


Tian Yuandong's new work: Opening the first layer of Transformer black box, the attention mechanism is not so mysterious
The Transformer architecture has swept across many fields including natural language processing, computer vision, speech, multi-modality, etc. However, the experimental results are currently very impressive, and the relevant research on the working principle of Transformer is still very limited.
The biggest mystery is why Transformer can emerge efficient representations from gradient training dynamics by relying only on a "simple prediction loss"?
Recently Dr. Tian Yuandong announced the team’s latest research results. In a mathematically rigorous way, he analyzed the performance of a layer of Transformer (a self-attention layer plus a decoder layer) in the next token prediction task. SGD training dynamics on.
## Paper link: https://arxiv.org/abs/2305.16380
This paper opens the black box of the dynamic process of how self-attention layers combine input tokens, and reveals the nature of potential inductive bias.
Specifically, under the assumption that there is no position encoding, long input sequences, and the decoder layer learns faster than the self-attention layer, the researchers proved that self-attention is a Discriminative scanning algorithm :
Starting from uniform attention (uniform attention), for the specific next token to be predicted, the model Gradually pay attention to different key tokens, and pay less attention to common tokens that appear in multiple next token windows
For different tokens, the model will gradually reduce the attention weight, following the training The order of co-occurrence between concentrated key tokens and query tokens from low to high.
What’s interesting is that this process does not lead to a winner-take-all, but is slowed down by a phase transition controlled by the two-layer learning rate, and finally becomes an (almost) fixed token combination. This dynamic is also verified on synthetic and real-world data.
Dr. Tian Yuandong is a researcher and research manager at the Meta Artificial Intelligence Research Institute and the leader of the Go AI project. His research directions are deep reinforcement learning and its application in games, as well as deep learning models theoretical analysis. He received his bachelor's and master's degrees from Shanghai Jiao Tong University in 2005 and 2008, and his doctorate from the Robotics Institute of Carnegie Mellon University in the United States in 2013.
was nominated for the 2013 International Conference on Computer Vision (ICCV) Marr Prize Honorable Mentions and the ICML2021 Outstanding Paper Honorable Mention Award.
After graduating from the Ph.D., he published a series of "Five-Year Doctoral Summary", covering aspects such as research direction selection, reading accumulation, time management, work attitude, income and sustainable career development. Summary of thoughts and experiences on doctoral career.
Revealing the 1-layer TransformerThe pre-training model based on the Transformer architecture usually only includes very simple supervision tasks, such as predicting the next word, filling in the blanks, etc., but it can Providing very rich representations for downstream tasks is mind-boggling.
Although previous work has proven that Transformer is essentially a universal approximator, previously commonly used machine learning models, such as kNN, kernel SVM, and multi-layer perceptron etc. are actually universal approximators. This theory cannot explain the huge gap in performance between these two types of models.
Researchers believe that it is important to understand the training dynamics of Transformer, that is, in During training, you can learn how parameters change over time.
The article first uses a rigorous mathematical definition to formally describe the training dynamics of SGD with a layer of positionless coding Transformer on the next token prediction (a commonly used training paradigm for GPT series models). .
The Transformer of layer 1 contains a softmax self-attention layer and a decoder layer that predicts the next token.
Assuming that the sequence is long and the decoder learns faster than the self-attention layer, prove The dynamic behavior of self-attention during training:
1. Frequency Bias
The model will gradually pay attention to those key tokens that co-occur with the query token in large quantities, and reduce its attention to those tokens that co-occur less.
2. Discriminative Bias
The model pays more attention to those to be predicted next The only unique token that appears in the next token, and loses interest in those common tokens that appear in multiple next tokens.
These two characteristics show that self-attention implicitly runs a discriminative scanning algorithm and has an inductive bias, that is, it is biased towards Unique key tokens that often co-occur with query tokens
Additionally, although self-attention layers tend to become sparser during training, as the frequency bias suggests, the model Because of the phase transition in the training dynamics, it does not collapse into one hot.
The final stage of learning does not converge to any saddle point with zero gradient, but instead enters an attention change Slow region (i.e. logarithm over time), with parameter freezing and learned.
The research results further show that the onset of phase transition is controlled by the learning rate: a large learning rate will produce sparse attention patterns, while under a fixed self-attention learning rate , a large decoder learning rate leads to faster phase transitions and dense attention patterns.
The researchers named the SGD dynamics discovered in their work scan and snap:
Scan phase: Self attention is focused on key tokens, that is, different tokens that often appear at the same time as the next predicted token; attention on all other tokens decreases.
snap stage: Attention is almost frozen, and the token combination is fixed.
This phenomenon has also been verified in simple real-world data experiments, using SGD trained on WikiText 1 Observing the lowest self-attention layer of the layer and the 3-layer Transformer, we can find that even if the learning rate remains constant throughout the training process, the attention will freeze at a certain moment during the training process and become sparse.
The above is the detailed content of Tian Yuandong's new work: Opening the first layer of Transformer black box, the attention mechanism is not so mysterious. For more information, please follow other related articles on the PHP Chinese website!

Hot AI Tools

Undresser.AI Undress
AI-powered app for creating realistic nude photos

AI Clothes Remover
Online AI tool for removing clothes from photos.

Undress AI Tool
Undress images for free

Clothoff.io
AI clothes remover

Video Face Swap
Swap faces in any video effortlessly with our completely free AI face swap tool!

Hot Article

Hot Tools

Notepad++7.3.1
Easy-to-use and free code editor

SublimeText3 Chinese version
Chinese version, very easy to use

Zend Studio 13.0.1
Powerful PHP integrated development environment

Dreamweaver CS6
Visual web development tools

SublimeText3 Mac version
God-level code editing software (SublimeText3)

Hot Topics










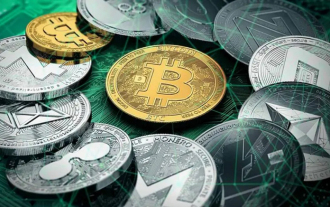
The top ten cryptocurrency exchanges in the world in 2025 include Binance, OKX, Gate.io, Coinbase, Kraken, Huobi, Bitfinex, KuCoin, Bittrex and Poloniex, all of which are known for their high trading volume and security.
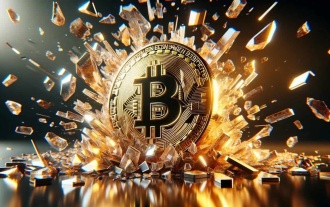
Bitcoin’s price ranges from $20,000 to $30,000. 1. Bitcoin’s price has fluctuated dramatically since 2009, reaching nearly $20,000 in 2017 and nearly $60,000 in 2021. 2. Prices are affected by factors such as market demand, supply, and macroeconomic environment. 3. Get real-time prices through exchanges, mobile apps and websites. 4. Bitcoin price is highly volatile, driven by market sentiment and external factors. 5. It has a certain relationship with traditional financial markets and is affected by global stock markets, the strength of the US dollar, etc. 6. The long-term trend is bullish, but risks need to be assessed with caution.
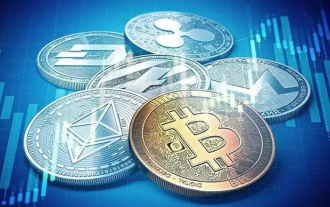
Currently ranked among the top ten virtual currency exchanges: 1. Binance, 2. OKX, 3. Gate.io, 4. Coin library, 5. Siren, 6. Huobi Global Station, 7. Bybit, 8. Kucoin, 9. Bitcoin, 10. bit stamp.
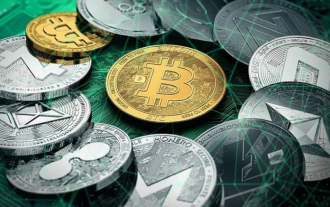
The top ten cryptocurrency trading platforms in the world include Binance, OKX, Gate.io, Coinbase, Kraken, Huobi Global, Bitfinex, Bittrex, KuCoin and Poloniex, all of which provide a variety of trading methods and powerful security measures.
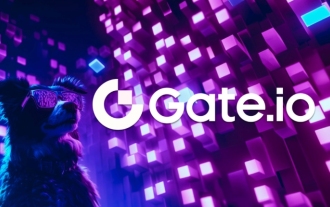
MeMebox 2.0 redefines crypto asset management through innovative architecture and performance breakthroughs. 1) It solves three major pain points: asset silos, income decay and paradox of security and convenience. 2) Through intelligent asset hubs, dynamic risk management and return enhancement engines, cross-chain transfer speed, average yield rate and security incident response speed are improved. 3) Provide users with asset visualization, policy automation and governance integration, realizing user value reconstruction. 4) Through ecological collaboration and compliance innovation, the overall effectiveness of the platform has been enhanced. 5) In the future, smart contract insurance pools, forecast market integration and AI-driven asset allocation will be launched to continue to lead the development of the industry.
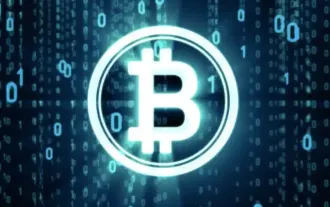
The top ten digital currency exchanges such as Binance, OKX, gate.io have improved their systems, efficient diversified transactions and strict security measures.
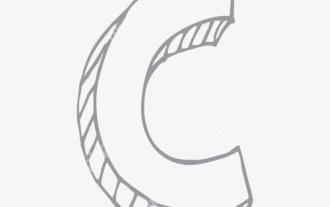
Using the chrono library in C can allow you to control time and time intervals more accurately. Let's explore the charm of this library. C's chrono library is part of the standard library, which provides a modern way to deal with time and time intervals. For programmers who have suffered from time.h and ctime, chrono is undoubtedly a boon. It not only improves the readability and maintainability of the code, but also provides higher accuracy and flexibility. Let's start with the basics. The chrono library mainly includes the following key components: std::chrono::system_clock: represents the system clock, used to obtain the current time. std::chron
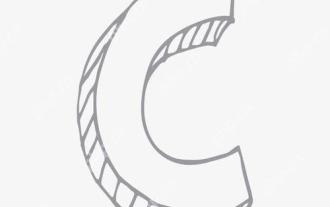
Handling high DPI display in C can be achieved through the following steps: 1) Understand DPI and scaling, use the operating system API to obtain DPI information and adjust the graphics output; 2) Handle cross-platform compatibility, use cross-platform graphics libraries such as SDL or Qt; 3) Perform performance optimization, improve performance through cache, hardware acceleration, and dynamic adjustment of the details level; 4) Solve common problems, such as blurred text and interface elements are too small, and solve by correctly applying DPI scaling.
