How to deal with data cleaning issues in C++ development
How to deal with data cleaning issues in C development
With the advent of the big data era, the quality of data has become a key factor in corporate decision-making and business development. In the process of big data analysis, data cleaning is a very important step, which involves removing noise from the data, filtering valid data, and repairing erroneous data. In C development, dealing with data cleaning issues is also a key task. This article will introduce how to use C to deal with data cleaning problems, and provide some practical tips and suggestions.
First of all, it is very important to understand the general process of data cleaning. Generally speaking, the data cleaning process can be divided into the following steps:
- Data collection and acquisition: Obtain raw data from various data sources, such as databases, files, API interfaces, etc.
- Data verification and screening: Verify the original data to determine whether it conforms to the expected format and specifications. Filter out the data that meets the requirements and discard the unqualified data.
- Data deduplication and denoising: Deduplicate the data and remove duplicate data. At the same time, various technical means such as interpolation, smoothing, filtering, etc. are used to remove noise in the data.
- Data repair and error correction: Repair erroneous data, such as filling in missing data values through interpolation algorithms, correcting erroneous data values through rules, etc.
- Data conversion and standardization: Format conversion of data, convert the data into a unified format and unit. Standardize data to conform to specific specifications and requirements.
The above is the general process of data cleaning. Next, we will introduce how to deal with the problems in each step in C development.
In the data collection and acquisition phase, we need to use C's input and output streams to read and write data. You can use the file stream provided by the standard library to read and write text files, use the database driver library to connect to the database to read and write data, use the network library to obtain API data, etc. What needs to be noted at this stage is that depending on the data source, you need to select appropriate libraries and technologies, and pay attention to exception handling and error handling to ensure the correct collection and acquisition of data.
In the data verification and filtering phase, we need to write code to perform data verification and filtering operations. Generally speaking, we can use regular expressions or string manipulation libraries to verify the format, length, etc. of data, and use logical operations to screen and filter data. What needs to be noted at this stage is to write robust code to handle various situations and perform error handling to ensure the accuracy and completeness of the data.
In the data deduplication and noise removal stages, we can use data structures such as hash tables or sets to remove duplicate data. For the removal of noise data, technologies such as filters and smoothing algorithms can be used. What needs to be noted at this stage is that appropriate algorithms and data structures must be selected for processing based on the characteristics of the data, and performance optimization must be performed to avoid performance bottlenecks during the processing.
In the data repair and error correction stage, we can use interpolation algorithms, correction rules and other methods to repair missing and erroneous data. What needs to be noted at this stage is to select an appropriate repair method based on the characteristics of the data, and conduct testing and verification to ensure the accuracy of the repair.
In the data conversion and standardization stage, we can use string operations and numerical conversion functions to perform data format conversion and unit conversion. What needs to be paid attention to at this stage is to ensure the accuracy of the conversion and to handle exceptions and errors.
The above are some tips and suggestions for dealing with data cleaning issues in C development. In specific projects, specific implementation and adjustments need to be made based on actual conditions. At the same time, in C development, you can also use some open source data cleaning tools and libraries, such as OpenRefine, Pandas, etc., to improve the efficiency and quality of development.
In short, data cleaning is an important task in C development. Mastering the appropriate skills and tools can efficiently handle data cleaning problems and improve data quality, thereby providing support for decision-making and business development.
The above is the detailed content of How to deal with data cleaning issues in C++ development. For more information, please follow other related articles on the PHP Chinese website!

Hot AI Tools

Undresser.AI Undress
AI-powered app for creating realistic nude photos

AI Clothes Remover
Online AI tool for removing clothes from photos.

Undress AI Tool
Undress images for free

Clothoff.io
AI clothes remover

Video Face Swap
Swap faces in any video effortlessly with our completely free AI face swap tool!

Hot Article

Hot Tools

Notepad++7.3.1
Easy-to-use and free code editor

SublimeText3 Chinese version
Chinese version, very easy to use

Zend Studio 13.0.1
Powerful PHP integrated development environment

Dreamweaver CS6
Visual web development tools

SublimeText3 Mac version
God-level code editing software (SublimeText3)

Hot Topics










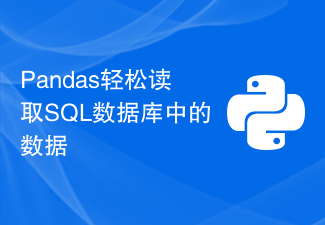
Data processing tool: Pandas reads data in SQL databases and requires specific code examples. As the amount of data continues to grow and its complexity increases, data processing has become an important part of modern society. In the data processing process, Pandas has become one of the preferred tools for many data analysts and scientists. This article will introduce how to use the Pandas library to read data from a SQL database and provide some specific code examples. Pandas is a powerful data processing and analysis tool based on Python
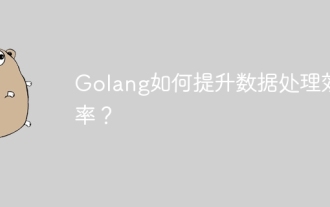
Golang improves data processing efficiency through concurrency, efficient memory management, native data structures and rich third-party libraries. Specific advantages include: Parallel processing: Coroutines support the execution of multiple tasks at the same time. Efficient memory management: The garbage collection mechanism automatically manages memory. Efficient data structures: Data structures such as slices, maps, and channels quickly access and process data. Third-party libraries: covering various data processing libraries such as fasthttp and x/text.
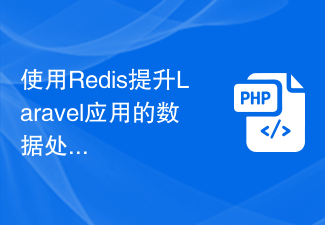
Use Redis to improve the data processing efficiency of Laravel applications. With the continuous development of Internet applications, data processing efficiency has become one of the focuses of developers. When developing applications based on the Laravel framework, we can use Redis to improve data processing efficiency and achieve fast access and caching of data. This article will introduce how to use Redis for data processing in Laravel applications and provide specific code examples. 1. Introduction to Redis Redis is a high-performance memory data
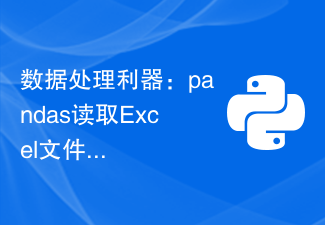
With the increasing popularity of data processing, more and more people are paying attention to how to use data efficiently and make the data work for themselves. In daily data processing, Excel tables are undoubtedly the most common data format. However, when a large amount of data needs to be processed, manually operating Excel will obviously become very time-consuming and laborious. Therefore, this article will introduce an efficient data processing tool - pandas, and how to use this tool to quickly read Excel files and perform data processing. 1. Introduction to pandas pandas
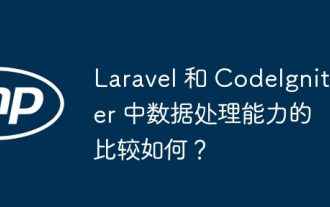
Compare the data processing capabilities of Laravel and CodeIgniter: ORM: Laravel uses EloquentORM, which provides class-object relational mapping, while CodeIgniter uses ActiveRecord to represent the database model as a subclass of PHP classes. Query builder: Laravel has a flexible chained query API, while CodeIgniter’s query builder is simpler and array-based. Data validation: Laravel provides a Validator class that supports custom validation rules, while CodeIgniter has less built-in validation functions and requires manual coding of custom rules. Practical case: User registration example shows Lar
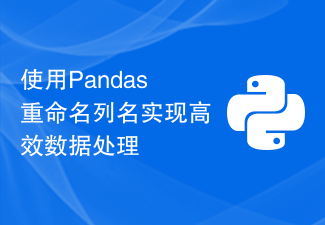
Efficient data processing: Using Pandas to modify column names requires specific code examples. Data processing is a very important part of data analysis, and during the data processing process, it is often necessary to modify the column names of the data. Pandas is a powerful data processing library that provides a wealth of methods and functions to help us process data quickly and efficiently. This article will introduce how to use Pandas to modify column names and provide specific code examples. In actual data analysis, the column names of the original data may have inconsistent naming standards and are difficult to understand.
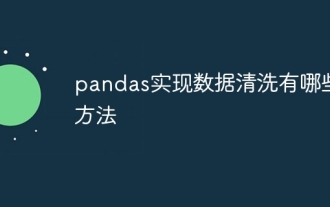
The methods used by pandas to implement data cleaning include: 1. Missing value processing; 2. Duplicate value processing; 3. Data type conversion; 4. Outlier processing; 5. Data normalization; 6. Data filtering; 7. Data aggregation and grouping; 8 , Pivot table, etc. Detailed introduction: 1. Missing value processing, Pandas provides a variety of methods for processing missing values. For missing values, you can use the "fillna()" method to fill in specific values, such as mean, median, etc.; 2. Repeat Value processing, in data cleaning, removing duplicate values is a very common step and so on.
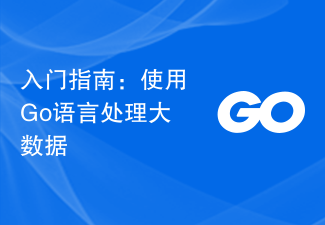
As an open source programming language, Go language has gradually received widespread attention and use in recent years. It is favored by programmers for its simplicity, efficiency, and powerful concurrent processing capabilities. In the field of big data processing, the Go language also has strong potential. It can be used to process massive data, optimize performance, and can be well integrated with various big data processing tools and frameworks. In this article, we will introduce some basic concepts and techniques of big data processing in Go language, and show how to use Go language through specific code examples.
