


The Open LLM list has been refreshed again, and a 'Platypus' stronger than Llama 2 is here.
In order to challenge the dominance of closed models such as OpenAI’s GPT-3.5 and GPT-4, a series of open source models are emerging, including LLaMa, Falcon, etc. Recently, Meta AI launched LLaMa-2, which is known as the most powerful model in the open source field, and many researchers have also built their own models on this basis. For example, StabilityAI used Orca-style data sets to fine-tune the Llama2 70B model and developed StableBeluga2, which also achieved good results on Huggingface's Open LLM rankings
The latest Open The LLM list ranking has changed, and the Platypus (Platypus) model has successfully climbed to the top of the list
The author is from Boston University and uses PEFT and LoRA And the dataset Open-Platypus fine-tuned and optimized Platypus based on Llama 2
The author introduced Platypus in detail in a paper
The paper can be found at: https://arxiv.org/abs/2308.07317
The following are the main contributions of this article:
- Open-Platypus is a small-scale dataset consisting of a curated subset of public text datasets . This dataset consists of 11 open source datasets with a focus on improving LLM’s STEM and logic knowledge. It consists mainly of questions designed by humans, with only 10% of questions generated by LLM. The main advantage of Open-Platypus is its scale and quality, which enables very high performance in a short time and with low time and cost of fine-tuning. Specifically, training a 13B model using 25k problems takes just 5 hours on a single A100 GPU.
- Describes the similarity elimination process, reduces the size of the dataset, and reduces data redundancy.
- The ever-present phenomenon of contamination of open LLM training sets with data contained in important LLM test sets is analyzed in detail, and the author's training data filtering process to avoid this hidden danger is introduced.
- Describes the process of selecting and merging specialized fine-tuned LoRA modules.
Open-Platypus Dataset
The author has currently released the Open-Platypus Dataset on Hugging Face
Contamination problem
To avoid benchmarking problems leaking into the training set, This approach first considers preventing this problem to ensure that the results are not simply biased by memory. While striving for accuracy, the authors are also aware of the need for flexibility in marking please say again questions because questions can be asked in a variety of ways and are influenced by general domain knowledge. To manage potential leakage issues, the authors carefully designed heuristics for manually filtering problems with more than 80% similarity to the cosine embedding of the benchmark problem in Open-Platypus. They divided potential leak issues into three categories: (1) Please say the question again; (2) Rephrase: This area presents a gray toned problem; (3) similar but not identical problem. To be cautious, they excluded all of these problems from the training set
Please say it again
This text almost exactly replicates the content of the test question set, with only slight modifications or rearrangements of the words. Based on the number of leaks in the table above, the authors believe this is the only category that falls under contamination. The following are specific examples:
Redescription: This area has a gray tint
The following issues are called redescriptions: This area takes on a shade of gray and includes issues that are not exactly, please, common sense. While the authors leave the final judgment on these issues to the open source community, they argue that these issues often require expert knowledge. It should be noted that this type of questions includes questions with exactly the same instructions but synonymous answers:
Similar but not identical
These questions have a high degree of similarity, but due to subtle changes between the questions, there are significant differences in the answers.
Fine-tuning and merging
After the data set is improved, the author focuses on two methods: low Rank approximation (LoRA) training and parameter efficient fine-tuning (PEFT) library. Unlike full fine-tuning, LoRA retains the weights of the pre-trained model and uses the rank decomposition matrix for integration in the transformer layer, thereby reducing trainable parameters and saving training time and cost. Initially, fine-tuning mainly focused on attention modules such as v_proj, q_proj, k_proj and o_proj. Subsequently, it was extended to the gate_proj, down_proj and up_proj modules according to the suggestions of He et al. Unless the trainable parameters are less than 0.1% of the total parameters, these modules all show better results. The author adopted this method for both the 13B and 70B models, and the result was that the trainable parameters were 0.27% and 0.2% respectively. The only difference is the initial learning rate of these models
The results
According to the Hugging Face Open LLM ranking data on August 10, 2023, The author compared Platypus with other SOTA models and found that the Platypus2-70Binstruct variant performed well, ranking first with an average score of 73.13
Stable -Platypus2-13B model stands out with an average score of 63.96 among 13 billion parameter models, which deserves attention
##Limitations
Platypus, as a fine-tuned extension of LLaMa-2, retains many of the constraints of the base model and introduces specific challenges through targeted training. It shares the static knowledge base of LLaMa-2, which may become outdated . Additionally, there is a risk of generating inaccurate or inappropriate content, particularly in cases of unclear prompts. While Platypus has been enhanced in STEM and English logic, its proficiency in other languages is not reliable and may be inconsistent. It occasionally produces biased or inconsistent harmful content. The author acknowledges efforts to minimize these issues but acknowledges the ongoing challenges, particularly in non-English languages.
The potential for abuse of Platypus is a concern. issues, so developers should conduct security testing of their applications before deployment. Platypus may have some limitations outside of its primary domain, so users should proceed with caution and consider additional fine-tuning for optimal performance. Users need to ensure that the training data for Platypus does not overlap with other benchmark test sets. The authors are very cautious about data contamination issues and avoid merging models with models trained on tainted datasets. Although it is confirmed that there is no contamination in the cleaned training data, it cannot be ruled out that some problems may have been overlooked. For details on these limitations, see the Limitations section in the paper
The above is the detailed content of The Open LLM list has been refreshed again, and a 'Platypus' stronger than Llama 2 is here.. For more information, please follow other related articles on the PHP Chinese website!

Hot AI Tools

Undresser.AI Undress
AI-powered app for creating realistic nude photos

AI Clothes Remover
Online AI tool for removing clothes from photos.

Undress AI Tool
Undress images for free

Clothoff.io
AI clothes remover

Video Face Swap
Swap faces in any video effortlessly with our completely free AI face swap tool!

Hot Article

Hot Tools

Notepad++7.3.1
Easy-to-use and free code editor

SublimeText3 Chinese version
Chinese version, very easy to use

Zend Studio 13.0.1
Powerful PHP integrated development environment

Dreamweaver CS6
Visual web development tools

SublimeText3 Mac version
God-level code editing software (SublimeText3)

Hot Topics










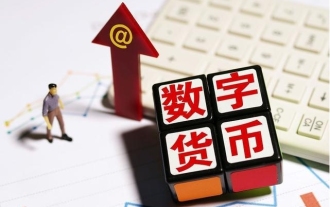
The top 10 digital virtual currency trading platforms are: 1. Binance, 2. OKX, 3. Coinbase, 4. Kraken, 5. Huobi Global, 6. Bitfinex, 7. KuCoin, 8. Gemini, 9. Bitstamp, 10. Bittrex. These platforms all provide high security and a variety of trading options, suitable for different user needs.
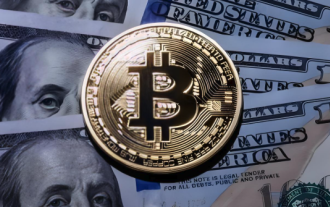
The built-in quantization tools on the exchange include: 1. Binance: Provides Binance Futures quantitative module, low handling fees, and supports AI-assisted transactions. 2. OKX (Ouyi): Supports multi-account management and intelligent order routing, and provides institutional-level risk control. The independent quantitative strategy platforms include: 3. 3Commas: drag-and-drop strategy generator, suitable for multi-platform hedging arbitrage. 4. Quadency: Professional-level algorithm strategy library, supporting customized risk thresholds. 5. Pionex: Built-in 16 preset strategy, low transaction fee. Vertical domain tools include: 6. Cryptohopper: cloud-based quantitative platform, supporting 150 technical indicators. 7. Bitsgap:
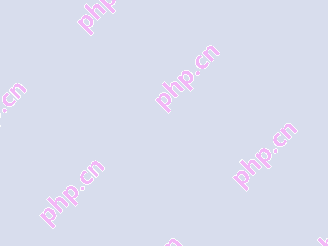
This groundbreaking development will enable financial institutions to leverage the globally recognized ISO20022 standard to automate banking processes across different blockchain ecosystems. The Ease protocol is an enterprise-level blockchain platform designed to promote widespread adoption through easy-to-use methods. It announced today that it has successfully integrated the ISO20022 messaging standard and directly incorporated it into blockchain smart contracts. This development will enable financial institutions to easily automate banking processes in different blockchain ecosystems using the globally recognized ISO20022 standard, which is replacing the Swift messaging system. These features will be tried soon on "EaseTestnet". EaseProtocolArchitectDou
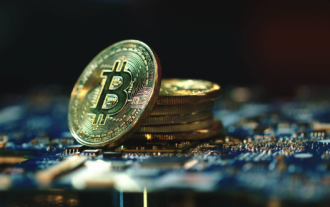
The prospects of digital currency apps are broad, which are specifically reflected in: 1. Technology innovation-driven function upgrades, improving user experience through the integration of DeFi and NFT and AI and big data applications; 2. Regulatory compliance trends, global framework improvements and stricter requirements for AML and KYC; 3. Function diversification and service expansion, integrating lending, financial management and other services and optimizing user experience; 4. User base and global expansion, and the user scale is expected to exceed 1 billion in 2025.
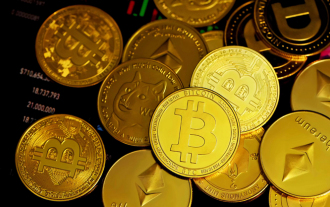
In its latest attempt, the resolved crypto exchange FTX has taken legal action to recover debts and pay back customers. In the latest efforts to recover debts and repay clients, the resolved crypto exchange FTX has filed legal action against specific issuers. FTX Trading and FTX Recovery Trust have filed lawsuits against certain token issuers who failed to fulfill their agreement to remit agreed coins to the exchange. Specifically, the restructuring team sued NFTStars Limited and Orosemi Inc. on Monday over compliance issues. FTX is suing the token issuer to recover the expired coins. FTX was once one of the most outstanding cryptocurrency trading platforms in the United States. The bank reported in November 2022 that its founder Sam
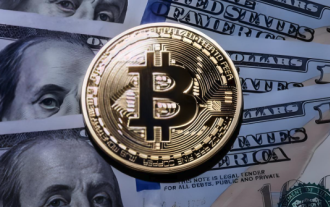
In the currency circle, the so-called Big Three usually refers to the three most influential and widely used cryptocurrencies. These cryptocurrencies have a significant role in the market and have performed well in terms of transaction volume and market capitalization. At the same time, the mainstream virtual currency exchange APP is also an important tool for investors and traders to conduct cryptocurrency trading. This article will introduce in detail the three giants in the currency circle and the top ten mainstream virtual currency exchange APPs recommended.
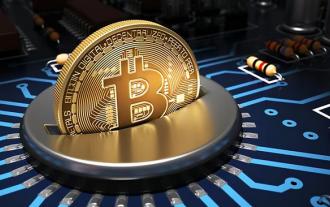
The top ten digital currency exchanges are: 1. Binance, 2. OKX, 3. Coinbase, 4. Kraken, 5. Huobi Global, 6. Bitfinex, 7. KuCoin, 8. Gemini, 9. Bitstamp, 10. Bittrex. These platforms all offer high security and a variety of trading options, suitable for different user needs.

In Composer, AI mainly improves development efficiency and code quality through dependency recommendation, dependency conflict resolution and code quality improvement. 1. AI can recommend appropriate dependency packages according to project needs. 2. AI provides intelligent solutions to deal with dependency conflicts. 3. AI reviews code and provides optimization suggestions to improve code quality. Through these functions, developers can focus more on the implementation of business logic.
