How to use open source datasets in Python?
With the advent of the big data era, data analysis and machine learning have become popular fields. However, how to obtain the data set, analyze it and train the model can be a difficult task for beginners. To solve this problem, the open source community has provided rich data sets, and Python, as a popular programming language, also provides various methods to use these data sets.
This article introduces methods and tools for using open source data sets in Python, such as data loading, browsing, cleaning, visualization and analysis. We will use publicly available data sets for practical demonstrations to help readers master these skills.
- Loading the data set
First, we need to load the data set into the Python program. There are many open source datasets that can be downloaded from the web, such as UCI Machine Learning Repository, Kaggle, etc. These data sets are generally saved in various formats such as CSV, JSON, and XML.
In Python, pandas is a very useful library. We can use pandas to load a CSV format data set with a few lines of code:
import pandas as pd data = pd.read_csv("example.csv")
- Data browsing
Once the data set is loaded into Python, we can start exploring the data. We can use the head() method of pandas to view the first few rows of data:
print(data.head())
If we want to view the last few rows in the data set, we can use the tail() method.
We can also use the shape attribute to get the size of the data set:
print(data.shape)
In addition, we can use the describe() method to get simple statistics of the data set, such as the minimum value and maximum value , average, etc.:
print(data.describe())
- Data Cleaning
When we browse the data set, we may find that there are missing values, outliers, or duplicate values in the data set. question. In data analysis and machine learning, these problems are very serious, so we need to clean them.
For missing values, we can use the fillna() method to fill them with 0 or the average value:
data.fillna(0, inplace=True)
If we want to delete duplicate rows in the data set, we can use drop_duplicates( ) Method:
data.drop_duplicates(inplace=True)
For outliers, we can use the standard deviation to determine whether it is abnormal and replace it with the mean:
mean = data["col"].mean() std = data["col"].std() cut_off = std * 3 lower, upper = mean - cut_off, mean + cut_off new_data = [x if x > lower and x < upper else mean for x in data["col"]] data["col"] = new_data
- Data Visualization
Data visualization is one of the important steps in data analysis. In Python, we can use libraries such as Matplotlib and Seaborn for data visualization.
For example, we can use the Matplotlib library to draw a line chart in the data set:
import matplotlib.pyplot as plt plt.plot(data["col"]) plt.show()
or use the Pairplot method of the Seaborn library to make a distribution chart of multiple variables:
import seaborn as sns sns.pairplot(data)
- Data Analysis
After data visualization, we can conduct more in-depth data analysis, such as building models, training models, predictions, etc. Python provides many libraries to support these operations, such as Scikit-learn and TensorFlow, among others.
For example, we can use the Scikit-learn library to build a linear regression model:
from sklearn.linear_model import LinearRegression from sklearn.model_selection import train_test_split X = data[["col1", "col2"]] y = data["target_col"] X_train, X_test, y_train, y_test = train_test_split(X, y, test_size=0.2) model = LinearRegression() model.fit(X_train, y_train) y_pred = model.predict(X_test)
In the above example, we use the train_test_split method to divide the data set into a training set and a test set, and then Use the LinearRegression class to build a model, and finally use the predict method to predict the test set.
Conclusion
This article introduces how to use open source datasets for data analysis and machine learning in Python. We use the pandas library to load and browse datasets, the Matplotlib and Seaborn libraries for data visualization, and the Scikit-learn library to build and train models. These techniques and tools are not only applicable to the open source data sets mentioned in this article, but also to other types of data sets, such as Web data, sensor data, etc. As data analysis and machine learning develop, these technologies and tools will continue to be updated and improved, providing better performance and ease of use.
The above is the detailed content of How to use open source datasets in Python?. For more information, please follow other related articles on the PHP Chinese website!

Hot AI Tools

Undresser.AI Undress
AI-powered app for creating realistic nude photos

AI Clothes Remover
Online AI tool for removing clothes from photos.

Undress AI Tool
Undress images for free

Clothoff.io
AI clothes remover

Video Face Swap
Swap faces in any video effortlessly with our completely free AI face swap tool!

Hot Article

Hot Tools

Notepad++7.3.1
Easy-to-use and free code editor

SublimeText3 Chinese version
Chinese version, very easy to use

Zend Studio 13.0.1
Powerful PHP integrated development environment

Dreamweaver CS6
Visual web development tools

SublimeText3 Mac version
God-level code editing software (SublimeText3)

Hot Topics










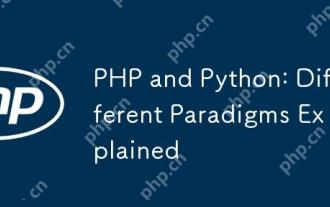
PHP is mainly procedural programming, but also supports object-oriented programming (OOP); Python supports a variety of paradigms, including OOP, functional and procedural programming. PHP is suitable for web development, and Python is suitable for a variety of applications such as data analysis and machine learning.
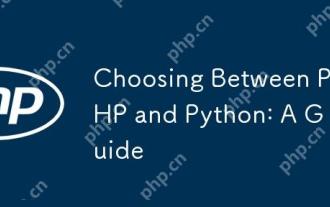
PHP is suitable for web development and rapid prototyping, and Python is suitable for data science and machine learning. 1.PHP is used for dynamic web development, with simple syntax and suitable for rapid development. 2. Python has concise syntax, is suitable for multiple fields, and has a strong library ecosystem.
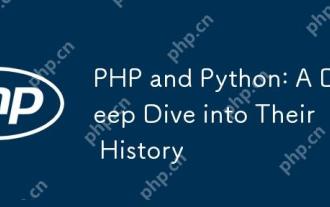
PHP originated in 1994 and was developed by RasmusLerdorf. It was originally used to track website visitors and gradually evolved into a server-side scripting language and was widely used in web development. Python was developed by Guidovan Rossum in the late 1980s and was first released in 1991. It emphasizes code readability and simplicity, and is suitable for scientific computing, data analysis and other fields.
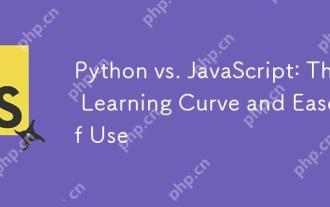
Python is more suitable for beginners, with a smooth learning curve and concise syntax; JavaScript is suitable for front-end development, with a steep learning curve and flexible syntax. 1. Python syntax is intuitive and suitable for data science and back-end development. 2. JavaScript is flexible and widely used in front-end and server-side programming.
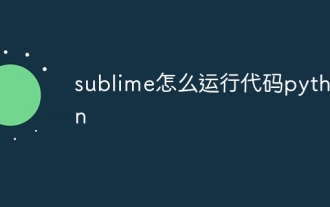
To run Python code in Sublime Text, you need to install the Python plug-in first, then create a .py file and write the code, and finally press Ctrl B to run the code, and the output will be displayed in the console.
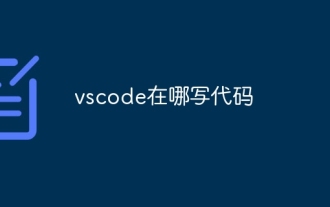
Writing code in Visual Studio Code (VSCode) is simple and easy to use. Just install VSCode, create a project, select a language, create a file, write code, save and run it. The advantages of VSCode include cross-platform, free and open source, powerful features, rich extensions, and lightweight and fast.
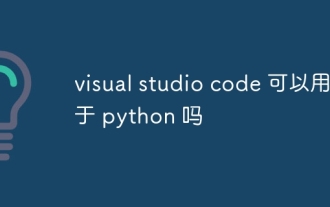
VS Code can be used to write Python and provides many features that make it an ideal tool for developing Python applications. It allows users to: install Python extensions to get functions such as code completion, syntax highlighting, and debugging. Use the debugger to track code step by step, find and fix errors. Integrate Git for version control. Use code formatting tools to maintain code consistency. Use the Linting tool to spot potential problems ahead of time.
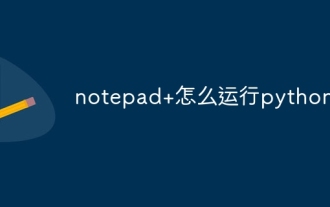
Running Python code in Notepad requires the Python executable and NppExec plug-in to be installed. After installing Python and adding PATH to it, configure the command "python" and the parameter "{CURRENT_DIRECTORY}{FILE_NAME}" in the NppExec plug-in to run Python code in Notepad through the shortcut key "F6".
