


Scrapy practice: how to crawl Twitter data and analyze user sentiment
With the increasing popularity of social media, a large number of users have generated massive amounts of data, and these data contain huge commercial value. In order to make better use of this data, we need a tool that can automatically obtain the data and analyze it. Scrapy is such a powerful crawler framework. Scrapy can help us quickly obtain large amounts of data and perform various forms of statistical analysis.
In this article, I will introduce to you how to use the Scrapy framework to crawl Twitter data and analyze user sentiment through analysis.
Step One: Install Scrapy
First, you need to make sure that the Python environment has been installed on your computer, and then enter the following statement on the command line to install Scrapy:
pip install scrapy
This process may take some time because the Scrapy installation package is relatively large.
Step 2: Create a Scrapy project
After installing Scrapy, we need to create a new Scrapy project. Assume that our project is named "twitter", enter in the command line:
scrapy startproject twitter
After execution, a folder named "twitter" will be created in the current directory, which contains the information required by the Scrapy framework. of various files and folders.
Step Three: Write the crawler code
After completing the creation of the Scrapy project, we need to write the crawler code. In Scrapy, the crawler code is written in a .py file in the spiders directory. We need to create a new .py file first. Assume that our file is named "twitter_spider.py" and enter:
scrapy genspider twitter_spider twitter.com
After executing the above command, a file named "twitter_spider.py" will be created in the spiders directory, with "twitter.com" as the initial URL by default.
Next, we need to write code in "twitter_spider.py" to crawl the Twitter website data. The following is a simple example:
import scrapy class TwitterSpider(scrapy.Spider): name = "twitter_spider" allowed_domains = ["twitter.com"] start_urls = ["https://twitter.com/search?q=feminist&src=typed_query"] def parse(self, response): filename = response.url.split("/")[-2] + ".html" with open(filename, 'wb') as f: f.write(response.body) self.log('保存文件: %s' % filename)
In the code, we specify the name of the crawler as "twitter_spider", the domain name allowed to be accessed as "twitter.com", and the initial URL is set to "https:// twitter.com/search?q=feminist&src=typed_query". When the crawler accesses this URL, it will call the parse method to parse the web page content. In the example, we save the crawled web page locally and output the saved file name.
Step 4: Run the Scrapy crawler
After writing the crawler code, we need to run the Scrapy framework to perform the crawler task. Enter in the command line:
scrapy crawl twitter_spider
After executing the command, the crawler will start running. After the operation is completed, the crawled data will be saved locally.
Step 5: Analyze user sentiment
Now, we have successfully used the Scrapy framework to crawl Twitter data. Next, we need to analyze the data and analyze the emotional tendencies of Twitter users.
In analyzing user sentiment, we can use some third-party sentiment analysis libraries to parse the text and determine the intensity of the sentiment. For example, the TextBlob sentiment analysis library in Python can help us determine the sentiment contained in the text and output a sentiment score.
The code for using TextBlob is as follows:
from textblob import TextBlob blob = TextBlob("I love this place.") print(blob.sentiment)
In the output result, the emotion score is between -1 and 1. If the score is -1, it means completely negative emotion; the score is 0, Indicates emotional neutrality; a score of 1 indicates completely positive emotion.
Now, we can apply this sentiment analysis function to the Twitter data set we crawled, get the sentiment score expressed by each user, and further analyze whether the user's emotional tendency is positive or negative.
To sum up, Scrapy is a flexible and powerful crawler framework that can help us quickly obtain massive data and make better analysis. By analyzing Twitter user sentiment, we can better understand users' preferences and attitudes, and then develop more effective promotion strategies.
The above is the detailed content of Scrapy practice: how to crawl Twitter data and analyze user sentiment. For more information, please follow other related articles on the PHP Chinese website!

Hot AI Tools

Undresser.AI Undress
AI-powered app for creating realistic nude photos

AI Clothes Remover
Online AI tool for removing clothes from photos.

Undress AI Tool
Undress images for free

Clothoff.io
AI clothes remover

Video Face Swap
Swap faces in any video effortlessly with our completely free AI face swap tool!

Hot Article

Hot Tools

Notepad++7.3.1
Easy-to-use and free code editor

SublimeText3 Chinese version
Chinese version, very easy to use

Zend Studio 13.0.1
Powerful PHP integrated development environment

Dreamweaver CS6
Visual web development tools

SublimeText3 Mac version
God-level code editing software (SublimeText3)

Hot Topics










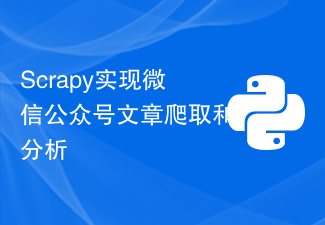
Scrapy implements article crawling and analysis of WeChat public accounts. WeChat is a popular social media application in recent years, and the public accounts operated in it also play a very important role. As we all know, WeChat public accounts are an ocean of information and knowledge, because each public account can publish articles, graphic messages and other information. This information can be widely used in many fields, such as media reports, academic research, etc. So, this article will introduce how to use the Scrapy framework to crawl and analyze WeChat public account articles. Scr
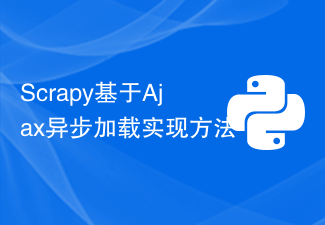
Scrapy is an open source Python crawler framework that can quickly and efficiently obtain data from websites. However, many websites use Ajax asynchronous loading technology, making it impossible for Scrapy to obtain data directly. This article will introduce the Scrapy implementation method based on Ajax asynchronous loading. 1. Ajax asynchronous loading principle Ajax asynchronous loading: In the traditional page loading method, after the browser sends a request to the server, it must wait for the server to return a response and load the entire page before proceeding to the next step.
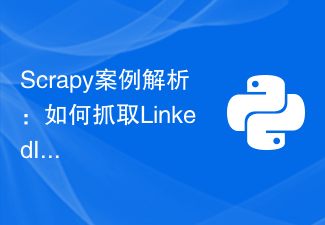
Scrapy is a Python-based crawler framework that can quickly and easily obtain relevant information on the Internet. In this article, we will use a Scrapy case to analyze in detail how to crawl company information on LinkedIn. Determine the target URL First, we need to make it clear that our target is the company information on LinkedIn. Therefore, we need to find the URL of the LinkedIn company information page. Open the LinkedIn website, enter the company name in the search box, and
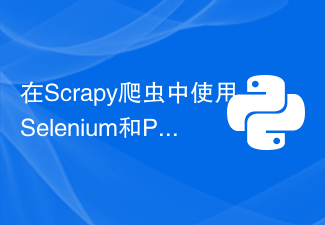
Using Selenium and PhantomJS in Scrapy crawlers Scrapy is an excellent web crawler framework under Python and has been widely used in data collection and processing in various fields. In the implementation of the crawler, sometimes it is necessary to simulate browser operations to obtain the content presented by certain websites. In this case, Selenium and PhantomJS are needed. Selenium simulates human operations on the browser, allowing us to automate web application testing
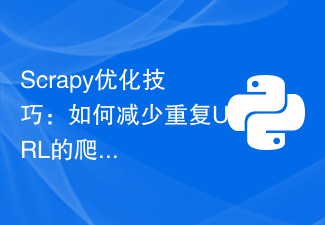
Scrapy is a powerful Python crawler framework that can be used to obtain large amounts of data from the Internet. However, when developing Scrapy, we often encounter the problem of crawling duplicate URLs, which wastes a lot of time and resources and affects efficiency. This article will introduce some Scrapy optimization techniques to reduce the crawling of duplicate URLs and improve the efficiency of Scrapy crawlers. 1. Use the start_urls and allowed_domains attributes in the Scrapy crawler to
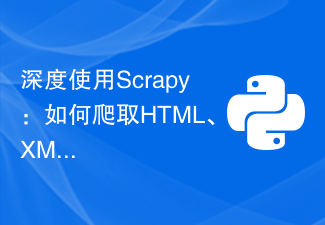
Scrapy is a powerful Python crawler framework that can help us obtain data on the Internet quickly and flexibly. In the actual crawling process, we often encounter various data formats such as HTML, XML, and JSON. In this article, we will introduce how to use Scrapy to crawl these three data formats respectively. 1. Crawl HTML data and create a Scrapy project. First, we need to create a Scrapy project. Open the command line and enter the following command: scrapys
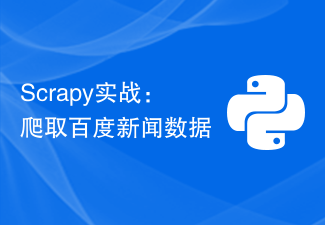
Scrapy in action: Crawling Baidu news data With the development of the Internet, the main way people obtain information has shifted from traditional media to the Internet, and people increasingly rely on the Internet to obtain news information. For researchers or analysts, a large amount of data is needed for analysis and research. Therefore, this article will introduce how to use Scrapy to crawl Baidu news data. Scrapy is an open source Python crawler framework that can crawl website data quickly and efficiently. Scrapy provides powerful web page parsing and crawling functions
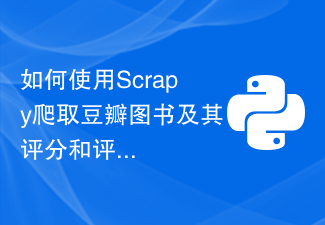
With the development of the Internet, people increasingly rely on the Internet to obtain information. For book lovers, Douban Books has become an indispensable platform. In addition, Douban Books also provides a wealth of book ratings and reviews, allowing readers to understand a book more comprehensively. However, manually obtaining this information is tantamount to finding a needle in a haystack. At this time, we can use the Scrapy tool to crawl data. Scrapy is an open source web crawler framework based on Python, which can help us efficiently
