


ACM MM 2023 | DiffBFR: Noise suppression face restoration method jointly proposed by Meitu & Chinese University of Science and Technology
The goal of Blind Face Restoration (BFR) is to restore high-quality face images from low-quality face images. This is an important task in the field of computer vision and graphics, and is widely used in various scenarios such as surveillance image restoration, old photo restoration, and facial image super-resolution.
However, this task is very challenging. nature, because the degradation of uncertainty will damage the quality of the image and even lead to the loss of image information, such as blur, noise, downsampling and compression artifacts. Previous BFR methods usually rely on generative adversarial networks (GAN) to solve these problems by designing various face-specific priors, including generative priors, reference priors, and geometric priors. Although these methods have reached the state-of-the-art level, they still cannot fully achieve the goal of obtaining realistic textures while restoring details
In the image restoration process, the datasets of face images are usually scattered in high-dimensional space , and the characteristic dimension of the distribution takes the form of a long-tail distribution. Different from the long-tail distribution of image classification tasks, the long-tail regional features in image restoration refer to attributes that have a small impact on identity but a large impact on visual effects, such as moles, wrinkles, and tones, etc.
According to the simplicity shown in Figure 1, in order not to change the original meaning, the experimental results need to be rewritten into Chinese. We can find that the past GAN-based method has obvious problems when processing the head and tail samples of long-tail distribution at the same time. Repair the image Over-smoothing and loss of detail may occur. The method based on Diffusion Probistic Models (DPM) can better fit the long-tail distribution and retain the tail characteristics while fitting the real data distribution
The content that needs to be rewritten is: GAN-based and DPM-based testing on long-tail issues
Meitu Imaging Research Institute (MT Lab) and the Chinese Academy of Sciences University researchers jointly proposed a new blind face image repair method, DiffBFR, which is based on DPM technology and successfully restored blind face images, repairing low-quality (LQ) face images to high-quality (HQ). A clear image of
What needs to be rewritten is: Paper link: https://arxiv.org/abs/2305.04517
This study explores The adaptability of two generative models, Generative Adversarial Network (GAN) and Deep Partial Model (DPM), in dealing with long-tail problems. By designing an appropriate face restoration module, more accurate detailed information can be obtained, thereby reducing the over-smoothing of the face that may occur in generative methods and improving the precision and accuracy of restoration. This research paper has been accepted by ACM MM 2023
DPM-based blind face image repair method - DiffBFR
The study found that the diffusion model is good at avoiding training mode collapse and fitting It is better than the GAN method in generating long-tail distribution. Therefore, DiffBFR chooses to use the diffusion probability model to enhance the embedding of face prior information, and uses this as the basic framework to choose DPM as the solution. This is because the diffusion model has the powerful ability to produce high-quality images within any distribution range
In order to solve the long-tail distribution of features on the face dataset found in the paper and the over-smoothing problem based on GAN methods in the past, This study explores a reasonable design to better fit the approximate long-tail distribution and overcome the over-smoothing problem in the repair process. Through a simple experiment of GAN and DPM with the same parameter size on the MNIST data set (Figure 1), the study found that the DPM method can reasonably fit the long-tail distribution, while GAN pays too much attention to the head features and ignores the tail features. As a result, tail features cannot be generated. Therefore, DPM is chosen as a solution to BFR
By introducing two intermediate variables, DiffBFR proposes two specific repair modules. The design adopts a two-stage approach, first recovering identity information from LQ images, and then enhancing texture details based on the distribution of real faces. This design consists of two key parts:
(1) Identity Restoration Module (IRM):
The purpose of this module is to retain the Face details. At the same time, a truncated sampling method is proposed, which replaces the denoising method using pure Gaussian random distribution in the reverse process by adding part of the noise to the low-quality image. The paper theoretically proves that this change shrinks the theoretical evidence lower bound (ELBO) of DPM, thereby restoring more original details. Based on theoretical proofs, two cascaded conditional diffusion models with different input sizes are introduced to enhance the sampling effect and reduce the training difficulty of directly generating high-resolution images. At the same time, it is further proved that the higher the quality of the conditional input, the closer it is to the real data distribution, and the more accurate the restored image is. This is also the reason why DiffBFR first restores low-resolution images
(2) Texture Enhancement Module (TEM):
The method used to texture polish images is to introduce an unconditional diffusion model. This model is completely independent of low-quality images, further making the restored results closer to real image data. The paper theoretically proves that an unconditional diffusion model trained on purely high-quality images contributes to the correct distribution of the output image in pixel-level space. That is, after using this model, the distribution of inpainted images has a lower FID than before using it, and is overall more similar to the distribution of high-quality images. Specifically, the identity information is retained by truncating the sampling at the time step, and the pixel-level texture is polished.
The sampling inference steps of DiffBFR are shown in Figure 2, and the schematic diagram of the sampling inference process is shown in Figure 3
The content that needs to be rewritten is: Figure 2 shows the sampling inference steps of the DiffBFR method
The content that needs to be rewritten is: Figure 3 shows the schematic diagram of the sampling inference process of the DiffBFR method
In order not to change the original meaning, the experimental results need to be rewritten into Chinese
Compare the visualization effects of the GAN-based BFR method and the DPM-based method, as shown in Figure 4
For Figure 5, the performance of the SOTA method for BFR is compared
The performance of the BFR method The comparison of visualization effects is shown in Figure 6
In the model, we can compare the performance of IRM and TEM through visualization
In the model, the performance of IRM and TEM is compared, as shown in Figure 8
What needs to be rewritten is: Compare the IRM performance of Figure 9 under different parameters
For Figure 10, we need to compare the different Performance of parameters
The content that needs to be rewritten is: Figure 11 shows the parameter settings of each module of DiffBFR
Summary is the process of re-expressing information or ideas in a concise and clear way. It does not change the original meaning but presents the same idea by using different vocabulary and sentence structure. The purpose of a summary is to provide a clearer, more concise presentation so that readers can more easily understand and digest the information conveyed. Summarizations are useful in a variety of situations, whether in academic papers, business reports, or everyday communications, where they can be used to convey important ideas and conclusions. In short, summary is an important communication tool that can help us convey and understand information more effectively
This paper proposes a blind degraded face image restoration model DiffBFR based on the diffusion model to Solve the problems of training model collapse and long tail disappearance based on previous GAN methods. By embedding prior knowledge into the diffusion model, high-quality and clear restored images can be generated from random severely degraded face images. Specifically, this study proposes two modules, IRM and TEM, which are used to restore reality and restore details respectively. Through theoretical derivation and experimental image demonstration, the superiority of the model is proven, and qualitative and quantitative comparisons are made with existing state-of-the-art methods
The content that needs to be rewritten is: Research Team
This paper was jointly proposed by researchers from Meitu Imaging Research Institute (MT Lab) and the University of Chinese Academy of Sciences. Meitu Imaging Research Institute (MT Lab) was established in 2010. It is a team of Meitu focusing on algorithm research, engineering development and product implementation in the fields of computer vision, deep learning, augmented reality and other fields. Since its establishment, the team has been committed to exploring research in the field of computer vision, and began deploying deep learning in 2013 to provide technical support for Meitu's software and hardware products. At the same time, they also provide targeted SaaS services for multiple vertical fields in the imaging industry, and promote the ecological development of Meitu's artificial intelligence products through cutting-edge imaging technology. They have participated in top international competitions such as CVPR, ICCV, and ECCV, won more than ten championships and runner-ups, and published more than 48 top international academic conference papers. Meitu Imaging Research Institute (MT Lab) has long been committed to research and development in the imaging field, has accumulated rich technical reserves, and has rich technology implementation experience in the fields of pictures, videos, design and digital people
The above is the detailed content of ACM MM 2023 | DiffBFR: Noise suppression face restoration method jointly proposed by Meitu & Chinese University of Science and Technology. For more information, please follow other related articles on the PHP Chinese website!

Hot AI Tools

Undresser.AI Undress
AI-powered app for creating realistic nude photos

AI Clothes Remover
Online AI tool for removing clothes from photos.

Undress AI Tool
Undress images for free

Clothoff.io
AI clothes remover

Video Face Swap
Swap faces in any video effortlessly with our completely free AI face swap tool!

Hot Article

Hot Tools

Notepad++7.3.1
Easy-to-use and free code editor

SublimeText3 Chinese version
Chinese version, very easy to use

Zend Studio 13.0.1
Powerful PHP integrated development environment

Dreamweaver CS6
Visual web development tools

SublimeText3 Mac version
God-level code editing software (SublimeText3)

Hot Topics
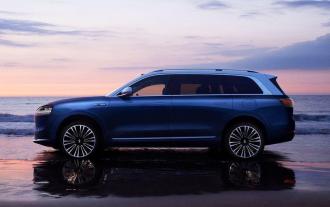
On July 29, at the roll-off ceremony of AITO Wenjie's 400,000th new car, Yu Chengdong, Huawei's Managing Director, Chairman of Terminal BG, and Chairman of Smart Car Solutions BU, attended and delivered a speech and announced that Wenjie series models will be launched this year In August, Huawei Qiankun ADS 3.0 version was launched, and it is planned to successively push upgrades from August to September. The Xiangjie S9, which will be released on August 6, will debut Huawei’s ADS3.0 intelligent driving system. With the assistance of lidar, Huawei Qiankun ADS3.0 version will greatly improve its intelligent driving capabilities, have end-to-end integrated capabilities, and adopt a new end-to-end architecture of GOD (general obstacle identification)/PDP (predictive decision-making and control) , providing the NCA function of smart driving from parking space to parking space, and upgrading CAS3.0
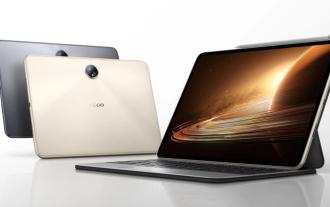
Last month, OnePlus released the first tablet equipped with Snapdragon 8 Gen3: OnePlus Tablet Pro. According to the latest news, the "baby-replacement" version of this tablet, OPPOPad3, will also be released soon. The picture above shows OPPOPad2. According to the Digital Chat Station, the appearance and configuration of OPPOPad3 are exactly the same as those of OnePlus Tablet Pro. Color: gold, blue (different from OnePlus’ green and dark gray). Storage version: 8/12/16GB+512GB. Release date: New products for the same period in the fourth quarter of this year (October-December): Find
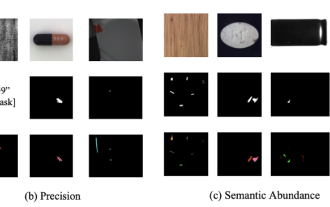
In modern manufacturing, accurate defect detection is not only the key to ensuring product quality, but also the core of improving production efficiency. However, existing defect detection datasets often lack the accuracy and semantic richness required for practical applications, resulting in models unable to identify specific defect categories or locations. In order to solve this problem, a top research team composed of Hong Kong University of Science and Technology Guangzhou and Simou Technology innovatively developed the "DefectSpectrum" data set, which provides detailed and semantically rich large-scale annotation of industrial defects. As shown in Table 1, compared with other industrial data sets, the "DefectSpectrum" data set provides the most defect annotations (5438 defect samples) and the most detailed defect classification (125 defect categories
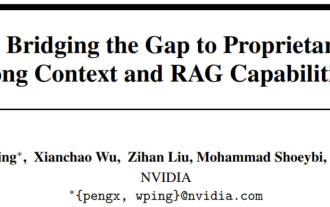
The open LLM community is an era when a hundred flowers bloom and compete. You can see Llama-3-70B-Instruct, QWen2-72B-Instruct, Nemotron-4-340B-Instruct, Mixtral-8x22BInstruct-v0.1 and many other excellent performers. Model. However, compared with proprietary large models represented by GPT-4-Turbo, open models still have significant gaps in many fields. In addition to general models, some open models that specialize in key areas have been developed, such as DeepSeek-Coder-V2 for programming and mathematics, and InternVL for visual-language tasks.
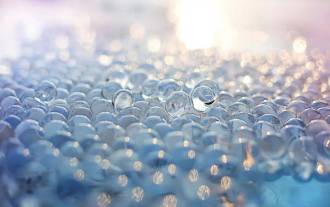
Editor |KX To this day, the structural detail and precision determined by crystallography, from simple metals to large membrane proteins, are unmatched by any other method. However, the biggest challenge, the so-called phase problem, remains retrieving phase information from experimentally determined amplitudes. Researchers at the University of Copenhagen in Denmark have developed a deep learning method called PhAI to solve crystal phase problems. A deep learning neural network trained using millions of artificial crystal structures and their corresponding synthetic diffraction data can generate accurate electron density maps. The study shows that this deep learning-based ab initio structural solution method can solve the phase problem at a resolution of only 2 Angstroms, which is equivalent to only 10% to 20% of the data available at atomic resolution, while traditional ab initio Calculation
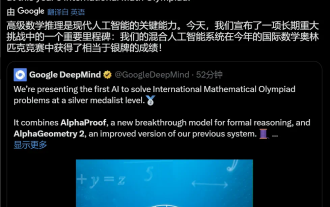
For AI, Mathematical Olympiad is no longer a problem. On Thursday, Google DeepMind's artificial intelligence completed a feat: using AI to solve the real question of this year's International Mathematical Olympiad IMO, and it was just one step away from winning the gold medal. The IMO competition that just ended last week had six questions involving algebra, combinatorics, geometry and number theory. The hybrid AI system proposed by Google got four questions right and scored 28 points, reaching the silver medal level. Earlier this month, UCLA tenured professor Terence Tao had just promoted the AI Mathematical Olympiad (AIMO Progress Award) with a million-dollar prize. Unexpectedly, the level of AI problem solving had improved to this level before July. Do the questions simultaneously on IMO. The most difficult thing to do correctly is IMO, which has the longest history, the largest scale, and the most negative
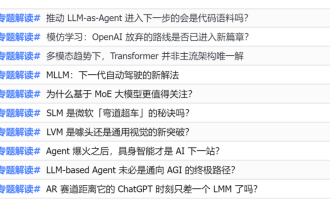
In 2023, almost every field of AI is evolving at an unprecedented speed. At the same time, AI is constantly pushing the technological boundaries of key tracks such as embodied intelligence and autonomous driving. Under the multi-modal trend, will the situation of Transformer as the mainstream architecture of AI large models be shaken? Why has exploring large models based on MoE (Mixed of Experts) architecture become a new trend in the industry? Can Large Vision Models (LVM) become a new breakthrough in general vision? ...From the 2023 PRO member newsletter of this site released in the past six months, we have selected 10 special interpretations that provide in-depth analysis of technological trends and industrial changes in the above fields to help you achieve your goals in the new year. be prepared. This interpretation comes from Week50 2023
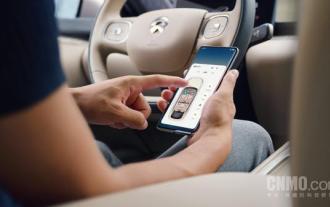
The new NIO NIO Phone (NIOPhone 2) will be released on July 27. As the release date approaches, on July 24, Bai Jian, Vice President of NIO Technology (Anhui) Co., Ltd., answered two of the most common questions about NIO Phone among netizens. NIOPhone "Why does NIO insist on making mobile phones?" Similar questions appear in almost all comment areas related to the new NIOPhone. Bai Jian responded that Weilai had started thinking and planning about making mobile phones very early, and it was not a whim, let alone as some people said, because some mobile phone brands have started to make cars. Bai Jian broke the news about the new NIOPhone "smart car and mobile phone"
