah with cryptocurrencies such as PiCoin, USDT, and USDC). What is the charm of PiBank? Let's find out! PiBank's main functions: One-stop management of bank accounts and cryptocurrency assets. Support real-time transactions and adopt biospecies
