Image style migration example in Python
Image style transfer is a technology based on deep learning that can transfer the style of one image to another image. In recent years, image style transfer technology has been widely used in the fields of art and film and television special effects. In this article, we will introduce how to implement image style migration using Python language.
1. What is image style transfer
Image style transfer can transfer the style of one image to another image. The style can be the artist's painting style, the photographer's shooting style, or other styles. The goal of image style transfer is to preserve the content of the original image while giving it a new style.
Image style transfer technology is a deep learning technology based on convolutional neural network (CNN). Its core idea is to extract the content and style information of the image through a pre-trained CNN model, and use optimization methods to combine the two or composited into a new image. Typically, the content information of an image is extracted through the deep convolutional layers of CNN, while the style information of the image is extracted through the correlation between the convolution kernels of CNN.
2. Implement image style migration
The main steps to implement image style migration in Python include loading images, preprocessing images, building models, calculating loss functions, using optimization methods to iterate and Output results. Next, we'll cover these step-by-step.
- Loading images
First, we need to load an original image and a reference image. The original image is the image that needs to be style transferred, and the reference image is the style image that is to be transferred. Loading images can be done using Python's PIL (Python Imaging Library) module.
from PIL import Image import numpy as np # 载入原始图像和参考图像 content_image = Image.open('content.jpg') style_image = Image.open('style.jpg') # 将图像转化为numpy数组,方便后续处理 content_array = np.array(content_image) style_array = np.array(style_image)
- Preprocessing image
Preprocessing includes converting the original image and the reference image into a format that the neural network can process, that is, converting the image into a Tensor and performing standardization at the same time . Here, we use the preprocessing module provided by PyTorch to complete.
import torch import torch.nn as nn import torchvision.transforms as transforms # 定义预处理函数 preprocess = transforms.Compose([ transforms.ToTensor(), transforms.Normalize(mean=[0.485, 0.456, 0.406], std=[0.229, 0.224, 0.225]) ]) # 将图像进行预处理 content_tensor = preprocess(content_image).unsqueeze(0).to(device) style_tensor = preprocess(style_image).unsqueeze(0).to(device)
- Building a model
The image style transfer model can use models that have been trained on large-scale image databases. Commonly used models include VGG19 and ResNet. Here we use the VGG19 model to complete. First, we need to load the pre-trained VGG19 model and remove the last fully connected layer, leaving only the convolutional layer. Then, we need to adjust the content information and style information of the image by modifying the weights of the convolutional layer.
import torchvision.models as models class VGG(nn.Module): def __init__(self, requires_grad=False): super(VGG, self).__init__() vgg19 = models.vgg19(pretrained=True).features self.slice1 = nn.Sequential() self.slice2 = nn.Sequential() self.slice3 = nn.Sequential() self.slice4 = nn.Sequential() self.slice5 = nn.Sequential() for x in range(2): self.slice1.add_module(str(x), vgg19[x]) for x in range(2, 7): self.slice2.add_module(str(x), vgg19[x]) for x in range(7, 12): self.slice3.add_module(str(x), vgg19[x]) for x in range(12, 21): self.slice4.add_module(str(x), vgg19[x]) for x in range(21, 30): self.slice5.add_module(str(x), vgg19[x]) if not requires_grad: for param in self.parameters(): param.requires_grad = False def forward(self, x): h_relu1 = self.slice1(x) h_relu2 = self.slice2(h_relu1) h_relu3 = self.slice3(h_relu2) h_relu4 = self.slice4(h_relu3) h_relu5 = self.slice5(h_relu4) return h_relu1, h_relu2, h_relu3, h_relu4, h_relu5 device = torch.device("cuda" if torch.cuda.is_available() else "cpu") model = VGG().to(device).eval()
- Calculate the loss function
Since the goal of image style transfer is to retain the content of the original image while giving it a new style, we need to define a loss function to achieve this goal. The loss function consists of two parts, one is content loss and the other is style loss.
Content loss can be defined by calculating the mean square error between the original image and the generated image in the feature map of the convolutional layer. The style loss is defined by calculating the mean square error between the Gram matrix between the feature map of the generated image and the style image in the convolutional layer. The Gram matrix here is the correlation matrix between the convolution kernels of the feature map.
def content_loss(content_features, generated_features): return torch.mean((content_features - generated_features)**2) def gram_matrix(input): batch_size , h, w, f_map_num = input.size() features = input.view(batch_size * h, w * f_map_num) G = torch.mm(features, features.t()) return G.div(batch_size * h * w * f_map_num) def style_loss(style_features, generated_features): style_gram = gram_matrix(style_features) generated_gram = gram_matrix(generated_features) return torch.mean((style_gram - generated_gram)**2) content_weight = 1 style_weight = 1000 def compute_loss(content_features, style_features, generated_features): content_loss_fn = content_loss(content_features, generated_features[0]) style_loss_fn = style_loss(style_features, generated_features[1]) loss = content_weight * content_loss_fn + style_weight * style_loss_fn return loss, content_loss_fn, style_loss_fn
- Iteration using the optimization method
After calculating the loss function, we can use the optimization method to adjust the pixel values of the generated image to minimize the loss function . Commonly used optimization methods include gradient descent method and L-BFGS algorithm. Here, we use the LBFGS optimizer provided by PyTorch to complete image migration. The number of iterations can be adjusted as needed. Usually, 2000 iterations can get better results.
from torch.optim import LBFGS generated = content_tensor.detach().clone().requires_grad_(True).to(device) optimizer = LBFGS([generated]) for i in range(2000): def closure(): optimizer.zero_grad() generated_features = model(generated) loss, content_loss_fn, style_loss_fn = compute_loss(content_features, style_features, generated_features) loss.backward() return content_loss_fn + style_loss_fn optimizer.step(closure) if i % 100 == 0: print('Iteration:', i) print('Total loss:', closure().tolist())
- Output results
Finally, we can save the generated image locally and observe the effect of image style migration.
import matplotlib.pyplot as plt generated_array = generated.cpu().detach().numpy() generated_array = np.squeeze(generated_array, 0) generated_array = generated_array.transpose(1, 2, 0) generated_array = np.clip(generated_array, 0, 1) plt.imshow(generated_array) plt.axis('off') plt.show() Image.fromarray(np.uint8(generated_array * 255)).save('generated.jpg')
3. Summary
This article introduces how to use Python language to implement image style transfer technology. By loading the image, preprocessing the image, building the model, calculating the loss function, iterating with the optimization method and outputting the result, we can transfer the style of one image to another. In practical applications, we can adjust parameters such as reference images and number of iterations according to different needs to obtain better results.
The above is the detailed content of Image style migration example in Python. For more information, please follow other related articles on the PHP Chinese website!

Hot AI Tools

Undresser.AI Undress
AI-powered app for creating realistic nude photos

AI Clothes Remover
Online AI tool for removing clothes from photos.

Undress AI Tool
Undress images for free

Clothoff.io
AI clothes remover

Video Face Swap
Swap faces in any video effortlessly with our completely free AI face swap tool!

Hot Article

Hot Tools

Notepad++7.3.1
Easy-to-use and free code editor

SublimeText3 Chinese version
Chinese version, very easy to use

Zend Studio 13.0.1
Powerful PHP integrated development environment

Dreamweaver CS6
Visual web development tools

SublimeText3 Mac version
God-level code editing software (SublimeText3)

Hot Topics










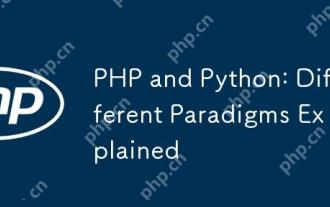
PHP is mainly procedural programming, but also supports object-oriented programming (OOP); Python supports a variety of paradigms, including OOP, functional and procedural programming. PHP is suitable for web development, and Python is suitable for a variety of applications such as data analysis and machine learning.
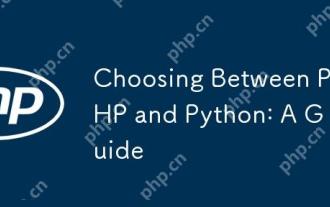
PHP is suitable for web development and rapid prototyping, and Python is suitable for data science and machine learning. 1.PHP is used for dynamic web development, with simple syntax and suitable for rapid development. 2. Python has concise syntax, is suitable for multiple fields, and has a strong library ecosystem.
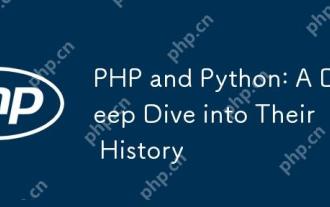
PHP originated in 1994 and was developed by RasmusLerdorf. It was originally used to track website visitors and gradually evolved into a server-side scripting language and was widely used in web development. Python was developed by Guidovan Rossum in the late 1980s and was first released in 1991. It emphasizes code readability and simplicity, and is suitable for scientific computing, data analysis and other fields.
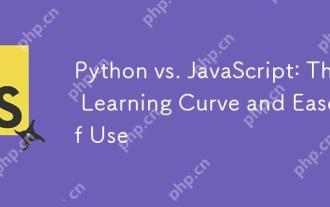
Python is more suitable for beginners, with a smooth learning curve and concise syntax; JavaScript is suitable for front-end development, with a steep learning curve and flexible syntax. 1. Python syntax is intuitive and suitable for data science and back-end development. 2. JavaScript is flexible and widely used in front-end and server-side programming.
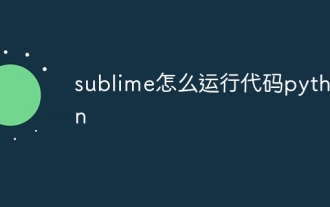
To run Python code in Sublime Text, you need to install the Python plug-in first, then create a .py file and write the code, and finally press Ctrl B to run the code, and the output will be displayed in the console.
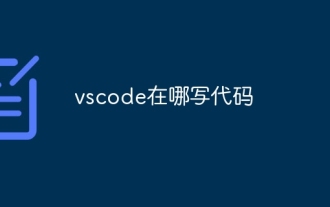
Writing code in Visual Studio Code (VSCode) is simple and easy to use. Just install VSCode, create a project, select a language, create a file, write code, save and run it. The advantages of VSCode include cross-platform, free and open source, powerful features, rich extensions, and lightweight and fast.
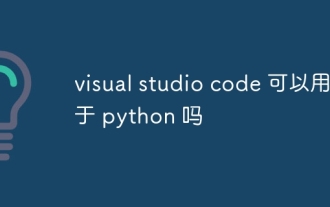
VS Code can be used to write Python and provides many features that make it an ideal tool for developing Python applications. It allows users to: install Python extensions to get functions such as code completion, syntax highlighting, and debugging. Use the debugger to track code step by step, find and fix errors. Integrate Git for version control. Use code formatting tools to maintain code consistency. Use the Linting tool to spot potential problems ahead of time.
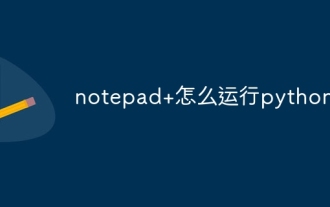
Running Python code in Notepad requires the Python executable and NppExec plug-in to be installed. After installing Python and adding PATH to it, configure the command "python" and the parameter "{CURRENT_DIRECTORY}{FILE_NAME}" in the NppExec plug-in to run Python code in Notepad through the shortcut key "F6".
