


Machine learning finds the right combination of hydrogen isotopes for future fusion power plants
Editor | Cabbage Leaf
Nuclear fusion, the power source of stars, is proposed as the future energy source of mankind and can provide clean and renewable energy, without the radioactive waste associated with current nuclear fission plants.
Like the fusion process that spills energy from the sun, future nuclear fusion facilities will violently slam together isotopes of hydrogen, the lightest element in the universe, in super-hot gas or "plasma" contained in powerful magnetic fields. In the body, helium is produced and energy is collected in the form of mass difference.
Before controllable nuclear fusion can actually occur on Earth, one thing scientists must know is what mixture of hydrogen isotopes to use - mainly "standard" hydrogen, which has a proton in its nucleus and a deuterium nucleus. There is one proton and one neutron in the nucleus, and there is one proton and two neutrons in the nucleus of tritium. Currently, this is done using spectra from prototype tokamak fusion devices, but this analysis can be very time-consuming.
In recent research, Mohammed Koubiti, associate professor at Aix-Marseille Université in France, conducted an evaluation to determine hydrogen isotope ratios for nuclear fusion plasma performance. He combined machine learning with plasma spectroscopy to conduct this research
The research is titled "Application of machine learning to spectroscopic line emission by hydrogen isotopes in fusion devices for isotopic ratio determination and prediction" , published in "The European Physical Journal D" on July 14, 2023.
Future power plants based on magnetic fusion reactions will certainly run on deuterium-tritium (DT) mixtures. However, due to tritium's radioactivity, the proportion of tritium in such mixtures must remain below thresholds set by regulatory agencies for obvious safety reasons.
Currently, tokamak and other devices dedicated to magnetic fusion research typically operate using pure hydrogen (H), deuterium (D) or HD gas mixtures. Although the European Joint Tokamak JET uses DT mixtures in rare cases, in order to comply with the regulatory limits on tritium content, it is necessary to accurately know the amount of tritium in the sealed container. "In terms of performance, the fusion power plant will Operate with a mixture of deuterium and tritium because they work best for fusion, but the amount of tritium must be controlled and tightly managed to comply with the limits imposed by regulatory agencies," Koubiti said. "In addition, it may be necessary to understand the behavior of tritium in real time. content, thereby optimizing the performance of the nuclear power plant."
One way to evaluate this is to determine the isotope ratio T/D T, which represents the tritium density as a percentage of the total plasma density in a deuterium-tritium plasma. Additionally, real-time control of tritium content may be necessary in fusion reactors operated by DT for safety or optimization purposes. In this case, real-time knowledge of the T/D T isotope ratio is required. Standard methods for determining isotope ratios do not allow for real-time application, but artificial intelligence can help.
"The ultimate goal is to avoid the use of spectroscopy, whose analysis is very time-consuming, and replace it with deep learning, or at least combine it with deep learning, to predict fusion Tritium content in plasma." Koubiti explains, "This study is just a step towards this goal. I am still using spectroscopy as a means that allows me to find other features that can be used by deep learning algorithms. Characteristics to predict changes in tritium content in fusion plasmas over time." Associate Professor Mohammed Koubiti discussed machine learning techniques (such as deep learning) combined with current measurements to be used to predict the future Some thoughts on the possibilities of fusion plasma devices. His paper focuses on machine learning in fusion plasma physics to make predictions before future experiments in facilities under construction such as ITER
More precisely, a brief introduction to a method based on the use of Hα A method to use simple spectral features of the /Dα line as input features for deep learning algorithms. The aim is to predict the isotope ratios of hydrogen-deuterium mixtures (HD plasmas) based on the input characteristics described above. Validation of the method was previously done using a set of 200 000 line spectra generated for typical conditions of a tokamak divertor.
Koubiti discusses the transition from generated to observed spectra and the possible extrapolation from HD to DT plasma discharges. Many issues that still need to be addressed are pointed out to achieve robust deep learning-based techniques capable of most accurately predicting physical quantities such as hydrogen isotope ratios in fusion plasmas operating with DT mixtures in future magnetic fusion-based power plants.
The next step is to complete the project by identifying non-spectral features that must be fed to any deep learning algorithm, Koubiti added. Subsequently, he plans to test these findings on several magnetic fusion devices, such as JET, ASDEX-Upgrade or tokamak devices such as WEST and DIII-D, as well as stellarator plasma devices that rely on external magnets to confine the plasma.
Word of mouth mentioned that I also plan to expand the application scope of deep learning technology to the field of non-plasma spectroscopy
Please click the following link to view the paper: https://link.springer.com/article /10.1140/epjd/s10053-023-00719-0
Related reports: https://phys.org/news/2023-09-Machine extraction of hydrogen isotopes-future nuclear energy.html
The above is the detailed content of Machine learning finds the right combination of hydrogen isotopes for future fusion power plants. For more information, please follow other related articles on the PHP Chinese website!

Hot AI Tools

Undresser.AI Undress
AI-powered app for creating realistic nude photos

AI Clothes Remover
Online AI tool for removing clothes from photos.

Undress AI Tool
Undress images for free

Clothoff.io
AI clothes remover

Video Face Swap
Swap faces in any video effortlessly with our completely free AI face swap tool!

Hot Article

Hot Tools

Notepad++7.3.1
Easy-to-use and free code editor

SublimeText3 Chinese version
Chinese version, very easy to use

Zend Studio 13.0.1
Powerful PHP integrated development environment

Dreamweaver CS6
Visual web development tools

SublimeText3 Mac version
God-level code editing software (SublimeText3)

Hot Topics










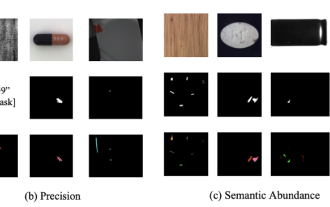
In modern manufacturing, accurate defect detection is not only the key to ensuring product quality, but also the core of improving production efficiency. However, existing defect detection datasets often lack the accuracy and semantic richness required for practical applications, resulting in models unable to identify specific defect categories or locations. In order to solve this problem, a top research team composed of Hong Kong University of Science and Technology Guangzhou and Simou Technology innovatively developed the "DefectSpectrum" data set, which provides detailed and semantically rich large-scale annotation of industrial defects. As shown in Table 1, compared with other industrial data sets, the "DefectSpectrum" data set provides the most defect annotations (5438 defect samples) and the most detailed defect classification (125 defect categories
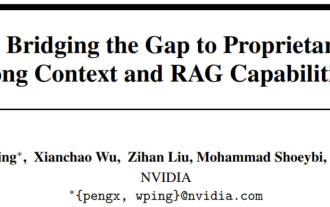
The open LLM community is an era when a hundred flowers bloom and compete. You can see Llama-3-70B-Instruct, QWen2-72B-Instruct, Nemotron-4-340B-Instruct, Mixtral-8x22BInstruct-v0.1 and many other excellent performers. Model. However, compared with proprietary large models represented by GPT-4-Turbo, open models still have significant gaps in many fields. In addition to general models, some open models that specialize in key areas have been developed, such as DeepSeek-Coder-V2 for programming and mathematics, and InternVL for visual-language tasks.
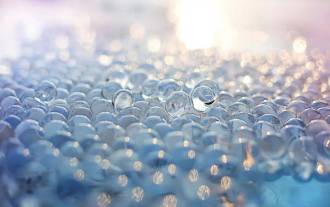
Editor |KX To this day, the structural detail and precision determined by crystallography, from simple metals to large membrane proteins, are unmatched by any other method. However, the biggest challenge, the so-called phase problem, remains retrieving phase information from experimentally determined amplitudes. Researchers at the University of Copenhagen in Denmark have developed a deep learning method called PhAI to solve crystal phase problems. A deep learning neural network trained using millions of artificial crystal structures and their corresponding synthetic diffraction data can generate accurate electron density maps. The study shows that this deep learning-based ab initio structural solution method can solve the phase problem at a resolution of only 2 Angstroms, which is equivalent to only 10% to 20% of the data available at atomic resolution, while traditional ab initio Calculation
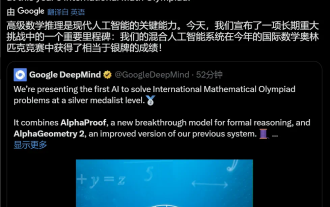
For AI, Mathematical Olympiad is no longer a problem. On Thursday, Google DeepMind's artificial intelligence completed a feat: using AI to solve the real question of this year's International Mathematical Olympiad IMO, and it was just one step away from winning the gold medal. The IMO competition that just ended last week had six questions involving algebra, combinatorics, geometry and number theory. The hybrid AI system proposed by Google got four questions right and scored 28 points, reaching the silver medal level. Earlier this month, UCLA tenured professor Terence Tao had just promoted the AI Mathematical Olympiad (AIMO Progress Award) with a million-dollar prize. Unexpectedly, the level of AI problem solving had improved to this level before July. Do the questions simultaneously on IMO. The most difficult thing to do correctly is IMO, which has the longest history, the largest scale, and the most negative
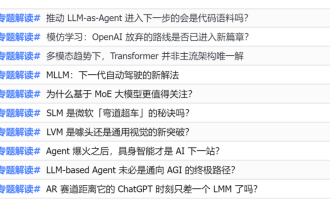
In 2023, almost every field of AI is evolving at an unprecedented speed. At the same time, AI is constantly pushing the technological boundaries of key tracks such as embodied intelligence and autonomous driving. Under the multi-modal trend, will the situation of Transformer as the mainstream architecture of AI large models be shaken? Why has exploring large models based on MoE (Mixed of Experts) architecture become a new trend in the industry? Can Large Vision Models (LVM) become a new breakthrough in general vision? ...From the 2023 PRO member newsletter of this site released in the past six months, we have selected 10 special interpretations that provide in-depth analysis of technological trends and industrial changes in the above fields to help you achieve your goals in the new year. be prepared. This interpretation comes from Week50 2023
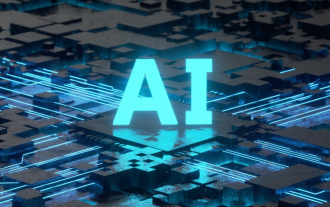
Editor |ScienceAI Question Answering (QA) data set plays a vital role in promoting natural language processing (NLP) research. High-quality QA data sets can not only be used to fine-tune models, but also effectively evaluate the capabilities of large language models (LLM), especially the ability to understand and reason about scientific knowledge. Although there are currently many scientific QA data sets covering medicine, chemistry, biology and other fields, these data sets still have some shortcomings. First, the data form is relatively simple, most of which are multiple-choice questions. They are easy to evaluate, but limit the model's answer selection range and cannot fully test the model's ability to answer scientific questions. In contrast, open-ended Q&A

Editor | KX Retrosynthesis is a critical task in drug discovery and organic synthesis, and AI is increasingly used to speed up the process. Existing AI methods have unsatisfactory performance and limited diversity. In practice, chemical reactions often cause local molecular changes, with considerable overlap between reactants and products. Inspired by this, Hou Tingjun's team at Zhejiang University proposed to redefine single-step retrosynthetic prediction as a molecular string editing task, iteratively refining the target molecular string to generate precursor compounds. And an editing-based retrosynthetic model EditRetro is proposed, which can achieve high-quality and diverse predictions. Extensive experiments show that the model achieves excellent performance on the standard benchmark data set USPTO-50 K, with a top-1 accuracy of 60.8%.
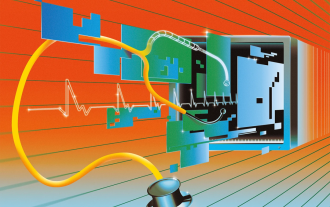
Editor | ScienceAI Based on limited clinical data, hundreds of medical algorithms have been approved. Scientists are debating who should test the tools and how best to do so. Devin Singh witnessed a pediatric patient in the emergency room suffer cardiac arrest while waiting for treatment for a long time, which prompted him to explore the application of AI to shorten wait times. Using triage data from SickKids emergency rooms, Singh and colleagues built a series of AI models that provide potential diagnoses and recommend tests. One study showed that these models can speed up doctor visits by 22.3%, speeding up the processing of results by nearly 3 hours per patient requiring a medical test. However, the success of artificial intelligence algorithms in research only verifies this
