


Based on information theory calibration technology, CML makes multi-modal machine learning more reliable
Multimodal machine learning has made impressive progress in various scenarios. However, the reliability of multimodal learning models lacks in-depth research. "Information is the elimination of uncertainty." The original intention of multi-modal machine learning is consistent with this - added modalities can make predictions more accurate and reliable. However, the paper "Calibrating Multimodal Learning" recently published in ICML2023 found that current multimodal learning methods violate this reliability assumption, and made detailed analysis and corrections.
Picture
- ##Paper Arxiv: https:// arxiv.org/abs/2306.01265
- Code GitHub: https://github.com/QingyangZhang/CML
The current multi-modal classification method has unreliable confidence, that is, when some modes are removed, the model may produce higher confidence, which violates the information theory "Information is the elimination of uncertainty" is the basic principle. To address this problem, this article proposes the Calibrating Multimodal Learning method. This method can be deployed in different multi-modal learning paradigms to improve the rationality and credibility of multi-modal learning models.
Picture
This work points out that current multi-modal learning methods have unreliable prediction confidence problems. Modal machine learning models tend to rely on partial modalities to estimate confidence. In particular, the study found that the confidence of current model estimates increases when certain modes are damaged. To solve this unreasonable problem, the authors propose an intuitive multi-modal learning principle: when the modality is removed, the model prediction confidence should not increase. However, current models tend to believe and be influenced by a subset of modalities, rather than considering all modalities fairly. This further affects the robustness of the model, i.e. the model is easily affected when certain modes are damaged.
To solve the above problems, some current methods adopt existing uncertainty calibration methods, such as Temperature Scaling or Bayesian learning methods. These methods can construct more accurate confidence estimates than traditional training/inference methods. However, these methods only match the confidence estimate of the final fusion result with the accuracy, and do not explicitly consider the relationship between the modal information amount and confidence. Therefore, they cannot essentially improve the credibility of the multi-modal learning model.
The author proposes a new regularization technique called "Calibrating Multimodal Learning (CML)". This technique enforces the matching relationship between model prediction confidence and information content by adding a penalty term to achieve consistency between prediction confidence and information content. This technique is based on the natural intuition that when a modality is removed, prediction confidence should decrease (at least it should not increase), which can inherently improve confidence calibration. Specifically, a simple regularization term is proposed to force the model to learn intuitive ordering relationships by adding a penalty to those samples whose prediction confidence increases when a modality is removed:
The above constraints are regular losses, which appear as penalties when modal information is removed and confidence increases.
Experimental results show that CML regularization can significantly improve the reliability of prediction confidence of existing multi-modal learning methods. Additionally, CML can improve classification accuracy and improve model robustness.
Multimodal machine learning has made significant progress in various scenarios, but the reliability of multimodal machine learning models is still a problem that needs to be solved. Through extensive empirical research, this paper finds that current multimodal classification methods have the problem of unreliable prediction confidence and violate the principles of information theory. To address this issue, researchers proposed the CML regularization technique, which can be flexibly deployed to existing models and improve performance in terms of confidence calibration, classification accuracy, and model robustness. It is believed that this new technology will play an important role in future multi-modal learning and improve the reliability and practicality of machine learning.
The above is the detailed content of Based on information theory calibration technology, CML makes multi-modal machine learning more reliable. For more information, please follow other related articles on the PHP Chinese website!

Hot AI Tools

Undresser.AI Undress
AI-powered app for creating realistic nude photos

AI Clothes Remover
Online AI tool for removing clothes from photos.

Undress AI Tool
Undress images for free

Clothoff.io
AI clothes remover

Video Face Swap
Swap faces in any video effortlessly with our completely free AI face swap tool!

Hot Article

Hot Tools

Notepad++7.3.1
Easy-to-use and free code editor

SublimeText3 Chinese version
Chinese version, very easy to use

Zend Studio 13.0.1
Powerful PHP integrated development environment

Dreamweaver CS6
Visual web development tools

SublimeText3 Mac version
God-level code editing software (SublimeText3)

Hot Topics










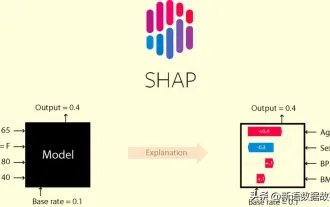
In the fields of machine learning and data science, model interpretability has always been a focus of researchers and practitioners. With the widespread application of complex models such as deep learning and ensemble methods, understanding the model's decision-making process has become particularly important. Explainable AI|XAI helps build trust and confidence in machine learning models by increasing the transparency of the model. Improving model transparency can be achieved through methods such as the widespread use of multiple complex models, as well as the decision-making processes used to explain the models. These methods include feature importance analysis, model prediction interval estimation, local interpretability algorithms, etc. Feature importance analysis can explain the decision-making process of a model by evaluating the degree of influence of the model on the input features. Model prediction interval estimate
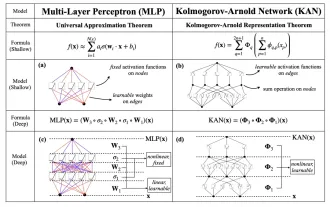
Earlier this month, researchers from MIT and other institutions proposed a very promising alternative to MLP - KAN. KAN outperforms MLP in terms of accuracy and interpretability. And it can outperform MLP running with a larger number of parameters with a very small number of parameters. For example, the authors stated that they used KAN to reproduce DeepMind's results with a smaller network and a higher degree of automation. Specifically, DeepMind's MLP has about 300,000 parameters, while KAN only has about 200 parameters. KAN has a strong mathematical foundation like MLP. MLP is based on the universal approximation theorem, while KAN is based on the Kolmogorov-Arnold representation theorem. As shown in the figure below, KAN has
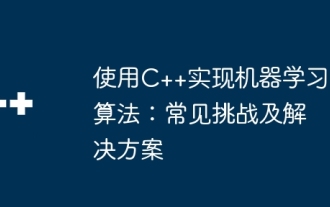
Common challenges faced by machine learning algorithms in C++ include memory management, multi-threading, performance optimization, and maintainability. Solutions include using smart pointers, modern threading libraries, SIMD instructions and third-party libraries, as well as following coding style guidelines and using automation tools. Practical cases show how to use the Eigen library to implement linear regression algorithms, effectively manage memory and use high-performance matrix operations.
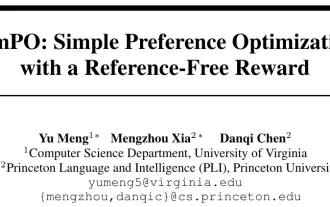
In order to align large language models (LLMs) with human values and intentions, it is critical to learn human feedback to ensure that they are useful, honest, and harmless. In terms of aligning LLM, an effective method is reinforcement learning based on human feedback (RLHF). Although the results of the RLHF method are excellent, there are some optimization challenges involved. This involves training a reward model and then optimizing a policy model to maximize that reward. Recently, some researchers have explored simpler offline algorithms, one of which is direct preference optimization (DPO). DPO learns the policy model directly based on preference data by parameterizing the reward function in RLHF, thus eliminating the need for an explicit reward model. This method is simple and stable
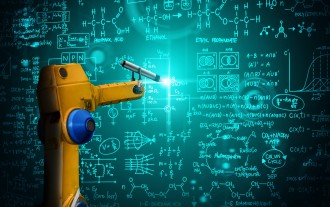
Machine learning is an important branch of artificial intelligence that gives computers the ability to learn from data and improve their capabilities without being explicitly programmed. Machine learning has a wide range of applications in various fields, from image recognition and natural language processing to recommendation systems and fraud detection, and it is changing the way we live. There are many different methods and theories in the field of machine learning, among which the five most influential methods are called the "Five Schools of Machine Learning". The five major schools are the symbolic school, the connectionist school, the evolutionary school, the Bayesian school and the analogy school. 1. Symbolism, also known as symbolism, emphasizes the use of symbols for logical reasoning and expression of knowledge. This school of thought believes that learning is a process of reverse deduction, through existing
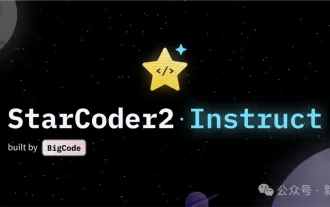
At the forefront of software technology, UIUC Zhang Lingming's group, together with researchers from the BigCode organization, recently announced the StarCoder2-15B-Instruct large code model. This innovative achievement achieved a significant breakthrough in code generation tasks, successfully surpassing CodeLlama-70B-Instruct and reaching the top of the code generation performance list. The unique feature of StarCoder2-15B-Instruct is its pure self-alignment strategy. The entire training process is open, transparent, and completely autonomous and controllable. The model generates thousands of instructions via StarCoder2-15B in response to fine-tuning the StarCoder-15B base model without relying on expensive manual annotation.
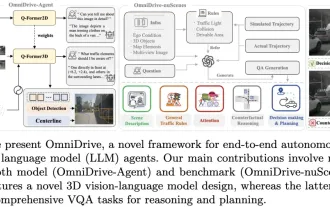
Written above & the author’s personal understanding: This paper is dedicated to solving the key challenges of current multi-modal large language models (MLLMs) in autonomous driving applications, that is, the problem of extending MLLMs from 2D understanding to 3D space. This expansion is particularly important as autonomous vehicles (AVs) need to make accurate decisions about 3D environments. 3D spatial understanding is critical for AVs because it directly impacts the vehicle’s ability to make informed decisions, predict future states, and interact safely with the environment. Current multi-modal large language models (such as LLaVA-1.5) can often only handle lower resolution image inputs (e.g.) due to resolution limitations of the visual encoder, limitations of LLM sequence length. However, autonomous driving applications require
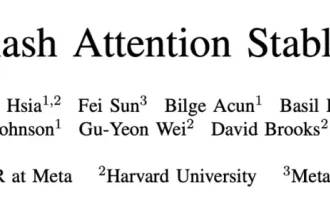
MetaFAIR teamed up with Harvard to provide a new research framework for optimizing the data bias generated when large-scale machine learning is performed. It is known that the training of large language models often takes months and uses hundreds or even thousands of GPUs. Taking the LLaMA270B model as an example, its training requires a total of 1,720,320 GPU hours. Training large models presents unique systemic challenges due to the scale and complexity of these workloads. Recently, many institutions have reported instability in the training process when training SOTA generative AI models. They usually appear in the form of loss spikes. For example, Google's PaLM model experienced up to 20 loss spikes during the training process. Numerical bias is the root cause of this training inaccuracy,
